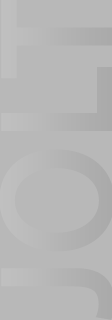 |
MERLOT Journal of Online
Learning and Teaching |
Vol.
6, No. 3, September 2010
|
Underlying Factors of Sense of Community in Asynchronous Computer Supported Collaborative Learning Environments
Factores Fundamentales de Sentido de Comunidad en Ambientes de Aprendizaje
Colaborativo Apoyado por Computador sin Cronología
|
Abstract
With the proliferation of online and distance courses human factors and social dimensions of computer supported collaborative learning (CSCL) environments are becoming more important than before. Research shows that development of a sense of community among individuals in the CSCL environments improves learning outcomes in such environments. The objective of this study is to examine the underlying dimensions of sense of community. Through an exploratory factor analysis two constructs have emerged: Individual’s sense of cohesion and awareness of others. Identification of these factors facilitates a better understanding of sense of community in CSCL environments, which in turn will guide the design of CSCL environments. In addition, the present paper examines the relationships between the facilitating factors of CSCL and the extracted factors in order to assess changes in individuals’ behavior. These factors include learner’s characteristics, course characteristics, instructor characteristics, and technical characteristics.
Keywords : Sense of community, Collaborative learning, Awareness of others, Sense of cohesion
|
Resumen
Debido a la proliferación de los cursos online y a distancia, los factores humanos y la dimensión social del medio ambiente de aprendizaje colaborativo apoyado por computador (CSCL) es cada día más importante. La investigación indica que el desarrollar un sentido de comunidad entre los individuos en el ambiente mejora el resultado del aprendizaje y el logro en dichos ambientes. El objetivo principal de este estudio es examinar las dimensiones fundamentales del Sentido de Comunidad. A través de un análisis exploratorio del factor realizado sobre una muestra de estudiantes postgraduados de un curso online de grado académico en una universidad Australiana, dos interpretaciones han sido descubiertas: el Sentido de Cohesión Individual y el Conocimiento de Otros Se espera que la identificación de estos factores faciliten una mejor comprensión del Sentido de Comunidad en los ambientes CSCL, que a su vez guiarán el diseño de los sistemas CSCL. Además, el presente documento examina la relación entre las características ambientales del CSCL, y los factores extraídos para evaluar los cambios en el comportamiento de las personas. Estas características ambientales incluyen las características del estudiante, características del curso, características del instructor, y características técnicas.
Palabras claves: Sentido de comunidad, aprendizaje online, aprendizaje colaborativo, Conocimiento de otros, Sentido de cohesión. |
Introduction
Computer Supported Collaborative Learning (CSCL) incorporates collaborative learning activities and communication between participants and among groups via networked computers, where interactions can be synchronous or asynchronous (Naidu & Ja¨rvela, 2006). The ubiquitous characteristic of CSCL environments, combined with their interactive group learning capabilities is becoming more and more important in education ( Strijbos & Weinberger, 2009; Janssen, Erkens, Kanselaar, & Jaspers 2006; Kreijns, Kirschner, & Jochems, 2002). Yet, while technologically those environments have considerably improved over recent years, studies have shown that the social dimension of CSCL is often neglected or assumed it will arise by default in these virtual learning environments (Kreijns, Kirschner, & Jochems, 2003). It is also reported that although such environments offer a variety of benefits, learners’ isolation and lack of sense of community are emerging as key pitfalls for effectiveness of those environments and therefore, deserve greater effort in future development efforts (Gunawardena, 1995; McPherson & Nunes, 2004).
A sense of community can be defined as the opposite of a sense of isolation and being out there on your own (Walker, 2008). It is believed that the notion of community is at the heart of learning (Nichani & Hung, 2002), in general, and computer supported collaborative learning, in particular (Wallace, 2003; Wrescha, Arbaugha, & Rebstock, 2005). The importance of a strong sense of community in CSCL environments for learning achievements and outcomes has been previously discussed. Walker (2008) pointed out that students with a strong sense of community are more likely to continue and succeed in the CSCL experience than students who feel separated from the community. Wegerif (1998) and Sadera, Robertson, Song, and Midon (2009) assessed the CSCL environments and emphasized a strong and positive relationship between the sense of community and learning outcomes. Rovai (2001, 2002) and Rovai, Wightinga, and Lucking (2004) also emphasized the importance of sense of community and avoidance of sense of isolation in the CSCL. They emphasized that a strong feeling of community enhances the flow of information among students, social support, commitment to group goals, and consequently, satisfaction with the learning experience.
Given the importance of a strong sense of community in the computer supported collaborative learning, the present study attempts to investigate and measure students’ perception of sense of community in an Australian postgraduate CSCL environment. While several previous studies have attempted to measure sense of community in CSCL environments, none of them has explicitly identified the underlying dimensions of this concept. Yet, findings of this paper have revealed that Awareness of Others and Sense of Cohesion are two distinct underlying dimensions of the sense of community in the CSCL. A further objective of this study is to assess the effect of facilitating factors on sense of community. Facilitating factors in this study are Learner’s Characteristics, Instructor’s Characteristics, Course Characteristics, and Technical Characteristics. The importance of this analysis is due to the fact that these four characteristics are generic factors that exist in all CSCL environments. Therefore, identification of deterministic characteristics will be useful in future CSCL designs for improving students’ perceived sense of community.
Review of Instruments in the Literature
This section reviews the previous instruments in the CSCL literature as well as the literature of virtual teams, online community, and online distance learning. The terms virtual team and online distance learning have often been used for studies where learning occurs through computer mediated collaboration and interactions between people, and therefore the instruments used in such areas have been considered in this present paper to create a larger pool of items for sense of community. Salisbury, Carte, and Chidambaram (2006) studied the characteristics of virtual teams and assessed people’s sense of cohesion in virtual environments. They developed the ‘Perceived Cohesion Scale for Virtual Teams’ which offers six measures for measurement of sense of cohesion within virtual teams. Salas, Kosarzycki, Bruke, Fiore, and Stone (2002) identified five major themes in distance learning research and reported that spirit of community, trust, cohesion, liking and attractions, are the latent factors of the social side of distance learning. Rovai (2002) developed the ‘Classroom Community Scale’ to measure sense of community in online communities. This 20-measure scale, however, does not recognize subcategories and possible underlying dimensions of sense of community. In another study, Cadieux (2002) proposed an index for online college communities. This study reviewed the literature and gathered a pool of measures for sense of community and built a forty-measure Sense of Class Community Index (SCCI). Summers, Beretvas, Svinicki, and Gorin (2005) investigated the social environment of online learning communities and stressed the importance of effect of social connectedness on better learning achievements. They measured social connectedness as well as students’ feeling of community in the class in order to measure individual’s perception of social environment. While Sense of Community Index (SCI) has been originally developed for face-to-face communities, Brook and Oliver (2002) extended this index to online environments. The objective of their study was to modify SCI based on particular characteristics of online learning communities and to develop an index to measure online individuals’ perception of sense of community.
In addition to the review of studies that have attempted to operationalize sense of community, this present paper has also reviewed the literature to seek the facilitating factors that may impact sense of community.
It is intended to examine whether learners’ perception of sense of community in the CSCL environments varies based on changes of any of the facilitating factors. These facilitating factors are Individual’s Characteristics, Instructor Characteristics, Course Characteristics, and Technical Characteristics which have been borrowed from Brandon and Hollingshead’s (1999) Model of CSCL Theory and Research. The Model of CSCL Theory and Research provides a clear and comprehensive structure for major influential issues in the literature of CSCL. This model has identified input and output variables in the CSCL process. The outputs include students’ knowledge and satisfaction which arise through their participation, group activities and cognitive processes. The input variables include technical characteristics, course characteristics, instructor characteristics, and learners’ characteristics as well as social and community characteristics.
Brandon and Hollingshead (1999) mentioned that instructors can encourage students to participate in CSCL activities, and their behavior may impact the students’ level of online activities. Moreover, Tolmie and Boyle (2000) suggested that the clarity of tasks in online classes as well as technical support of online classes may influence students’ willingness to have more interaction and participation in the CSCL environments. In another study, Dutton and Dutton (2002) compared online classes with traditional face-to-face classes and argued that students in online classes are different from students in traditional classes. The study then explored factors that influence the behavior and performance of online students and found out that marital status and learners’ experience with computers were among learners’ characteristics that might influence their behavior in the environment. Dutton and Dutton (2002) also reported that the level of study is a course characteristic that may influence Individual’s online performance and behavior.
In another study, the provision of technical training to students as well as the level of information and help provided to use CSCL courses was reported as other factors that may impact on students’ interactions in CSCL classes (McMahon, Gardner, Gray, & Mulhern, 1999). Students’ level of access to the instructors as well as the number of enrolled and dropped courses is among these other factors that may influence students’ interaction in the CSCL environments (Daradoumis, nez-Mone´sb, & Xhafa, 2006; Muilenburga & Berge, 2005). In addition to the above factors, this present study has also included age, sex and work experience as learners’ characteristics in the analysis. The above factors are summarized in Table 1.Data Collection
The measures from the reviewed instruments were put together and then similar or repeated measures were consolidated. The remaining measures were considered for designing a self-reported questionnaire for this present study. Table 2 shows selected measures from the reviewed instruments with a short description for each item. A five-level Likert-scale questionnaire was designed, and questions were randomly ordered. In the questionnaire, students’ perception about each measure was rated from strongly disagree to strongly agree. The questionnaire was published in an external website and a short description of the study along with a link to the questionnaire was e-mailed to the students. Face validity of the questionnaire was examined, and a copy of the questionnaire was sent to a professional editor for further English and grammatical checks. Four information systems doctoral candidates were asked to read the questionnaire and send their comments regarding formatting, length, and layout of the questionnaire. They were also asked to comment about any ambiguities in the questionnaire arising from the wording of the questions.
Factor Analysis
The context of this study was a postgraduate general management degree offered by a large Australian university. Entry to this degree program is based on appropriate work experience, a prior degree and being employed full-time. Full-time study in the program is not permitted as being currently employed is an important component of the learning model in this postgraduate program. Most students study one to three courses per semester. Courses are offered over three semesters each year and are offered both online and face-to-face. Students taking the courses online are not required to attend any face-to-face learning activities. In each session, 80% of students choose to study in the online mode with the remaining 20% attending face-to-face classes. The vast majority of the student cohort, 92%, is domiciled in Australia. The data were collected during the 2008-2009 summer term which spanned ten weeks over the southern-hemisphere summer that includes the Christmas and New Year period. Two hundred students were enrolled in the ten courses offered. Summer terms are accelerated in that the normal 12 weeks of content is presented over 6 weeks.
Table 1: Facilitating factors.
Category |
Variable |
Description |
Study |
Learners’ Characteristics
(LC) |
Students’ experience with the computer technology |
It might promote their participation in CSCL |
(Tolmie & Boyle, 2000); (Dutton & Dutton, 2002); (Brandon & Hollingshead, 1999) |
Marital Status |
Single , married |
(Dutton & Dutton, 2002) |
Age |
- |
- |
Sex |
- |
- |
Work experiences |
- |
- |
Course Characteristics
(CC) |
Clarity of tasks in online courses |
More clarity increases participation |
(Tolmie & Boyle, 2000) ; (Brandon & Hollingshead, 1999) |
Number of enrolled courses |
- |
(Muilenburga & Berge, 2005) |
Number of dropped courses |
- |
Level of degree |
UnderGrad/PostGrad |
(Dutton & Dutton, 2002) |
Technical Characteristics
(Thatcher) |
Technical support |
Level of receiving support from staff or computer administrators for system configurations and possible failures |
(Tolmie & Boyle, 2000) ; (Benbunan-Fich & Hiltz, 2003; Brandon & Hollingshead, 1999); (McMahon et al., 1999) |
Technical training |
Level of training provided for students to acquire computer skills |
(McMahon et al., 1999) |
Lecturer / Instructor Characteristics
(IC) |
Level of Discussion facilities’ Information |
The level of provided information about discussion facilities |
(McMahon et al., 1999) |
Instructor’s support and access |
It helps students to higher learning achievements |
(Daradoumis et al., 2006) |
Instructor’s behavior |
Instructor can encourage students’ participation |
(Brandon & Hollingshead, 1999) |
Of the 200 students who received the instrument, 41 responded, representing a response rate of 25%. One response was removed as most of the questions were unanswered. Of the 40 remaining survey respondents, 27 (67.5%) were male, 13 (32.5%) were female, 12 (30%) were single, and 28 (70%) were married. The average age of the participants was 37.5 years, ranging from 27 to 54 years. The average work experience was 17.7 years, and this ranged from 8 to 30 years.
Table 2: Measures representing Sense of Community.
Code |
Measure |
Description |
SC1 |
Feeling of connectedness |
The extent to which one feels connected to others in the community |
SC2 |
Sense of Isolation (Feeling lonely) |
The extent to which one feels lonely in this environment |
SC3 |
Cohesion |
The degree to which people care about others and have sense of cohesion |
SC4 |
Spirit |
The feelings of friendship, cohesion, and bonding, sense of connectedness, to feel a part of and be included in the group |
SC5 |
Respect in relationship |
The degree to which people respect each other in their interactions in the environment |
SC6 |
Trust |
Represents a willingness to rely on other members of the community in whom one has confidence |
SC7 |
Interdependence among others |
Refers to non-task relationship between people and disclosure of personal information |
SC8 |
Sense of belonging |
The extent one feels he/she belongs to the community |
SC9 |
Sense of membership |
The degree one perceives is accepted as a member of community |
SC10 |
Sense of influence |
The extent to which one has sense of influence on what happens in the environment |
SC11 |
Shared emotional connection |
Ones’ perception about how much people have similar understandings and interests |
SC12 |
Awareness |
Feeling that people are aware of and care about what happens in the environment |
An exploratory factor analysis (EFA) was performed. To determine appropriateness of factor analysis for this present study, Bartlett’s tests of sphericity was carried out and indicated existence of correlations among measures (Sig. = 0.000). Also, to examine sampling adequacy, a test of Kaiser–Meyer–Olkin (KMO) was performed which indicated a satisfactory level of 0.809. The axis-factoring method was chosen as the factor analysis extraction method. This was due to the argument offered by Hair, Anderson, Tatham and Black (1995) that the use of common factoring methods (including Axis-factoring) is suggested in situations where little knowledge is available about unique error variance. Nevertheless, an unrestricted rotation method was selected which Gorsuch (1997) recommended for situations where the researcher has no prior knowledge about possible correlation between constructs. Therefore, the Promax rotation solution with kappa=4 (which is one of unrestricted rotation methods) was chosen as the rotation method for the EFA analysis.
The first run of the analysis extracted three constructs with eigenvalues greater than one. A five step measure-selection approach was taken to rigorously select appropriate measures. The first step examined measures’ communalities. Fabrigar, Maccallum, Wegener, and Strahan (1999) discussed that while eigenvalues greater than one is a popular criterion in factor selection, it is not sufficient in factor analysis and must be used in parallel with other methods. They pointed out that researchers can examine measures’ communalities to find unnecessary measures for each construct. Communality is a proportion of each measure’s variance that can be explained by the constructs. A higher communality for each measure is desired as it shows a higher level of explained variances by the extracted construct. In this present study a communality of 0.5 was chosen as the threshold since we realized a value greater than that may cause over dropping of measures. Table 3 shows communality of measures after the first run of the analysis. The bolded measures with initial and extraction communality of less than 0.5 were nominated to be removed from the analysis. Yet, before removing them and running the EFA again, we wanted to ensure that, according to Moore and Benbasat (1991), removing a specific measure would not make the domain coverage suffer. Therefore, the second step of our measure-selection approach was to subjectively decide dropping those measures which might make the domain coverage suffer. Through a discussion among authors, it was concluded that dropping SC6 (trust) and SC2 (sense of loneliness) might negatively affect the domain coverage of ‘Sense of Community’, especially because these two measures have been used in many previous studies. Therefore, at this stage these two measures were retained for further analysis. After removing other nominated measures the factor analysis was run again and nine measures loading on two factors resulted.
Table 3. Primarily EFA, Communalities
(Extraction Method: Principal Axis Factoring)
Measure |
Initial |
Extraction |
SC1 |
.726 |
.705 |
SC2 |
.472 |
.446 |
SC3 |
.792 |
.845 |
SC4 |
.590 |
.555 |
SC5 |
.355 |
.267 |
SC6 |
.362 |
.432 |
SC7 |
.680 |
.637 |
SC8 |
.733 |
.809 |
SC9 |
.673 |
.954 |
SC10 |
.348 |
.208 |
SC11 |
.424 |
.263 |
SC12 |
.629 |
.582 |
The third step was finding salient constructs. Gorsuch (1997) suggested that among extracted constructs, only those that have at least three salient measures should be considered where salient was defined as ‘a loading that is greater than |0.4| and is highest loading for the variable’. The benefit of this criterion is to avoid selection of constructs that only have one or two measures load on them. The loadings of measures on the resulted factors showed that both of them were salient and no violation was detected.
The forth step examined constructs against their reliability level. Moore and Benbasat (1991) pointed out that for each extracted construct, those measures which have no positive effect on the reliability level should be dropped. This ensures that the remaining measures have remarkable contribution in representing their corresponding construct. They mentioned that for the purpose of a pilot study with small sample size, the level of reliability ( α) depends on the nature of each study. But in general, while a level of 0.50 - 0.60 could be sufficient, a reliability level of 0.70-0.80 was recommended. As Table 4 shows, SC4 and SC6 were therefore nominated to be dropped from the analysis.
Finally in the fifth step, the remaining measures were examined against experts’ judgments. Collection and analysis of experts’ judgments were performed as part of the content validity of the study to better examine which measures did not reasonably represent their corresponding factor. Details of content validity are explained in the next section, and the result is reported in this section. As shown in the third row of Table 4, the results of the content validity indicates measures that were nominated to be dropped from the analysis. Some of the measures that were suggested by the CSCL researchers to be removed in this table had been already nominated in previous steps.
Table 4. Examining factors against reliability level and expert’s judge
Variable/Factors
Reliability and explained variance |
Sense of Community |
Construct 1 |
Construct 2 |
Initial Reliability ( a) |
0.768 |
0.886 |
Reliability can be improved by removing measure |
SC6 |
- |
Nominated measures to be removed by experts’ judge |
SC6, SC4 |
- |
Reliability ( a) after removing measures |
0.909 |
0.886 |
Total explained variance by extracted factors |
75.49% |
Table 5 shows the loadings of measures on their corresponding constructs. Exploratory factor analysis on sense of Community uncovered two constructs. The first construct has four measures: sense of independence among others, connectedness, belonging, and cohesion. This construct is labeled ‘sense of cohesion’ because the above four measures reflect a similar concept and also because sense of cohesion has the highest loading on this construct. The second factor includes three measures: sense of loneliness, membership, and awareness. According to their definitions in Table 2, these three measures reflect an individual’s knowledge of others and general awareness of what happens in the environment. Awareness is the measure with the highest loading, and therefore, this constructs is named ‘awareness of others’. This measure also closely resembles the notion of level-2 awareness according to the ‘awareness net’ modeling language ( Daneshgar & Wang, 2007).
Table 5. Results of Exploratory Factor Analysis (Pattern Matrix)
Code |
Measure name |
Reliability
(α) |
Factor |
Sense of Cohesion |
Awareness of Others |
SC1 |
Feeling of connectedness |
0.909 |
.789 |
.091 |
SC3 |
Cohesion |
.884 |
.047 |
SC7 |
Interdependence among others |
.734 |
.120 |
SC8 |
Sense of belonging |
.908 |
-.117 |
SC2 |
Sense of isolation |
0.886 |
.048 |
.813 |
SC9 |
Sense of membership |
.130 |
.444 |
SC12 |
Awareness |
-.069 |
.904 |
Extraction Method: Principal Axis Factoring. Rotation Method: Promax with Kaiser Normalization. Rotation converged in 3 iterations.
The Impact of the Facilitating Factors on Sense of Community
This section examines the impact of individual’s characteristics, course characteristics, technical characteristics, and instructor characteristics on students’ perception of sense of cohesion and awareness of others, as the underlying factors of sense of community.
Partial Least Square (PLS) technique has been used to assess the relationship between the constructs in Figure 1 PLS-Graph (Version 3.00) has been used to assist with this analysis. Partial Least Squares (PLS) is recommended for assessing interrelationships in models with a small sample size (Gefen & Straub, 2005). PLS computes t-values which show significance of coefficients of the relationship between independent and dependent factors. To achieve t-values, bootstrapping was executed. Figure 1 shows the path coefficients as well as significance of the coefficients. According to Figure 1, learner characteristics is the only factor that has a significant impact on both sense of cohesion and awareness of others. Moreover, learner characteristics has a significant impact on individuals’ awareness of others. Technical characteristics also influences sense of cohesion. The remaining coefficients are not significant. The implications of these findings are discussed later in this paper.
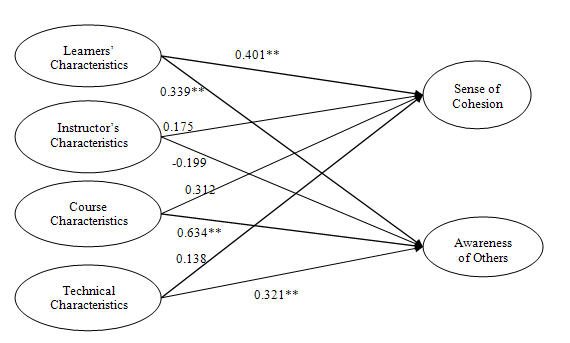
Figure 1. Effect of the facilitating factors on Sense of Cohesion and Awareness of Others (significant relationships are flagged by ‘**’ ( a = 0. 01))
Reliability and Validity of the Study
Table 5 earlier revealed a strong reliability level for the extracted constructs. One reason for achieving a high reliability for the extracted constructs in this study is the use of a strict five-step measurement selection approach. Through this approach, measures with a high loading on the corresponding factors were carefully screened and chosen.
Furthermore, the convergent validity and discriminant validity of the findings have been also assessed. Convergent validity tests whether the measures are most closely associated with their corresponding factors. Straub, Boudreau, and Gefen (2004) pointed out that convergent validity is evidenced when the measures for various factors converge or highly correlate with one another, specially comparing to their correlation to other factors. As Table 6 shows, all t-values are significant at the alpha level of 0.05.
Discriminant validity examines whether the measures that represent a construct differ from those that are not believed to make up that construct (Straub et al., 2004). To assess discriminant validity, two criteria must be satisfied ( Gefen & Straub, 2005) :
Each measure must highly load on its corresponding factor.
Average Variance Extracted (AVE) numbers in the PLS must be calculated. It must be checked if the square root of the AVE value of each factor is higher than the correlation among any pairs of the constructs.
Table 6. t-values for convergent validity test ( α = 0.05)
Variable/measures |
Original sample Estimate (loading) |
Mean of
subsamples |
t-value |
Sense of Cohesion SC1
SC3
SC7
SC8 |
|
|
17.554
37.273
22.646
19.111
|
Awareness of Others SC2
SC9
SC12 |
|
|
|
These two criteria assure that constructs can be discriminated from one another. The first criterion has already been satisfied since according to Table 6, all significant measures have loaded on their corresponding construct. In order to check the second criterion, the AVE values as well as correlations between latent variables were considered. Table 7 shows the correlation between the two factors of this study where diagonal numbers are bolded and represent the square root of AVE of each factor. As this table shows, in each column, the AVE value of each factor is higher than the correlation between them, indicating existence of convergent validity of the study.
Table 7. Discriminant validity analysis.
|
Sense of Cohesion |
Awareness of Others |
Sense of Cohesion |
0.891 |
|
Awareness of Others |
0.583 |
0.833 |
In addition to the above tests, content validity of the study was also examined. Content validity deals with the question as to whether the measures in a study converge in a manner that could be used to represent and measure the content of given construct (Straub et al., 2004). Content validity is established through expert opinions as well as through literature review. Content validity has been performed in this present paper with two objectives: first, to examine if the chosen measures reasonably represent sense of community; and second, if not, which measure (s) should to be excluded from the study. Sixty five CSCL researchers from different countries in the area of CSCL were identified from the related literature. The definition of sense of community as well as the measures in Table 2 and their corresponding definitions were listed in an online form and the link of this form was e-mailed to these researchers. A brief description of the study and methodology was also outlined. Three questions were asked: (1) All these measures combined, reasonably represent the definition of the construct? (Yes/No); (2) If No, then please list the measure (s) that do not reasonably represent the construct; (3) Any comments on this issue will be very much appreciated.
Twenty-two responses were received. The results were promising, as 86% of the respondents confirmed that the current set of measures reasonably represents Sense of Community in the CSCL environments. These findings confirmed that the pool of measures from different sources represent the same concept. Moreover the following comments were received:
(1) ‘Spirit’ (SC4) and ‘Trust’ (SC6) should be removed,
(2) ‘Feeling lonely’ should be renamed to ‘Sense of isolation’, and
(3) The extracted constructs from EFA analysis must carefully be defined in order to clearly show distinction between the factors and their corresponding measures.
Therefore, as Table 4 shows, ‘Spirit’ and ‘Trust’ has been nominated to be removed from the analysis. Also, Table 2 shows that ‘feeling lonely’ was renamed to ‘sense of isolation’. Furthermore, to address the third comment, a discussion has been provided in the next section to further distinguish sense of cohesion from awareness of others, as the two factors extracted from sense of community.
Research Limitations
As the average age of participants in this study was 36 and they were enrolled part-time, the present study could therefore be extended to incorporate different cohorts of university students, and as a result, more comprehensively identify students’ requirements of the CSCL environments. Another limitation is the moderate sample size of the study. While this was due to the limited number of students enrolled in the summer semester, the 25% response rate still indicates a reasonable sample size for representing the overall population. Moreover, the results of Bartlett’s tests of sphericity and test of Kaiser–Meyer–Olkin (KMO) assured us of the existence of correlations among measures as well as sampling adequacy, respectively.
Discussion and Conclusion
While many previous studies have investigated sense of community in the CSCL environments, none of them has identified underlying dimensions of the sense of community. In contrast, the present study has revealed individuals’ sense of cohesion and awareness of others as two underlying dimensions of sense of community. A five-step measurement selection approach along with validity tests and a confirmation from CSCL researchers confirms that all chosen measures belonged to the sense of community.
Theoretically, these findings are important because they imply that individuals’ perception of sense of cohesion and awareness of other group members’ activities are two complementary components of sense of community in CSCL. Sense of cohesion addresses individuals’ emotional ties with the group members such as liking, caring, and connectedness. This bonds students together and creates a sense of belonging to the CSCL community whereas awareness of others represents an individual’s perception of the degree to which he/she is sensitive to the actions created by other individuals in the community.
Practically, the results of this present study facilitate a better understanding of sense of community and provide guidelines for more sociable CSCL developments. Currently, many CSCL environments enable students to create personalized profiles and provide virtual spaces, such as online Coffee Shop for off-task discussions. This serves to get students more engaged in learning activities, to improve students’ connectedness, and to enhance sense of belonging in the learning environment. Yet, the findings of this present study suggest that to achieve such goals, CSCL developers should also add features and capabilities to the environment, which enable students to be aware of other’s activities and status. One example of efforts which implies complementariness of awareness and cohesion and also practically operationalizes awareness in CSCL environment is the group awareness widget developed by Kirschner, Strijbos, Kreijns, and Beers (2004). They emphasized the role of effective social relationships on learning performance in CSCL environment and discussed approaches to improve effective social relationships. Group awareness widgets may help with the three following functionalities: (1) graphically present group awareness information for achieving tele-proximity, (2) graphically present history awareness in terms of a structured way of showing footprints of people in the environment, and (3) communication media for coupling perception-action.
Another finding of the present study is that among the four facilitating factors, Learners’ Characteristics is the only one that has a significant impact on Sense of Cohesion. This implies that perceived Sense of Cohesion varies in the CSCL environments based on different Learners’ Characteristics, such as age, gender and marital status. Yet, Instructor’s Characteristics did not show a significant impact on either of the dependent factors. Therefore, while previous studies report that Instructor’s characteristics and attitude may have positive impact on perceived learning performance (Abrantes, Seabra, & Lages, 2007), the results of this study suggest further studies are required to investigate instructors’ role in the social and community aspect of CSCL environments. Moreover, individual’s Awareness of others is strongly affected by Technical Characteristics and Course Characteristics, which shows that a more enhanced technical feature of the learning technology and a better organization of courses may lead to a higher level of students’ awareness of other people in the CSCL environment.
In conclusion, this paper has examined the underlying dimensions of sense of community in a CSCL environment and revealed that individual’s sense of cohesion and awareness of others are two underlying factors of sense of community. This finding is important, as each extracted factor represents a different aspect of sense of community. In addition, this paper shows that amongst the facilitating factors, Learner’s Characteristics significantly impacted one’s sense of cohesion, and Technical Characteristics and Course Characteristics significantly affected awareness of others. Overall these findings provide a better understanding of Sense of Community in CSCL environments and facilitating factors that impact it, which in turn may help guide future design of CSCL environments.
|
References
Abrantes, J. L., Seabra, C., and Lages, L. F. (2007). Pedagogical affect, student interest, and learning performance. Journal of Business Research, 60, 960-964.
Benbunan-Fich, R., and Hiltz, S. R. (2003). Mediators of the Effectiveness of Online Courses. IEEE Transactions on Professional Communication, 46 (4), 298-312.
Brandon, D. P., and Hollingshead, A. B. (1999). Collaborative learning and computer supported groups. Communication Education, 48.
Brook, C., and Oliver, R. (2002). Supporting The Development Of Learning Communities In Online Settings. Paper presented at the Ed-Media.
Cadieux, C. P. (2002). Variables Associated with a Sense of Classroom Community and Academic Persistence in an Urban Community College Online Setting. OLD Dominion University.
Daradoumis, T., nez-Mone´sb, A. M., and Xhafa, F. (2006). A layered framework for evaluating online collaborative learning interactions. International Journal of Human-Computer Studies, 64, 622-635.
Dutton, J., and Dutton, M. (2002). HOW DO ONLINE STUDENTS DIFFER FROM LECTURE STUDENTS? Journal of asynchronous learning networks, 6 (1), 1-20.
Fabrigar, L. R., Maccallum, R. C., Wegener, D. T. & Strahan, E. J. (1999) Evaluating the Use of Exploratory Factor Analysis in Psychological Research. Psychological Methods, 4, 272-299.
Gefen, D., and Straub, D. (2005). A Practical Guide To Factorial Validity Using PLS-Graph: Tutorial And Annotated Example. Communications of the Association for Information Systems, 6, 91-109.
Gunawardena, C. N. (1995). Social Presence Theory and Implications for Interaction and Collaborative Learning in Computer Conference. International Journal of Educational Telecommunications, 1 (2/3), 147-166.
Hair, J. F., Anderson, R. E., Tatham, R. L. & Black, W. C. (1995). Multivariate Data Analysis with readings, New Jersey, Prentice Hall.
Kirschner, P. A., Strijbos, J.-W., Kreijns, K., and Beers, P. J. (2004). Designing Electronic Collaborative Learning Environments. Journal of educational technology research and development, 52 (3), 47-66.
Kreijns, K., Kirschner, P. A., and Jochems, W. (2003). Identifying the pitfalls for social interaction in computer-supported collaborative learning environments: a review of the research. Computers in Human Behavior, 19, 335-353.
McMahon, J., Gardner, J., Gray, C., and Mulhern, G. (1999). Barriers to student computer usage: staff and student perceptions. Journal of Computer Assisted Learning, 15, 302-311.
McPherson, M., and Nunes, M. B. (2004). The failure of a virtual social space (VSS) designed to create a learning community: lessons learned. British Journal of Educational Technology, 35 (3), 305-321.
Moore, G. C., and Benbasat, I. (1991). Development of an Instrument to Measure the Perception of Adopting and Information Technology Innovation. Information Systems Research, 2 (3), 192-222.
Muilenburga, L. Y., and Berge, Z. L. (2005). Student Barriers to Online Learning: A factor analytic study. Distance Education, 26 (1), 29-48.
Naidu, S., and Ja¨rvela, S. (2006). Analyzing CMC content for what? Computers and Education, 46, 96–103.
Nichani, M., and Hung, D. (2002). Can a Community of Practice Exist Online? Educational Technology.
Rovai, A. P. (2001). Classroom community at a distance A comparative analysis of two ALN-based university programs. Internet and Higher Education, 4, 105-118.
Rovai, A. P. (2002). Development of an instrument to measure classroom community. Internet and Higher Education, 5, 197–211.
Rovai, A. P., Wightinga, M. J., and Lucking, R. (2004). The Classroom and School Community Inventory: Development, refinement, and validation of a self-report measure for educational research. Internet and Higher Education, 7, 263-280.
Sadera, W. A., Robertson, J., Song, L., and Midon, M. N. (2009). The Role of Community in Online Learning Success. MERLOT Journal of Online Learning and Teaching, 5 (2), 227-284.
Salas, E., Kosarzycki, M. P., Bruke, C. S., Fiore, S. M., and Stone, D. L. (2002). Emerging themes in distance learning research and practice: some food for thought. International journal of management reviews, 4 (2), 135-153.
Salisbury , W. D., Carte, T. A., and Chidambaram, L. (2006). Cohesion in Virtual Teams: Validating the Perceived Cohesion Scale in a Distributed settings. Database for Advances in Information Systems, 37 (2/3), 147-155.
Straub, D., Boudreau, M.-C., and Gefen, D. (2004). Validation Guidlines For IS Positivist Research. Communications of the Association for Information Systems, 13, 380-427.
Summers, J. J., Beretvas, S. N., Svinicki, M. D., and Gorin, J. S. (2005). Evaluating Collaborative Learning and Community. The Journal of Experimental Education, 73 (3), 165-188.
Thatcher, A. (2006). Building and maintaining an online academic conference series. International Journal of Industrial Ergonomics, 36 (12), 1081-1088.
Tolmie, A., and Boyle, J. (2000). Factors infuencing the success of computer mediated communication (CMC) environments in university teaching: a review and case study. Computers and Education, 34, 119-140.
Walker, B. K. (2008). Bridging the Distance: How Social Interaction, Presence, Social Presence, and Sense of Community Influence Student Learning Experiences in an Online Virtual Environment. The University of North Carolina at Greensboro, Greensboro.
Wallace, R. M. (2003). Online Learning in Higher Education: a review of research on interactions among teachers and students. Education, Communication and Information, 3 (2), 241-280.
Wegerif, R. (1998). The Social Dimension of synchronous Learning Networks. Journal of asynchronous learning networks, 2 (1).
Wrescha, W., Arbaugha, J. B., and Rebstock, M. (2005). International online management education courses: A study of participation patterns. Internet and Higher Education, 8, 131-144.
|
|
|
|
|
|