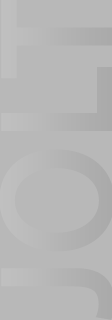 |
MERLOT Journal of Online
Learning and Teaching |
Vol.
4, No. 3, September 2008
|
|
Learning Support Systems for Management Education:
Screening for Success
Owen P. Hall, Jr.
Graziadio School of Business and Management
Pepperdine University
Malibu, CA USA
ohall@pepperdine.edu
|
Abstract
Working adults are entering MBA programs in
increasing numbers. Some matriculating
professionals have difficulty meeting the
competitive rigors of business school life for a
variety of reasons. One solution to these challenges
is to provide learning support systems geared toward
individual student backgrounds and interests. The
purpose of this paper is to illustrate a screening
process for delivering content in a non-residential
MBA program for
two diverse academic disciplines: organizational
management and accounting. The results show that
analytical-based classification techniques can
effectively identify marginal students for the
purpose of providing additional learning resources.
The analysis further shows that intelligent tutors
can deliver customized Web-based content at a time
convenient to the working professional.
Keywords:
Working adults, learning support systems, management
education, student screening, Internet, distance
learning, intelligent agents, non-residential MBA
|
Introduction
Many working professionals enrolling into MBA
programs are often challenged with the competitive
rigors of graduate school. This situation can be
attributed to a number of factors including 1) time
elapsed since completing undergraduate
degree, 2) lack of
an undergraduate business degree, and 3) lack of
familiarity with modern learning resources such as
digital libraries. In response many business schools
provide a variety of support systems to help
transition the student back to academic life.
Schools of business also recognize that the
complexities and interrelated nature of modern
business call for an integrated as well as
results-oriented learning framework for management
education (Camuffo, 2004). This
approach is particularly appropriate for working
professionals as many already possess a rich
work-related experiential capability that can
contribute to the collective learning environment in
an andragogical context (Hynes, 2002). In an
integrated learning environment the focus is on
understanding how basic management functions such as
operations, finance, and marketing are linked.
Furthermore, it has been long recognized that
active, participatory and systematic learning is
more effective than passive learning and that
learners need feedback early and often (Atwater,
2006).
One learning stratagem that supports this
perspective is the Instructional Management System (IMS)
cooperative initiative (Graves, 1999). This
initiative is designed to promote systematic
thinking regarding the delivery of higher education,
to improve learning outcomes and to increase return
on instruction investments. Web-based learning
support systems are the embodiment of the IMS
initiative. These systems are receiving increased
attention because they provide the capability to
deliver customized content at a time convenient to
the student (Kathawala, 2002).
In the screening process outlined in this paper
entering MBA students are identified as requiring
additional preparation. Internet-based artificial
agents are then used to provide these students with
the appropriate learning support resources. The
objective of this support process is to assist
struggling students to succeed. The same process
also can be used for students that require a
refresher during their course of studies. This paper consists of
three parts: 1) a review of the relevant literature;
2) a statistical analysis of an MBA program
involving fully employed students, and 3) an
application of the screening paradigm to the diverse
disciplines of management organization and
accounting.
Background and Literature
Today, the general pedagogical direction in
management education is moving increasingly towards
a learning-centric perspective (Driver, 2002).
Accordingly, the roadmap to effective learning for
working professionals is a flexible and customized
curriculum. This perspective is predicated on the
fact that many
students do not enter MBA programs with the same
technical or work experience background. Therefore,
providing self-paced customized content as part of
the overall curriculum design can further enhance
the learning experience. Generally speaking,
students tend to participate more in learning
systems that are content-rich and that feature
extensive variety (Neo, 2004).
The literature is replete with studies on estimating
MBA student performance (Hancock, 1999; Clayton,
2004). The primary focus of these studies has been
on admissions screening. The Graduate Management
Aptitude Test (GMAT) and undergraduate GPA (grade
point average) have been found to be correlated with
successful performance in residential MBA programs (Hedlund,
2006; Sireci, 2006). These factors, plus work
experience, were found to be significant for working
professionals enrolled in evening or weekend
non-residential MBA programs (Carver, 1994). Many of
the analytical admission studies have used classical
regression techniques where the R-squares tend to be
small often in the 0.20 to 0.40 range (Yang, 2001).
Classification analysis which provides for a more
direct identification of student performance
potential has been shown to be somewhat more
efficient than classical regression forecasting
techniques (Wilson, 1995).
Customized asynchronous learning systems provide a
practical environment for competency development.
Once the screening system has classified the student
as potentially marginal, the specific
resource learning package can be identified and
delivered via the Internet. Using customized
content and tutorials, which also can be delivered
via the Internet, students can control the learning
pace which in turn should help ameliorate the
frustration of falling behind. This is particularly
important for preparatory type courses such as
organizational behavior and accounting that
typically provide the foundation for MBA core
courses. Figure 1 illustrates how the screening
paradigm could be used for providing learning
support resources. This system would be part of the
overall blended (hybrid) learning environment, which
combines the best practices in
both traditional
and Web-based instruction (Hwang, 2006). In a
similar way classification screening models can also
be used for identifying learning support resources
for matriculating
students.
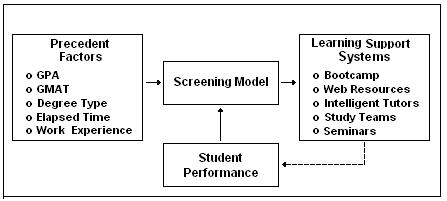
Figure 1.
Learning Support System Design Concept
|
This learning support system consists of the
following three basic components:
·
Predictor variables including GMAT score, GPA, type
of undergraduate degree, elapsed time since
graduation and work related experience.
·
Screening model (e.g., neural nets) for identifying
students requiring additional academic support.
·
Learning support systems comprising discipline
specific seminars, bootcamps, and Web-based
resources supported by intelligent tutors.
Web-based learning support systems have been found
to be increasingly effective in providing management
education to working adults (Lundgren, 2003; Shih,
2003; H. Huang, 2002). The 24/7 capability of
the Web supports the challenges associated with
non-traditional MBA programs. For example, a working
student on travel status can remain current with the
course material and the lesson plan. Furthermore,
students can engage in remedial learning at a
convenient time. Some characteristics of Web-based
learning support systems are highlighted below:
·
Provide a high degree of interaction and
collaboration that supports working professionals.
·
Present a long sought solution to the ongoing
problems associated with working adult students.
Students can now have a dynamic and personal
experience for continuous learning.
·
Offer learners a
purposeful entry to the Internet and online
resources, and to a new era of learning
technologies.
·
Underpin new patterns of relationships between
education and business that directly impact the
learning process.
The delivery of customized remedial content via the
Internet can be facilitated though the use of
intelligent tutors. These artificial intelligence
based systems are defined as purposeful autonomous
entities capable of adapting to changing demands
such as found in many unstructured and
semi-structured educational applications.
Intelligent agents allow the active reconfiguration
of the learning presentation according to current
requirements and the availability of information
sources (Li, 2007).
Typically, intelligent tutors should possess the
following four basic characteristics: autonomous,
proactive, flexible, and user-friendly. The social
interface between the agent and the student should
be highly visual with limited user required inputs.
It is within this design context that the learning
objectives can be best achieved. For example, if a
student is having difficulty as detected by testing,
simulation or self-assessment, then the synthetic
agent would identify and provide specific additional
learning content to the student via the Internet.
The content delivery modes could include streaming
videos, virtual seminars and simulations. To be
effective, the agent must include such
functionalities as personalized content management,
learner model, learner plan, and adaptive
interaction (Xu, 2006). The use of intelligent
tutors is on the rise throughout academe with
particular emphasis on adult education (Ghaoui,
2003; Cheung, 2003).
A database was developed based on the above
literature review and an assessment of the available
student records. The primary target variable was
student GPA at time of graduation from the MBA
program. An overview of the developed database along
with some preliminary statistical findings is
presented in the following section.
Database
The database consisted of files on students that
have graduated from
an Association to Advance Collegiate Schools of
Business (AACSB) accredited working professional MBA
program for the period 2000 to 2006. The sample
size was 1880. Table 1 provides descriptive
statistics for the variables used in this study. The
data show, for example, that slightly over one-third
of the students had an undergraduate business or
economics degree. The incoming GPA data was adjusted
for grade inflation. Undergraduate grade inflation
has been averaging approximately 0.015 per year over
the past 30 years (Kezim, 2005). This adjustment was
called for due to the fact that 15 percent of the
students had been away from the classroom for more
than 10 years. The average elapsed time since
receiving their undergraduate degree was nearly six
years. The difference between the average
unadjusted and adjusted incoming GPA is 0.08. For
the current study elapse time since completing the
undergraduate degree is used as a proxy for work
experience. The outgoing GPA was not adjusted since
the average GPA over the study’s timeframe had not
changed.
The time to complete the MBA program, while not a
precedent variable, was included in the database.
The primary interest for including this variable was
twofold: 1) to determine if any of the precedent
variables are useful in estimating the time to
compete the program, and 2) to determine the extent
of the relationship between time to complete and
outgoing GPA. The existence of such relationships
could be used for class scheduling, in the first
case, and for identifying students that could use
additional learning resources, in the second case.
Table 1.
Cohort Group Descriptive Statistics
Variable |
Mnemonic |
Mean |
S. Dev. |
Entering Age (yrs.) |
Age |
29.9 |
5.2 |
Gender (% female, F=1)) |
Gender |
0.37 |
- |
Undergraduate Business/Econ Degree (%, B/E=1) |
BAE |
0.38 |
- |
Incoming GPA * |
GPAI |
3.05 |
0.41 |
Time Elapse since Undergraduate Degree (yrs.) |
Period |
5.77 |
4.5 |
GMAT Score |
GMAT |
528 |
78.8 |
Time to Complete MBA (mos.) |
Time |
34.3 |
12.7 |
Outgoing GPA |
GPAO |
3.53 |
0.23 |
* Adjusted for grade inflation.
Table 2 presents zero-order correlation coefficients
for the database. The correlation data revealed the
following statistically significant relationships
with GPAO:
·
Age (r = 0.09, p = 0.00) ●
Period (r = 0.21, p = 0.00)
·
GPAI (r = 0.36, p = 0.00) ● GMAT
(r = 0.47, p = 0.00)
Table 2. Correlation Matrix (Pearson)
Variables |
1 |
2 |
3 |
4 |
5 |
6 |
1. Age |
1 |
|
|
|
|
|
2. GPAI |
0.06* |
1 |
|
|
|
|
3. Period |
0.71* |
0.08* |
1 |
|
|
|
4. GMAT |
0.01 |
0.18* |
0.12* |
1 |
|
|
5. Time |
0.06* |
- 0.01 |
0.03 |
- 0.11* |
1 |
|
6. GPAO |
0.09* |
0.36* |
0.21* |
0.47* |
- 0.11* |
1 |
*Significant at 0.05
These correlations are consistent with those found
for non-traditional MBA programs (Carver, 1994).
Specifically, GMAT score had the largest positive
correlation with GPAO (r = 0.47, p=0.00). The
correlation for the non-adjusted incoming GPA was
0.33 or 0.03 points lower than the adjusted GPA. Not
surprisingly, student age and the elapsed time since
completing undergraduate training are highly
correlated (r=0.77, p=0.00). The time to complete
the program was found to be negatively correlated
with GPAO (r = -0.11, p = 0.0). This suggests that
students taking less than a full course load may
also be candidates for additional learning support
resources. Typically, a full course load for
non-traditional MBA students is eight to ten units
per term. The results of a more detailed analysis of
the database are highlighted in the following
section.
|
Results and Analysis
The database was more fully explored using a single
hidden layer, feed-forward neural net model and a
logistic regression model. The neural net model
consisted of one input, one hidden and one output
layer. Neural nets use complex network relationships
to mimic the connections between sets of data. Among
other things, neural nets have the advantage of not
requiring prior assumptions about possible
relations, as is the case with traditional analysis
methods (Adya, 1998).
A dichotomous categorical variable based on student
GPA at the time of graduation (GPAO) was used in the
classification analysis. The two categories were
marginal, defined as a student with a GPA less than
3.3, and successful, defined as a student with a GPA
of 3.3 or greater (Hardgrave, 1994). The division
yielded approximately 20 percent of the alumni
records classified as marginal and the remaining 80
percent classified as successful. The large ratio
between successful and marginal (i.e., 4:1) tends to complicate the analytical screening
process. The results of the screening analysis are
highlighted in Table 3. The overall accuracy rate of
the neural net is 88 percent compared to 82 percent
for the logistic regression. This statistic is
defined as the proportion of correct classifications
across all categories. |
Table 3. Comparison of Classification Analysis of
Target Variable (%) |
|
Neural Net |
Logistic |
|
Classified Marginal |
Classified Successful |
Classified Marginal |
Classified Successful |
Actual Marginal |
82 |
18 |
16 |
84 |
Actual Successful |
4 |
96 |
3 |
97 |
Neural nets have previously been used for predicting
MBA student success (Naik, 2004). This model
included ten explanatory variables and a database
consisting of 184 observations. The results
indicated that the neural net model outperformed
both the logit and probit models based on the same
classification scheme used in the present study. The
results reported in Table 3 are consistent with the
Naik findings. The performance differences between
the two modeling approaches in Table 3 are quite
apparent when comparing the marginal
classifications. Here the neural net model shows
improved classification performance over the
logistic model (82 percent
versus 16
percent).
Table 4 shows the relative importance of the
predictor variables for the two modeling approaches
where GPAO is the classification variable (marginal
and successful). The predictor variables are ranked
based on the neural net (NN) model importance
factors (IP). As can be seen the neural net
classifier ranked GPAI as the most important factor
followed by GMAT. The logistic model uses the odds
ratio as an indication of the relative importance of
the predictor variables. For example, GMAT has an
odds ratio near one. This suggests that a 10-point
increase in GMAT score (the minimum increment)
indicates that the odds of being successful in the
MBA program increase by a factor of ten percent.
Table 4.
Analysis
of Variable Relative Importance
Variable |
NN(IP) |
Logistic (Odds Ratio)* |
GPAI |
0.329 |
6.44 |
GMAT |
0.219 |
1.01 |
Period |
0.206 |
1.14 |
Age |
0.200 |
0.95 |
Bus/Econ |
0.045 |
1.30 |
*Statistically
significant using Wald test @ 0.05
Applications
The learning support process outlined above will now
be illustrated for two diverse MBA disciplines:
organizational management and accounting. A similar
approach can be followed for core courses such as
finance, economics, marketing, and information
systems. In many MBA programs, especially those
geared towards working adults, some students have
limited interpersonal, cognitive processing, and
writing capabilities. Furthermore, many
matriculating students will not have an
undergraduate degree in business. Once a student has
been identified via the screening process as
marginal, the next step is to identify the
appropriate learning support resources. Testing and
simulations, for example, can be used to specify the
content and delivery mode for each of these
objectives. The evidence suggests that
students engaged in simulations retain about 75
percent of the instructional content compared to
five percent for lectures (Johne, 2003).
Intelligent tutors can also play a
constructive role
in delivering content. Two key issues associated
with the use of intelligent agents include (Harrer,
2006):
-
What types of learning applications are most
amenable to cognitive tutoring?
-
At what pace should feedback be provided
(instantaneous or lagged)?
Organizational Management Discipline
Effective communication is a fundamental
prerequisite to modern management practice.
Web-based simulations can be used to help develop
basic writing skills for struggling students. For
example, writing-based simulation models provide the
student with the context to develop analytical
thinking in a realistic business context (Rehling,
2005). In a simulation environment, students are
able to work with writing and problem-solving
situations that are linked to the larger business
decision-making process. This construct allows
students to develop higher order cognitive skills.
A major learning objective in graduate management
education is to enhance decision-making skills, that
is, the development of cognitive competencies such
as problem solving, critical thinking, formulating
questions, searching for relevant information,
making informed judgments, conducting observations,
inventing and creating new ideas, and analyzing and
presenting data. Business decisions are invariably
outcomes of multi-disciplinary discussions involving
extensive interactions. A virtual learning
environment
provides an ideal platform for enhancing students
experience in capturing inputs from a variety of
sources (Martins, 2004). This process tends to
mirror the professional work environment and thus
serves as a reinforcing vehicle. In particular,
these networks provide an efficient platform for
enhancing students’ experiences in understanding how
to capture and process information from a variety of
sources at a time and place of the students
choosing.
Organizational Management Support Systems
The nature of organizational management suggests
that no one learning approach is optimal. Instead
course content can be
delivered via a variety of learning venues (DeSanctis,
2003). A number of these venues are highlighted in
Figure 2. This
paradigm underscores ways in which traditional and
Web-based learning methods can be used in
combination to optimize content delivery and
communication. Quadrant I (Asynchronous – Personal)
represents personalized learning such as writing or
planning. The focus of Quadrant II is on individual
online learning such as taking a virtual facility
tour or blogging. Quadrant III involves team
interactive learning on the Internet such as
simulations and linear chatrooms. In Quadrant IV
the emphasis is on real time multi-student learning
experiences such as team presentations and faculty
lectures. Typically, asynchronous learning is geared
more towards individual knowledge acquisition while
synchronous learning tends to involve multiple
student learning environments.
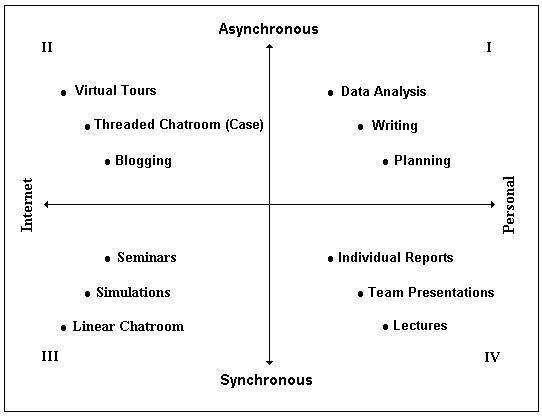 Figure
2.
Learning Venues
The hybrid learning environment
outlined in Figure 2 has many of the attributes of the
collaborative learning process associated with
virtual professional team structures. A core
requirement in these organizational arrangements is
the need for knowledge sharing. Virtual team
learning expands the potential for cognitive,
affective and action-learning outcomes (Clark,
2006). For example, many professional education
courses rely heavily on case method teaching which
requires class preparation through study group
meetings. For students, the class environment is
highly analogous to that of the emerging
multi-disciplinary teams in today’s organizations.
Further analogies can be found in the nature of the
case analysis task that requires preparation of a
recommended intervention within a limited time
frame. Students with expertise in the subject
industry or discipline usually add a rich dimension
to these analyses. Guest
speakers, real or virtual, can also add a dynamic
perspective to student discussions.
Collaborative Learning
There are a variety of virtual collaborative formats
including chatrooms, bulletin boards and blogs.
There are two basic chatroom options: linear
(synchronous) and threaded (asynchronous). In a
linear chatroom environment students are encouraged
to interact proactively in near real time to a
specific case or problem. The primary focus is on
the interactive process. Chatrooms and electronic
bulletin boards have been successfully utilized in a
variety of graduate level courses including
organizational behavior (Brower, 2003). Some critics
of distance learning suggest that virtual learners
remain isolated from each other, which reduces
personal student and instructor interactions,
thereby, diminishing opportunities for effective
learning. However, recent evidence indicates that
students engaged in virtual learning communities
score higher on measures of interaction than do
students in a traditional classroom setting (Hay,
2004).
Fully employed, non-residential students are often
called away on work related assignments, including
global travel which place additional burdens on
students. Consequently, developing a sense of
community is an essential ingredient for optimizing
the learning experience in a fully employed,
non-residential graduate management program. Shared
emotional connection includes
feelings of
relationships, shared history, and community
spirit. As students
become more connected with other students through
these virtual communities, they reap the benefits of
both professional and social relationships (Oravec,
2003). Student
participation in virtual communities can increase
involvement within the traditional classroom
environment (Bakardjieva, 2002). Furthermore, teams
that communicate regularly and interact socially
outside of class form more social capital which
often translates into improved face-to-face
communication (Aquino, 2005). Faculty can
facilitate this dynamic learning process by
constantly challenging assumptions and guiding the
dialogue.
One approach for maintaining a sense of community in
a virtual environment is through the process of
blogging (Flatley, 2005).
This is particularly the case in many fully employed
business management programs (Clyde,
2005). Blogging has
already found widespread acceptance in professional
societies for maintaining a personal log in
preparation for an accreditation examination (Naish,
2005). Professional applications where blogging is
used include organizational development, human
resources and law. Typically blogs are
updated frequently, often daily, and are presented
in reverse chronological order. Blogs can be
individual or team-based (i.e., group blogs). The
current state of the technology makes creating and
maintaining weblogs extremely straightforward. Blogs
can be used to share course or curriculum related
information or to provide more personal views and
feeling (Bar-llan, 2005). The posting of one’s
views do not necessarily have to be class-related.
It is simply part of the overall process of
developing a sense of community among classmates and
teammates.
The virtual community systems as outlined in this
paper can also help facilitate the use of students
as course co-producers. In this context students
and student teams can participate in the design and
delivery of specific course and curriculum content.
Working managers already possess extensive business
expertise and in some areas may have detailed
knowledge and expertise. This andragogical
perspective further enhances the value of the
virtual learning community.
Accounting Discipline
The Accounting Education Change Commission (AECC),
among other bodies, has been active in calling for
the incorporation of both innovative teaching as
well as active learning paradigms into accounting
courses (Saunders, 2003). In many MBA programs,
especially those geared towards working adults,
often, less than one-half of the students have taken
an accounting course. Furthermore, many of the
students with an undergraduate business degree have
not taken an accounting course in many years or do
not have job responsibilities that involve
accounting or financial activities. Some typical
learning objectives for an MBA-level accounting
principles course include:
·
Understanding the different emphases in managerial
and financial accounting
·
Preparing and analyzing income statements, balance
sheets, and cash flows
·
Evaluating the annual report of a public company
·
Assessing emerging issues, such as revenue
recognition and restructuring costs
·
Appreciating the ethical, global and regulatory
issues of accounting.
·
Comprehending and using accounting information
technology.
Again testing and simulations can be used to specify
the supplemental content and delivery mode for each
of these objectives. In this regard, early-on
screening that directs marginal students to an
accounting principles boot camp has been found to
improve significantly overall grade performance in
subsequent accounting related courses (J. Huang,
2005).
Delivering Customized Accounting Knowhow
The overall pedagogical approach for providing
customized accounting content is illustrated in
Table 5. For sake of clarity, this example, features
the first content layer for each of the five
candidate topical areas (e.g., accounting overview)
and a single delivery mode (e.g., streaming video).
In a fully deployed system there would be many more
topics, three-to-four support levels and a
comparable number of delivery modes.
|
Table 5.
Examples of Accounting Content Support
Topic |
Content Focus (1st level) |
Example Delivery Mode |
Accounting Overview |
Present main accounting concepts and how they
form the basis for GAAP |
Streaming Video |
Financial Statements |
Illustrate how to prepare a trial balance and
to develop income/balance sheets |
Computing Template |
|
Introduce formal record keeping and the steps
involved in the process. |
PowerPoint Presentation |
Ethics |
Demonstrate the importance of ethics in the
accounting profession. |
Simulation |
Information Technology |
Show how technology is used to enhance the
accounting process. |
Virtual Tour |
Web-based simulation can be used to illustrate the
basic principles of many accounting topics at a time
and place convenient to the student. For example,
the use of simulation in teaching ethics and
professional responsibilities in accounting has been
found to be an effective alternative to the
traditional classroom lecture (Haywood, 2003).
Accounting simulation models also provide the
student with the context to develop analytical
thinking in a realistic problem-solving environment
(Marriott, 2004). In a simulation environment
students are able to work with accounting
spreadsheets that are linked to the larger business
decision-making process. This construct allows
students to develop higher order cognitive skills.
The content delivery mode components outlined in
Table 5 are not mutually exclusive and can be used
in combination. If after participating in a
simulation the student is still having difficulties
additional resources like an ethics video or a case
as part of a study team exercise can be provided
(Dowling, 2003).
Another benefit of the screening process is that
students with the appropriate capabilities could opt
out of preparatory and core accounting classes,
thereby allowing for more specialization. This
approach was applied to a core MBA accounting course
(Chewing, 1999). The results, based on a logit
model, showed that prior accounting work,
quantitative GMAT scores and grade point averages in
undergraduate accounting courses were most
significant in classifying students. The call for
accounting assessment plans by both AACSB and the
accounting education community has resulted in the
development of a multi-dimensional framework
consisting of broad-based involvement, multi-trait
analysis, and follow-up assessment (Stout, 2005).
This plan includes multiple assessment measures and
provides feedback regarding both academic
(curriculum-related) and administrative
(operational) dimensions of performance. One way to
manage the process of content identification and
delivery is through the use of intelligent tutors.
Intelligent Tutors for Accounting
The use of intelligent tutors in accounting courses
is on the rise. For example, an expert system (AUDPORT)
has been used to assist accounting students to
better understand the audit reporting process
(McDuffie, 2006). This
knowledge-based system uses the criteria contained
in the Statement on
Auditing Standards No. 58
to determine the type of audit opinion (unqualified,
qualified, adverse disclaimer) that should be
rendered by the student after conducting a financial
audit under generally accepted auditing standards (GAAS).
Presented in the following are some selected AUDPORT
questions.
·
Did the client consistently apply generally accepted
accounting principles from year-to-year in the
financial statements that were audited?
·
Did the auditor discover any matters pertaining to
the financial statements, such as a subsequent event
or related party transaction, that are significant
enough to be referenced in the audit opinion?
·
Did the auditor discover a lack of adequate
disclosure in the financial statements or the
footnotes?
This learning system was tested in a first-level,
hybrid-based auditing class. The results, as
measured by grade performance and student
preferences, compared favorably with a traditional
course covering the same material. Intelligent
tutors have also been applied to courses in
accounting internal controls (Changchit, 2003). The
American Institute of Certified Public Accountants (AICPA)
defines internal controls as:
“A process - affected by an entity's board of
directors, management, and other personnel -
designed to provide reasonable assurance regarding
the achievement of objectives in the following
categories: reliability of financial reporting,
effectiveness and efficiency of operations, and
compliance with applicable laws and regulations.”
The expert learning system used in this
investigation was based on extensive interviews with
an internal control specialist. The specialist
characterized 126 internal control weaknesses and
the processes used to identify each weakness. The
results of this experiment showed that students
using the intelligent tutor package outperformed the
control group in detecting control violations. More
specifically, this study demonstrated that business
students using an expert system tutor detected
significantly more internal control weaknesses than
students who were exposed to traditional instruction
on detecting control violations.
An Application of Intelligent Tutors for Continuing
MBA Students
The expert system approach outlined above was used
in providing specialized accounting content for
continuing students enrolled in a fully employed MBA
program. The particular application involved
cost-based accounting (ABC).
Figure 3 shows a sample case and consultation system
used in this learning application. The student is
guided through a series of prompts regarding the
case. Explanations or clues are provided for each
prompt. The consultation can be taken more than once
since, among other things, some of the prompts are
randomized.
Once the student has completed the consultation the
expert system provides an overall learning
assessment and a PowerPoint review presentation. The
consultation can be taken more that once since,
among other things, some of the prompts are
randomized.
Conclusions
Working professionals are returning to the classroom
in record numbers. Many working adults entering a
non-residential MBA program have been away from the
classroom for some time, or do not have an
undergraduate degree in business, or both. These
conditions suggest the need for specialized learning
systems for assisting the student at the beginning
and over the course of the program. The purpose of
this paper is to illustrate a screening process for
delivering content in a non-residential MBA for two
diverse academic disciplines: organizational
management and accounting. An analysis of 1,880
alumni records for the period 2000 to 2006 shows
that GMAT scores, incoming GPA, and elapsed time
since completing
undergraduate degrees are useful in helping
identify potentially marginal students entering a
non-traditional MBA program. The developed neural
net model yielded an overall classification record
of 88 percent. Neural nets are only one of many
analytical screening systems that can be used for
this purpose. The capability of identifying marginal
students via analytical screening opens up the
opportunity to provide specialized learning support
material.
|
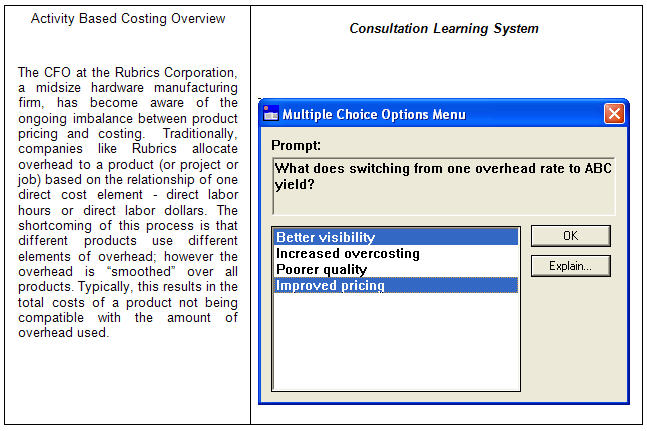
|
Figure 3.
Sample Expert System Accounting Case and
Consultation (ABC)
Web-based learning support systems provide a vehicle
to enhanced learning and are particularly attractive
for working adults engaged in an MBA program.
Specifically, consultation systems, enhanced
interactive simulations and virtual tours offer the
student a customized learning experience at a
convenient time and place. Intelligent agents are
one promising technology to support the
customization of the learning process in management
education. These tutorial systems provide learning
content based on student performance and background
characteristics. Similar systems are being used
throughout industry to improve both productivity and
effectiveness and can play a role in enhancing
student learning. The discussion shows that the
two-stage screening and content delivery process
does enhance student performance in a MBA fully
employed program. The screening and tutorial
processes should be integrated with an assessment
system. Ongoing assessment is an essential
ingredient in the delivery of graduate management
education.
References
Adya, M. Collopy, F. (1998). How effective are
neural nets at forecasting and prediction: A review
and evaluation. Journal of Forecasting, 17,
481.
Aquino, K.; Serva, S. (2005). Using a dual role
assessment to improve group dynamics and
performance. Journal of Management Education,
29,(1), 17.
Atwater, J.; Pittman, P. (2006). Facilitating
systemic thinking in business classes, Decision
Sciences Journal of Innovative Education, 4(2),
273.
Bakardjieva, M. ( 2002). Involving the virtual
subject. Ethics and Information, 2(3), 233.
Bar-llan, J. (2005). Information hub blogs.
Journal of Information Science, 31(4), 297.
Brower, H. (2003). On emulating classroom discussion
in a distance-delivered OBHR course: Creating an
on-line learning community.
Academy of Management Learning & Education,
2(1), 22.
Camuffo, A.; Gerli, F. (2004). An integrated
competency-based approach to management education.
International Journal of Training & Development,
8(4), 240.
Carver,
M.; King, T. (1994). An empirical investigation of
the MBA admission criteria for nontraditional
programs. Journal of Education for Business, 70(2),
95.
Changchit, C. (2003). An investigation into
the feasibility of using an internet-based
intelligent system to facilitate knowledge transfer.
Journal of Computer Information Systems,
43(4), 91.
Cheung, B.; Hui, L.; Zhang, J.; Yiu, S. (2003).
SmartTutor: An intelligent tutoring system in
web-based adult education, Journal of Systems &
Software; 68(1), 11.
Chewing, E.; Spiler, E. (1999). Validation of
factors relevant to the exemption of accounting
course in MBA programs. Journal of Accounting
Education, 17(2), 123.
Clark, D.; Gibb, J. (2006). Virtual Team learning:
An Introductory Study Team Exercise. Journal of
Management Education, 30 (6), 765.
Clayton, G.; Cate, Y. (2004). Predicting MBA
no-shows and graduation success with discriminate
analysis, International Advances in Economic
Research, 10(3), 235.
Clyde,
L. (2005). Educational blogging. Teacher
Librarian, 32(3), 43.
DeSanctis, G. et al. (2003). Learning in online
forums. European Management Journal, 21(5),
565.
Driver,
M. (2002). Investigating the benefits of web-centric
instruction for study learning: An exploratory study
of an MBA course. Journal of Education for
Business, 77(4), 238.
Dowling,
C.; Godfrey, J.; Gyles, N. (2003). Do hybrid
flexible delivery methods improve accounting
students’ learning outcomes? Accounting Education,
12(4), p373.
Flatley, M. (2005). Blogging for enhanced teaching
and learning. Business Communication Quarterly,
68 (1), 77.
Ghaoui, C.; Janvier, W. (2003). Interactive
E-Learning. International Journal of Distance
Education Technologies, 2(3), 26.
Graves,
W. (1999). The instructional management system
cooperative: converting random acts of progress into
global progress. Educom Review, 34(6), 32.
Hancock, T. (1999). The gender difference:
Validity of standardized admission tests in
predicting MBA performance. Journal of Education
for Business, 75(2), 91.
Hardgrave, B.; Wilson, R.; Kent, K. (1994).
Predicting graduate student success: A comparison of
neural networks and traditions techniques.
Computers & Operations Research, 21, 249.
Harrer, A.; Mclaren, M.; Walker, E.; Bollen, L.;
Sewall, J. (2006). Creating cognitive tutors for
collaborative learning: steps toward realization.
User Modeling and User – Adapted Interaction,
16(3), 175.
Haywood,
M; McMullen, D.; Wygal, D., (2004). Using games to
enhance student understanding of professional and
ethical responsibilities. Issues in Accounting
Education, 19(1), 85.
Hedlund, J.; Wilt, J.; Nebel, K.; Ashford,
S.; Sternberg, R. (2006). Assessing practical
intelligence in business school admissions: A
supplement to the graduate management admissions
test. Learning & Individual Differences,
16(2), 101.
Huang,
H. 2002. Student perceptions in an online mediated
environment, International Journal of
Instructional Media, 29(4), 408.
Huang,
J. (2005). Prerequisite change and its effect on
intermediate accounting performance. Journal of
Education for Business, 80(5), 283.
Hwang, A.; Arbaugh, J. (2006). Virtual and
traditional feedback-seeking behaviors: Underlying
competitive attitudes and consequent grade
performance, Decision Sciences of Innovation
Education, 4(1), 1.
Hynes, G. (2002). Strategies for teaching management
communications. Business Communications
Quarterly, 65, 85.
Johne, M. (2003). Virtual environments.
CMA Management,
76(10), 28.
Kathawala,
Y.; Abdou, K.; Elmuti, D. (2002). The global MBA: a
comparative assessment for its future. Journal
of European Industrial Training, 26(1), 14.
Kezim, B; Pariseau, S; Quinn, F. (2005).
Is Grade Inflation Related to Faculty Status?
Journal of Education for Business, 80(6), 358
Kiang,
M. (2003). A comparative assessment of
classification methods. Decision Support Systems,
35(4), 441.
Li, X. (2007).
Intelligent agent-supported online education,
Decision Sciences Journal
of Innovative Education,
5(2), 311.
Lundgren,
T.; Nantz, K. (2003). Student attitudes towards
Internet courses: A longitudinal study. Journal
of Computer Information Systems, 43(3), 61.
Marriott, N. (2004). Using computerized business
simulations and spreadsheet models in accounting
education: a case study. Accounting Education,
13(4), 55.
McDuffie, R.; Smith, S.; Murphy, L. (2006). Impact
of an audit reporting expert system on learning
performance: a teaching note. Accounting
Education, 15(1), 189.
Naik,
B. (2004). Using neural networks to predict MBA
student success. College Student Journal, 38
(1), 143.
Neo, T.; Neo, M. (2004). Classroom
innovation: engaging students in interactive
multimedia learning. Campus – Wide Information
Systems, 21(3), 118.
Oravec, J. (2003). Blending by Blogging: weblogs in
blended learning initiatives. Journal of
Educational Media, 28(2), 225.
Rehling, L. (2005). Teaching in a high conference
room: Academic adaptations and workplace
simulations. Journal of Business and Workplace
Simulations, 19(1), 98.
Saunders,
G.; Ellen, J.; Christopher, R. (2003). Teaching
outside the box: A look at the use of some
nontraditional teaching models in accounting
principles courses,” Journal of
American
Academy of Business,
3(1), 162.
Shih,
Y. (2003). A survey of distance education challenges
and technologies. International Journal of
Distance Learning Technologies, 1(1), 1.
Sireci, S. (2006). Evaluating the predictive
validity of graduate management admission test
scores, Educational & Psychological Measurement,
65(2), 305.
Stout, D.; Borden, J.; German, M.; Monahan, T.
(2005). Designing and implementing a comprehensive
assessment plan for a graduate accounting program.
Accounting Education, 14(4), 395.
Wilson, R.; Hardgrave, B. (1995). Predicting
graduate student success in an MBA program:
Regression versus
classification,
Educational & Psychological
Measurement; 55(2), 186.
Yang, B.; Lu, D. (2001). Predicting academic
performance in management education:
An empirical investigation of MBA success.
Journal of Education for Business, 77(1), 15.
Xu, D.; Wang, H. (2006). Intelligent agent supported
personalization for virtual learning environments,
Decision Support Systems, 42(2), 825.
|
|
|
|
|