Assessing Faculty Attitudes Toward Technological Change
in Graduate Management Education
|
Owen P. Hall, Jr.
Professor of Decision Sciences
Graziadio School of Business and Management
Pepperdine University
Malibu, CA 90263 USA
owen.hall@pepperdine.edu
Abstract
Distance learning has come a long way since Sir Isaac Pitman initiated the first correspondence course in the early 1840s. The changing demands of the global business community call for new and innovative learning systems for enhancing graduate management education. Learning technologies offer an approach for meeting these challenges. However, faculty resistance to change can represent a major barrier to more fully implementing this learning paradigm. The purpose of this paper is twofold: (1) to highlight the results of a survey on faculty perceptions regarding the role of new learning technologies in graduate management education; and (2) to outline an approach for helping bridge the gap between faculty adoption of new learning technologies and the demand for change in delivering world-class business education.
Keywords: graduate management education, Rogers' innovation model, neural nets, classification and regression tree (CART) analysis, learning technologies, learning management system (LMS), implementation strategies, faculty attitudes, faculty collaboration |
Introduction
Graduate management education is undergoing a seismic change as a result of changing worldwide dynamics (Daniel, Kanwar, & Uvalic-Trumbic, 2006; Hawawini, 2005). Traditional business schools will either transform themselves to meet these growing challenges from businesses, governments, and students or lose market share to alternative providers of business education (e.g., for-profit institutions). Some of the factors driving this shift include globalization, changing student demographics, increasing interest in sustainability, and enabling technology. The same networking and computing systems that revolutionized global commerce in the 1990s and 2000s are now bringing about the technical reformation in management education. Integrating the Internet with the new learning technologies makes it possible for institutions of higher learning to offer a variety of student-centric programs (e.g., online) on a global basis (Dykman & Davis, 2008). For example, a web-based learning management system (LMS) can provide high quality curricula and content at a time and place convenient to the student (Nor Aziah Alias & Ahmad Marzuki Zainuddin, 2005). LMSs also offer the student both a customized and an integrated learning experience and can be used for learning outcomes assessments. Furthermore, LMSs provide flexible content to students in both individual and collaborative contexts. For working adult management programs, flexibility and convenience are a growing requirement. Many working professionals are finding it increasingly difficult to attend class on a routine basis due to work related demands like travel. This is where technology can make a difference. Some of the design principles associated with adopting new learning technologies like LMSs include:
- Knowledge and information presented over multiple learning platforms;
- Learning material presented in digital and reusable formats;
- Content delivery independent of time and space;
- Knowledge and information presented in small "bite-sized" chunks.
The faculty represents the essential ingredient in transitioning from the traditional classroom format to a web-oriented learning environment (blended or online). There are a number of factors that need to be addressed to insure that the faculty members are able to make the transition from the traditional classroom environment to the virtual world of web-based learning. Three specific factors are incentives, training, and collaboration. This paper's primary contribution to the field of online learning is the methodological approach and corresponding implementation strategies. The paper is organized as follows: (1) a review of the relevant literature; (2) an introduction into data mining techniques (neural nets and classification and regression trees – CART) used in the analysis; (3) an analysis of the data obtained from a recent faculty survey; and (4) a discussion on the implications as related to curriculum and programs.
"Thirty years from now the big university campuses will be relics."
– Peter Drucker, in an interview with Forbes magazine (Lenzner & Johnson, 1997, p. 126)
Literature Review and Background
The rate at which technology is adopted in organizations varies considerably. Rogers' (2003) diffusion of technological innovation model, which was first used for predicting the introduction rate of hybrid corn seed, provides a helpful paradigm for better understanding the adoption process. Rogers' model, while arguably one of the most popular, is only one of several technology adoption paradigms (Fishbein & Ajzen, 1975; Hamelink, 1984). Applying Rogers' model to academia suggests that a significant proportion of faculty react slowly in embracing technological innovation as highlighted in Figure 1. This format represents a slight variation to the original presentation with the incorporation of a fifth category – Luddites. In a commercial setting the adoption of new technologies throughout the organization, for the most part, centers on economics. In academia this is not the case. There are some faculty members who will never adopt new learning technologies no matter the nature of the incentives and disincentives and, in fact, may erect roadblocks just as their namesakes did some 200 years ago. This reality is the primary reason for adding the Luddite category. These same diffused patterns in faculty adoption behavior were also observed in a study on assessing the pedagogical and technological perceptions of management faculty (Mehra & Mital, 2007). Rogers' model is based on the Empirical Rule gleaned from statistics. The Empirical Rule states that approximately 68% of the population resides within plus or minus one standard deviation from the mean while 95.5% are contained within plus or minus two standard deviations. In the case of Figure 1 less than 16% of the faculty can be regarded as innovators or enthusiasts. Clearly, this percentage does not represent a critical mass. According to Rogers, the majority, which constitutes a little over two thirds of the population, will begin to embrace the technology only after some period of consideration. Laggards will adopt only if they are certain that it will not fail and that there will be no negative consequences to themselves (e.g., negative student evaluations).
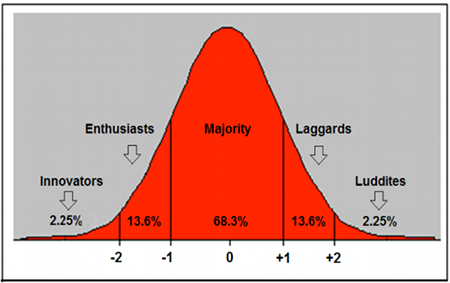
Figure 1. Faculty adoption of technology model
Some basic characteristics of early adopters (innovators and enthusiasts) of learning technology include risk takers, revolutionaries, and horizontal communicators. In contrast, late adopters (majority and laggards) tend to be risk avoiders, evolutionaries, and vertical communicators. Developing an effective diffusion plan based on the experiences of the early adopters can greatly enhance the likelihood that a technology will be successfully integrated into the curriculum by groups beyond the innovators and early adopters (Sahin & Thompson, 2006). The ongoing reporting of success stories will further enhance the opportunities to engage and energize the majority. The perceived usefulness, ease of use, and risk minimization are three factors that are essential for obtaining a buy in by the majority.
The technology acceptance model (TAM) is another adoption construct that has been used to predict faculty acceptance and use of new technology (Davis, 1989). Perceived usefulness and perceived ease-of-use are two key factors associated with this model. The findings from a study, which focused on online education, revealed that enjoyment, perceived usefulness, ease of use, and previous e-tool use predicted students' intentions to use e-tools (Martínez-Torres et al., 2008). In a related study, ease of use and perceived usefulness accounted for 80% of the variance in faculty intent to teach online courses (Kim, 2008). The TAM methodology has also been used to identify faculty members that are oriented toward embracing web-based learning technologies including online education (Stewart, Bachman, & Johnson, 2010). The data from this study indicated that while faculty acceptance is related to behavioral intentions to use new learning technologies, it is not related to faculty perceptions regarding the merits of new learning technologies. Another study found that perceived usefulness was a strong indicator of faculty acceptance, while perceived ease of use offered little additional predictive power (Gibson, Harris, & Colaric, 2008).
Innovators and early adopters tend to embrace new learning modalities based on their own initiative and technical background without the need for significant institutional support. The emergence of a new learning system may provide sufficient motivation for these two groups to embrace the technology. Both cohorts tend to have an experimentation mindset, which often disposes them toward adoption and the associated problem solving (Zayim, Yildirim, & Saka, 2006). However, the chances of inducing widespread adoption of learning systems and technology by the pragmatic majority is another matter. The opportunities will significantly increase if the majority are recognized as a distinct group within the community and made a part of the planning and policy making process. Furthermore, members of the majority tend to have limited interest in the technology and are often oriented toward problems related to their disciplines as opposed to technical innovation. Therefore, an innovation must be demonstrated as both an effective and easily applied solution to a focused need. To overcome these potential barriers the adoption process should be related to their perceived needs and supported by a well-developed and efficient IT staff (Ali, 2003). The motivation for the majority to adopt new learning technologies may not be the same across disciplines. Innovation by laggards is more likely to occur through ongoing majority involvement and continued administrative support. In this way, such training and support will be more transferable to the laggards. The Luddite segment, as the name implies, can be the source of potentially significant disruptions in the overall adoption process. The above discussion is supported by some recent findings which suggest that motivational factors like self-efficacy and philosophy are strongly correlated to adoption practices while teaching experience and peer-pressure are not correlated (Zhen, Garthwait, & Pratt, 2008).
The following are some guiding principles that can help in engaging the majority (Sherry & Gibson, 2002; Starke-Meyerring & Wilson, 2008):
- First-time success. Learning innovations are most likely to be accepted and used by the majority of the faculty if success is experienced early on. Early success also extends the peer network, both within and outside the institution, thereby magnifying the impact on adoption and diffusion.
- Ongoing peer support. Complementing the experience of initial success, there should be ample "handholding" along the way as other applications are introduced. Live peer support not only serves as assistance and encouragement; it contributes to the person-to-person communication that promotes diffusion throughout an educational community.
- Real task activities. The majority are pragmatists who see technology in terms of real problems. Initiatives designed to introduce and use learning technology should address real task activities and requirements. Using Internet access to information and resources, both intra- and inter-institutional, can address many faculty and administrative issues and requirements.
- Ownership and identity. Encouraging and enabling the faculty to "create an active presence" on the Web is essential for adopting new learning technologies. Participating in webinars, creating a personal home page, and publishing electronic papers all contribute to the electronic world-community and help ease "cultural assimilation."
As can be seen, the need for institutional support and commitment to engage the majority is essential. Resources in the form of funds and release time are but two vehicles for motivating the majority to adopt. This top down approach is in sharp contrast to the bottom up approach associated with innovators and early adopters. In either case, however, a system structure must be in place that provides a seamless interface between the adopter and the student body. Any significant failures on the part of the support system will, in general terms, doom any administrative adoption initiative. Technology training opportunities for the majority are also crucial. The results of a recent study showed significant correlations between technology literacy and pedagogical practice integration (Georgina & Olson, 2008). The data also revealed that faculty technology training may be maximized for the integration of pedagogy by using small group faculty forums with a trainer. Technology based adoption trends are also impacted by the product distribution offer by the institution. Similar adoption patterns are not limited to the West. A recent study found that the majority of faculty members from China Agricultural University (CAU) were in the early stages of the innovation-decision process. Only one third of CAU faculty members were in the decision, implementation, or confirmation stage (Li & Lindner, 2007). Even today many business schools are still "full-service" providers offering general educational products (e.g., M.B.A.). With the ongoing unbundling of the business school industry, certain schools may become increasingly specialized (e.g., M.S. in Finance) and faculty could conceivably form faculty groups that serve multiple universities (Friga, Bettis, & Sullivan, 2003). The following two sections provide an overview on the two data mining techniques (neural nets and CART) used in this study. A neural net model was constructed for screening the large number of candidate study variables. A CART analysis was then conducted on the reduced variable set.
Neural Nets
Neural networks have been characterized as "computing devices that use design principles similar to the information processing system of the human brain" (Bharath & Drosen, 1994, p. xvii). They use complex network relationships to mimic the connections between sets of data. Among other things, neural nets have the advantage of not requiring prior assumptions about the data or about possible relationships within the data, as is often the case with traditional analysis methods (e.g., regression). In the most common schema, each neuron in one layer is connected to each neuron in the preceding layer as is illustrated in Figure 2. In this example, the prediction of the growing importance of the Web (binary variable) by the faculty in graduate management education is derived as a function of input states and a set of weights. The specific input states in Figure 2 are the following: (1) member of the Applied Behavioral Sciences Department (ABS); (2) faculty perceived importance of the Web in business (IWB); and (3) faculty plans to more fully embrace the Web (FEW). The values for the input states may come from the activation of other neurons or specific environmental factors. The example numerical value inside the node represents the threshold value for firing or activating the neuron. In this case, if the sum of the weights (W1, W2, W3) times the corresponding variable values (ABS, IWB, FEW) exceeds 1.5 (Σ Wi * Xi), then the neuron is "fired," which suggests a certain level of change in the perceived importance of the Web.
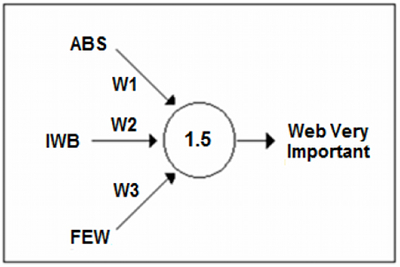
Figure 2. Example predictive neural node
The values for the weights (W1, W2, W3), which can be positive or negative, and the thresholds are determined through an iterative process with the goal of minimizing the aggregate error. The architecture of a neural net consists, at a minimum, of three layers: an input neuron or neuron layer, a "hidden" layer, and an output neuron. There may also be one or more intermediate or "hidden" layers of neurons. Neural net models, like various regression techniques, are impacted by degrees of freedom. In some instances adding more hidden layers can increase the degrees of freedom for a given database. Neural networks have seen increased use in educational studies (González & DesJardins, 2002; Herzog, 2006; Im, Kim, Bae, & Park, 2005). One area of particular interest is classification analysis wherein the target variable is characterized into two or more categories. An example is predicting a prospective student's university performance, where the target variable categories consist of good, average, and poor (Oladokun, Adebanjo, & Charles-Owaba, 2008). The results from this study showed that the model was able to correctly predict the performance of over 70% of the prospective students. The neural net model for the present study consisted of one input, one hidden, and one output layer using Ward's Neuroshell classifier.
Regression Classification
CART is a non-parametric analytical procedure that generates variable based structural trees using one of the following two modeling applications (Steinberg & Golovnya, 2007):
- Classification trees when the target variable is binary;
- Regression trees when the target variable is continuous.
Trees are formed by a collection of rules based on values of certain variables in the modeling process. Rules are selected according to how well splits based on variables' values can differentiate observations pertaining to the dependent variable. Once a rule is selected and splits a node into two, the same logic is applied to each dependent node. The splitting process is terminated when no improvement in the model's performance can be achieved. Each branch of the tree ends in a terminal node. A terminal node occurs when no additional splitting would be useful. The data observations fall into exactly one terminal node. Each terminal node is uniquely defined by a set of rules. Figure 3 illustrates the tree splitting process where again the target variable is the faculty's perceived importance of the Web to graduate management education. The first variable selected is the intensity of the faculty's commitment to embrace the Web (Q3SA – node #1). The binary split leads to nodes #2 and terminal node #4. Notice that in this case 87.5% of those faculty members that believe that the Web is essential to graduate management education were classified correctly based on variable Q3SA.
The classification performance is not as robust when faculty intensity is not strong (i.e., only 62% were classified correctly). This is why node #2 is split using the faculty's perceived importance of the Web in business (Q2SA). This yields terminal node #1 where 81.3% of those who do not believe in the importance of the Web in graduate management education were classified correctly.
Finally, node #3 is split into two terminal nodes (#2 and #3) depending on whether the faculty member was associated with the Applied Behavioral Sciences (ABS) Department. These example results suggest that ABS faculty tend to have a more positive attitude toward the Web than non-ABS members, although the sample size is very small. In some CART applications the number of splits can become quite large, which often requires some pruning. Furthermore, sample size can have a significant impact on the classification process. The CART modeling approach offers a number of advantages in many analytical situations (Lewis, 2000; Su, Chen, Cheng, & Chen, 2010):
- Results are more understandable compared with OLR and neural nets. Tree logic makes it easier to comprehend model outcomes;
- Model is extremely robust to the effect of outliers. The data-splitting nature of decision rules makes it possible to distinguish between datasets with different characteristics and hence to neutralize outliers in separate nodes;
- Relative ease in modeling variable interactions.
CART has seen extensive application in the study of higher education (Weerts & Ronca, 2009; Yu, DiGangi, Jannasch-Pennell, & Kaprolet, 2010). One of CART's advantages is that it can handle missing data, different relationships between variables in different parts of the measurement space, and outliers (Feldman & Gross, 2005). This study was conducted without any pre-analysis that might have narrowed down the field of potential predictors. However, the pre-processing by another classifier often can improve the accuracy of the CART classifier (Abu-Hanna & de Keizer, 2003). This two-step methodology was the approach taken in the current study.
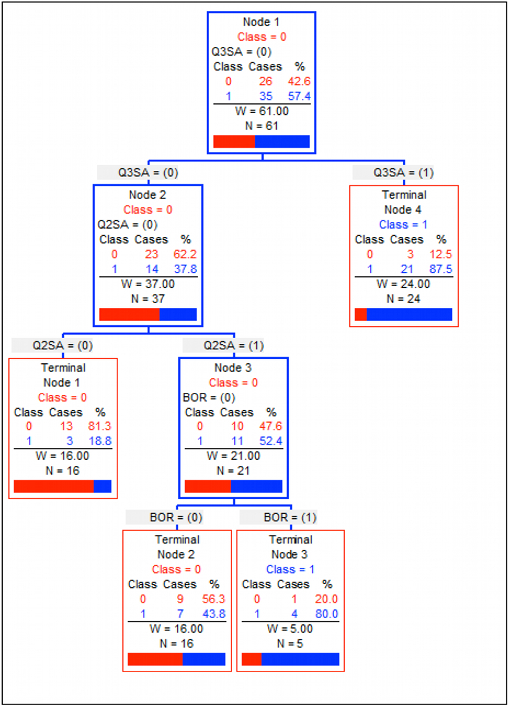
Figure 3. Example CART structure
Database
An Internet-based survey was conducted of faculty in the Graziadio School of Business and Management (GSBM) at Pepperdine University during the Spring of 2011. This survey is part of an ongoing effort to measure GSBM faculty attitudes and trends toward learning technology, innovation, and curriculum design. A multiple-choice format was used for the survey. There were basically two types of questions: the first involving the level of intensity regarding technology adoption, and the second being the estimated timeframe for the adoption of various learning technologies. Both types of questions were measured on a 5-point scale. Data was also collected on the faculty member's department. Presented in the following are descriptive results for selected survey questions. Figure 4 indicates that over 80% of the faculty believes that GSBM should more fully embrace the use of the Internet for educational purposes. This is not to say that 80% of the faculty members surveyed are using the Internet in a significant way.
The GSBM faculty was asked to forecast the timeframe for adopting e-books. The results are shown in Figure 5. Approximately 60% of the faculty plan to be using e-books within the next two years. This corresponds to a position slightly to the right of the midpoint in Rogers' (2003) distribution (see Figure 1). Furthermore, fewer than 15% indicated no plans to adopt e-books which is approximately equal to the last two categories in Rogers' model (laggards and Luddites).
Figure 6 portrays a completely different picture. Here less than 40% of the GSBM faculty plan to use the Internet for broadcasting webinars within the next two years. These results suggest that Rogers' (2003) adoption model for webinars is considerably less mature compared to the e-book model. This should not be too surprising since e-book adoption is significantly easier to implement compared to webinars.
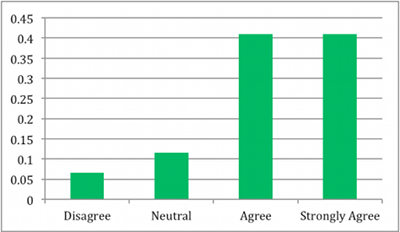
Figure 4. Importance of more fully embracing the Internet (N = 61)
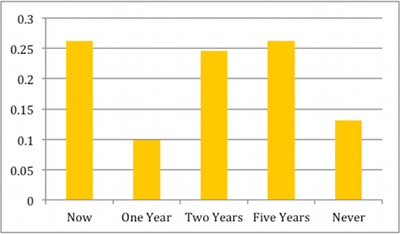
Figure 5. Timeframe to adopt e-books (N = 61)
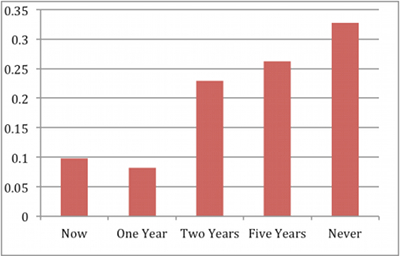
Figure 6. Timeframe to use webinars (N = 61)
As mentioned in the Introduction, sustainability is a growing issue on many university campuses (Clegg, 2008). Many faculty members champion sustainability in two ways: (1) integrating sustainability ideas into the curriculum; and (2) reducing energy consumption associated with the learning process. The latter provides the faculty with the opportunity to contribute directly to sustainability by reducing student commuting and reliance on print-books through the use of Internet-based learning management systems. Recent data suggests that web-based learning oriented programs can reduce both energy consumption and CO2 emissions by approximately 86% compared with full-time campus-based programs through reduced commuting (Roy, Potter, & Yarrow, 2008). Furthermore, transitioning from printed materials to electronic based materials can reduce energy consumption and carbon emissions associated within the print industry by upwards of 90% (Engelhaupt, 2008). E-books, in particular, and digital material, in general, can be easily distributed via the Internet, and the cost of an e-book is approximately 25% that of a comparable printed book (Christopher, 2008). As this evidence suggests, the adoption of new learning technologies can contribute directly to enhancing both energy and environmental sustainability.
Analysis
As previously discussed, the small sample size (N = 61) associated with the survey necessitated a reduction in the original variable set. Accordingly, a two-phase approach was used. First, a neural net model was used to prune the original candidate list down to a more manageable subset. Second, a CART analysis was performed to develop a set of decision rules. This survey design resulted in a total of 18 dummy predictor variables. The target variable was the perceived importance of the Internet to the future of the school (1 = Very important, 0 = Other). Because of the small sample size a holdout group was not used. This approach often results in overly optimistic model performance (Picard & Cook, 1984). The results from the neural nets screening analysis are highlighted in Table 1. For example, the factor "now using Internet simulations" had the highest association with the target variable. The relative influencing factors generated by the neural net analysis were used to select the final set of variables for the CART analysis.
Table 1. Neural net variable rankings
Factor |
|
Now using Internet simulations
|
|
|
|
Using e-books within one year
|
|
Strongly agree business is increasingly using the Internet
|
|
Member Decision Science/Marketing Department
|
|
Member Behavioral Science/Management Department
|
|
Strongly agree faculty needs to fully embrace the Internet
|
|
Agree faculty needs to embrace the Internet
|
|
Providing webinars within two years
|
|
Using Internet simulations within one year
|
|
Member Finance/Accounting Department
|
|
Agree business is increasingly using the Internet
|
|
|
|
Using e-books within two years
|
|
Neutral about faculty embracing the Internet
|
|
Providing webinars within one year
|
|
Using Internet simulations within two years
|
|
Member Economics/Law Department
|
|
Not surprisingly, those faculty members that are either currently using Internet-based learning systems, or plan to in the near future, recognize the growing importance of the Web in management education. Also, two of the faculty departments surveyed showed a much stronger commitment to Internet adoption compared to the other departments. The top seven ranked variables from Table 1, which were gleaned from the neural net analysis, served as the basis for developing the screening rule set using the CART methodology. The optimized classification rules were based on the following factors: (1) Decision Sciences and Marketing Department; (2) Applied Behavioral Sciences and Organization Theory Department; (3) strongly believe that business is increasingly using the Internet; and (4) strongly agree that faculty need to more fully embrace the Internet. The classification analysis results are shown in Table 2. The CART decision rules correctly identified 85% of the Internet supporters (sensitivity) and 75% of the non-Internet supporters (specificity). The positive predictive value (PPV) and negative predictive value (NPV) were 0.80 and 0.81, respectively. For example, the reported PPV indicates that 80% of the faculty that were classified as Internet supporters were classified correctly. The overall performance of the classification process suggests that the CART model obtained reasonably good separation between supporters and non-supporters.
Table 2. Neural net variable rankings
Note. PPV = Positive predictive value (ratio of the number of supporters of Internet-based learning classified correctly divided by the total number of those classified as supporters); NPV = Negative predictive value (ratio of the number of non-supporters of Internet-based learning classified correctly divided by the total number of those classified as non-supporters).
Implementation Strategy
Implementing new learning technologies on a wholesale basis in a graduate management program is not a simple task. A number of specific steps must be taken to ensure successful deployment. These steps include:
- Thinking long term. Link the adoption of new learning technologies to the mission statement of the institution. Look ahead five years in terms of development direction and tempo.
- Developing content. Formulate a content acquisition plan based on consensus. Typically, the development and acquisition of web-based content can account for upwards of 50% of the overall budget.
- Comparison shopping. Carefully evaluate the portal providers. Any disruption in providing 24/7 learning will court disaster.
- Implementing a phased approach. Consider prototyping new learning technologies in a specific program (e.g., Executive Management) to gain experience and confidence.
To reap the full potential of the new learning technologies, the course design must involve more than simply "attaching" a series of websites to the standard classroom format. Generally, the course structure must be redesigned to provide a seamless transition between face-to-face learning and asynchronous learning (Conway, Easton, & Schmidt, 2005). Student "buy in" represents another key factor to the successful implementation of the LMS paradigm. Students must be convinced that the convenience and richness of Internet resources offsets the perceived notion that they can only learn in a classroom. Several ways to accomplish this is to have students serve as course co-producers and organizing students into self-sustaining Internet based support teams. The latter approach helps reduce the chances that no one is left behind. Furthermore, a gradual move from a frontal to an increased self-learning methodology may induce more overall success (Timmor & Rymon, 2008). Consequently, improved learning outcomes may occur if students are exposed to more than one learning format while transitioning to the high-tech stratagem.
Arguably, the single most important element of the implementation stratagem is faculty "buy in" and orientation. As previously outlined some faculty are reluctant to embrace new instructional modalities. This is often due to technology phobia, a lack of motivation, and no additional compensation. While some institutions do provide some incremental resources for course development, the general perspective is that it is up to faculty to make the transition as part of the modernization of academia. One approach to help ameliorate some faculty concerns is through the use of web-based training modules. These systems not only introduce the faculty to the "power" of the Internet but also show them how to design and implement courses based on the latest learning technologies. As mentioned earlier, small group faculty forums using an e-moderator represent another implementation approach.
The deployment of new learning technologies on a wide-scale basis will result in significant direct and indirect costs for schools of business. Often, these costs are both difficult to estimate and measure. Institutions need to develop a cost structure that recognizes both the local context and cultural conditions, as these will influence outcomes (Bradley, Seidman, & Painchaud, 2011). The total cost associated with infrastructure development is often underestimated. Furthermore, faculty and staff development costs are not fully understood, particularly the extra time required for faculty to learn how to use the technology and to develop appropriate content (Normand, Littlejohn, & Falconer, 2008). One cost structure paradigm that addresses these challenges is called INSIGHT (Nicol & Coen, 2003). The structure consists of three basic cost considerations: 1) infrastructure, 2) value added, and 3) academic support. This system allows program designers to evaluate competitive priorities within the context of the overall institution (e.g., student expectations versus faculty expectations). In addition to cost considerations, some additional administrative challenges in implementing new learning technologies include the following:
- Training faculty for successful system deployment and usage;
- Providing high quality and consistent system access;
- Setting specific performance goals and metrics;
- Preparing students for entry and ongoing use;
- Sustaining system operation and flexibility;
- Establishing the overall culture.
Developing the internal capability to deploy new learning technologies (e.g., LMS) is complex and expensive. Furthermore, an internalized approach may not take advantage of ongoing developments in delivery technology, for example, search engine technology. One implementation strategy that helps overcome these issues consists of developing institutional partners with both content and application service providers. This approach draws on the basic ideas behind supply chain management and is consistent with the increased use of suppliers in large volume operations, such as those found in most business programs. Measuring ongoing effectiveness and performance is a key to the successful sustainability of new learning technologies (Weippl, 2007).
Conclusion
This paper contributes to the body of knowledge in the field of online learning in three ways: (1) highlights the results of a survey on faculty perceptions regarding the role of new learning technologies in graduate management education; (2) introduces a two-stage variable classification methodology based on neural nets and CART; and (3) outlines an approach for helping bridge the gap between faculty adoption of new learning technologies and the demand for change in delivering world class business education. While the sample size is relatively small, the survey results show a significant divide between faculty innovators and those that are resistant to change. These results are consistent with those reported in the literature. The survey data also revealed that technology adoption rates vary across disciplines. Many business school faculty recognize that massive forces are in play that are shaking the very foundations of traditional graduate management education. Some of the factors impacting the classical business school model include globalization, technology, sustainability, and student demographics. Arguably the single most important step in developing a viable technology implementation strategy is to link the role of learning technologies to the mission and vision statements of the institution. Furthermore, even though the technology is moving rapidly, taking a long-term view is essential. One area for future research is to identify incentives for the faculty to embrace the new learning technologies. Finding the right set of incentives is crucial at a time of great stress on graduate management education. Faculty training represents another important aspect of enhancing the technology adoption process. Research into the best methods for providing enhanced training is certainly needed. Collaboration networks provide a vehicle for helping faculty transition to more fully embracing new learning technologies. The goal of faculty collaboration networks is to provide a platform where the faculty can share and exchange ideas to drive innovation regarding student learning. The small sample size and narrow discipline focus limits the ability to generalize the results, however, the methodological approach and implementation strategies hopefully contribute to the conversation regarding faculty adoption of new learning technologies.
References
Abu-Hanna, A., & de Keizer, N. (2003). Integrating classification trees with local logistic regression in intensive care prognosis. Artificial Intelligence in Medicine, 29(1), 5-23. doi:10.1016/S0933-3657(03)00047-2
Ali, A. (2003). Faculty adoption of technology: Training comes first. Educational Technology, 43(2), 51-53.
Bharath, R., & Drosen, J. (1994). Neural network computing. New York, NY: McGraw-Hill.
Bradley, M. J., Seidman, R. H., & Painchaud, S. R. (2011). Saving higher education: The integrated, competency-based three-year bachelor's degree program. San Francisco, CA: Jossey-Bass.
Christopher, L. C. (2008). Academic publishing: Digital alternatives to expensive print books. The Seybold Report, 8(19), 11-14.
Clegg, P. (2008). Creativity and critical thinking in the globalised university. Innovations in Education and Teaching International, 45(3), 219-226. doi:10.1080/14703290802175982
Conway, R. N., Easton, S. S., & Schmidt, W. V. (2005). Strategies for enhancing student interaction and immediacy in online courses. Business Communication Quarterly, 68(1), 23-35. doi:10.1177/1080569904273300
Daniel, J., Kanwar, A., & Uvalic-Trumbic, S. (2006). A tectonic shift in global higher education. Change: The Magazine of Higher Learning, 38(4), 16-23. doi:10.3200/CHNG.38.4.16-23
Davis, F. D. (1989). Perceived usefulness, perceived ease of use, and user acceptance of information technology. MIS Quarterly, 13(3), 319-340. doi:10.2307/249008
Dykman, C. A., & Davis, C. K. (2008). Part one – The shift toward online education. Journal of Information Systems Education, 19(1), 11-22.
Engelhaupt, E. (2008). Would you like that book in paper or plastic? Environmental Science & Technology, 42(12), 4242-4245. doi:10.1021/es087144e
Feldman, D., & Gross, S. (2005). Mortgage default: Classification trees analysis. The Journal of Real Estate Finance and Economics, 30(4), 369-396. doi:10.1007/s11146-005-7013-7
Fishbein, M., & Ajzen, I. (1975). Belief, attitude, intention, and behavior: An introduction to theory and research. Reading, MA: Addison-Wesley.
Friga, P. N., Bettis, R. A., & Sullivan, R. S. (2003). Changes in graduate management education and new business school strategies for the 21st century. Academy of Management Learning & Education, 2(3), 233-249. doi:10.5465/AMLE.2003.10932123
Georgina, D. A., & Olson, M. R. (2008). Integration of technology in higher education: A review of faculty self-perceptions. The Internet and Higher Education, 11(1), 1-8. doi:10.1016/j.iheduc.2007.11.002
Gibson, S. G., Harris, M. L., & Colaric, S. M. (2008). Technology acceptance in an academic context: Faculty acceptance of online education. Journal of Education for Business, 83(6), 355-359. doi:10.3200/JOEB.83.6.355-359
González, J. M .B., & DesJardins, S. L. (2002). Artificial neural networks: A new approach to predicting application behavior. Research in Higher Education, 43(2), 235-258. doi:10.1023/A:1014423925000
Hamelink, J. M. (1984). Integration of technology. Performance & Instruction Journal, 23(2), 26-27. doi:10.1002/pfi.4150230212
Hawawini, G. (2005). The future of business schools. Journal of Management Development, 24(9), 770-782. doi:10.1108/02621710510621286
Herzog, S. (2006). Estimating student retention and degree-completion time: Decision trees and neural networks vis-à-vis regression. New Directions for Institutional Research, 131, 17-33. doi:10.1002/ir.185
Im, K. H., Kim, T. H., Bae, S., & Park, S. C. (2005). Conceptual modeling with neural network for giftedness identification and education. In L. Wang, K. Chen, & Y. S. Ong (Eds.), Advances in Natural Computation: Proceedings of the First International Conference on Natural Computation (ICNC 2005), Part II. Vol. 3611 Lecture Notes in Computer Science (pp. 530-538). Heidelberg, Germany: Springer. doi:10.1007/11539117_76
Kim, M.-R. (2008). Factors influencing the acceptance of e-learning courses for mainstream faculty in higher institutions. International Journal of Instructional Technology and Distance Learning, 5(2), 29-44. Retrieved from http://www.itdl.org/Journal/Feb_08/article03.htm
Lenzner, R., & Johnson, S. S. (1997). Seeing things as they really are. Forbes, 159(5), 122-128. Retrieved from http://www.forbes.com/forbes/1997/0310/5905122a.html
Lewis, R. J. (2000, May). An introduction to classification and regression tree (CART) analysis. Paper presented at the 2000 Annual Meeting of the Society for Academic Emergency Medicine, San Francisco, CA. Retrieved January 17, 2009, from http://www.saem.org/download/lewis1.pdf (archived at http://web.archive.org/web/*/http://www.saem.org/download/lewis1.pdf)
Li, Y., & Lindner, J. R. (2007). Faculty adoption behavior about web-based distance education: A case study from China Agricultural University. British Journal of Educational Technology, 38(1), 83-94. doi:10.1111/j.1467-8535.2006.00594.x
Martínez-Torres, M. R., Toral Marín, S. L., Barrero García, F., Gallardo Vázquez, S., Arias Oliva, M., & Torres, T. (2008). A technological acceptance of e-learning tools used in practical and laboratory teaching, according to the European higher education area. Behaviour & Information Technology, 27(6), 495-505. doi:10.1080/01449290600958965
Mehra, P., & Mital, M. (2007). Integrating technology into the teaching–learning transaction: Pedagogical and technological perceptions of management faculty. International Journal of Education and Development using Information and Communication Technology, 3(1), 105-115. Retrieved from http://ijedict.dec.uwi.edu/viewarticle.php?id=265&layout=html
Nicol, D., & Coen, M. (2003). A model for evaluating the institutional costs and benefits of ICT initiatives in teaching and learning in higher education. ALT-J, Research in Learning Technology, 11(2), 46-60. doi:10.1080/0968776030110205
Nor Aziah Alias, & Ahmad Marzuki Zainuddin (2005). Innovation for better teaching and learning: Adopting the learning management system. Malaysian Online Journal of Instructional Technology, 2(2), 27-40. Retrieved April 19, 2009, from http://pppjj.usm.my/mojit/articles/pdf/August05/03-Article-037-NorAziah-UiTM%20version%202%5B1%5D.pdf (archived at http://web.archive.org/web/20090419041330/http://pppjj.usm.my/mojit/articles/pdf/August05/03-Article-037-NorAziah-UiTM%20version%202%5B1%5D.pdf)
Normand, C., Littlejohn, A., & Falconer, I. (2008). A model for effective implementation of flexible programme delivery. Innovations in Education and Teaching International, 45(1), 25-36. doi:10.1080/14703290701757351
Oladokun, V., Adebanjo, A., & Charles-Owaba, C. (2008). Predicting students' academic performance using artificial neural network: A case study of an engineering course. The Pacific Journal of Science and Technology, 9(1), 72-79. Retrieved from http://www.akamaiuniversity.us/PJST9_1_72.pdf
Picard, R. R., & Cook, R. D. (1984). Cross-validation of regression models. Journal of the American Statistical Association, 79(387), 575-583. doi:10.1080/01621459.1984.10478083
Rogers, E. M. (2003). Diffusion of innovations (5th ed.). New York, NY: Free Press.
Roy, R., Potter, S., & Yarrow, K. (2008). Designing low carbon higher education systems: Environmental impacts of campus and distance learning systems. International Journal of Sustainability in Higher Education, 9(2), 116-130. doi:10.1108/14676370810856279
Sahin, I., & Thompson, A. (2006). Using Rogers' theory to interpret instructional computer use by COE faculty. Journal of Research on Technology in Education, 39(1), 81-104. Available from ERIC database. (EJ768868)
Sherry, L., & Gibson, D. (2002). The path to teacher leadership in educational technology. Contemporary Issues in Technology and Teacher Education, 2(2), 178-203. Retrieved from http://www.citejournal.org/vol2/iss2/general/article2.cfm
Starke-Meyerring, D., & Wilson, M. (Eds.). (2008). Designing globally networked learning environments: Visionary partnerships, policies, and pedagogies. Rotterdam, The Netherlands: Sense.
Steinberg, D., & Golovnya, M. (2007). CART 6.0 User's Guide. San Diego, CA: Salford Systems. Retrieved from http://dist.salford-systems.com/CART6/CART6.pdf
Stewart, C., Bachman, C., & Johnson, R. (2010). Predictors of faculty acceptance of online education. MERLOT Journal of Online Learning and Teaching, 6(3), 597-616. Retrieved from https://jolt.merlot.org/vol6no3/stewartc_0910.htm
Su, C.-H., Chen, T.-L., Cheng, C.-H., & Chen, Y.-C. (2010). Forecasting the stock market with linguistic rules generated from the minimize entropy principle and the cumulative probability distribution approaches. Entropy, 12(12), 2397-2417. doi:10.3390/e12122397
Timmor, Y., & Rymon, T. (2008). Don't imitate, innovate: The case of the hybrid education format in a management course. International Journal of Information and Operations Management Education, 2(4), 341-358. doi:10.1504/IJIOME.2008.023438
Weerts, D. J., & Ronca, J. M. (2009). Using classification trees to predict alumni giving for higher education. Education Economics, 17(1), 95-122. doi:10.1080/09645290801976985
Weippl, E. R. (2007). Dependability in e-assessment. International Journal on E-Learning, 6(2), 293-302. Available from EdITLib Digital Library. (19793)
Yu, C. H., DiGangi, S., Jannasch-Pennell, A., & Kaprolet, C. (2010). A data mining approach for identifying predictors of student retention from sophomore to junior year. Journal of Data Science, 8(2), 307-325. Retrieved from http://www.jdsruc.org/upload/JDS-574(2010-4-1142427).pdf
Zayim, N., Yildirim, S., & Saka, O. (2006). Technology adoption of medical faculty in teaching: Differentiating factors in adopter categories. Educational Technology & Society, 9(2), 213-222. Retrieved from http://www.ifets.info/journals/9_2/17.pdf
Zhen, Y., Garthwait, A., & Pratt, P. (2008). Factors affecting faculty members' decision to teach or not to teach online in higher education. Online Journal of Distance Learning Administration, 11(3). Retrieved from http://www.westga.edu/~distance/ojdla/fall113/zhen113.html