Is E-Learning for Everyone?
An Internal-External Framework
of E-Learning Initiatives
|
Pingying Zhang
Department of Management
Coggin College of Business
University of North Florida
Jacksonville FL 32224 USA
Pingying.zhang@unf.edu
Lakshmi Goel
Department of Management
Coggin College of Business
University of North Florida
Jacksonville FL 32224 USA
l.goel@unf.edu
Abstract
Higher educational institutions are increasingly using electronic platforms as teaching environments. There is a gap in research that investigates individual-level factors that contribute to, and hamper, e-learning initiatives. A framework called the Internal-External Model is proposed in an effort to explain individual e-learning success. This framework is derived from the strategic management technique of identifying strengths, weaknesses, opportunities and threats (SWOT). The framework is tested through data collected at a large southern university in the US, and the findings reported. Finally, implications for research and practice are presented. This paper makes two contributions. First, prior research on e-learning success is synthesized by identifying relevant empirical literature. In doing so, inconsistencies in empirical findings of prior research in e-learning success are highlighted. Second, the study offers a theoretical framework that may account for these inconsistencies by specifying the simultaneous role played by both internal and external factors. Results support a model where a favorable external environment for e-learning together with strong internal drives towards e-learning would in general lead to higher e-learning outcomes.
Keywords: E-learning outcomes, SWOT analysis, E-learning intentions, E-learning satisfaction.
|
Introduction
There is an increased interest in integrating electronic platforms as teaching environments. Various forms of e-learning, from online content distribution and testing to synchronous instructor-led sessions, are being adopted for training and education. The acceleration towards developing and leveraging e-learning strategies is fueled by three drivers that are characteristic of today's business environment.
The first is that of globalization. As geographic and temporal barriers become less salient, most organizations are moving to a global business landscape. A company most likely has some global footprint, if not in the form of physical presence, then through its international suppliers, consumers, offshore vendors, or business partners. 24/7 global reach, and communication technologies help organizations conduct business anytime, anywhere. This phenomenon has correspondingly increased demand for distance education and virtual courses in higher educational institutions such as business schools (Harper, Chen, & Yen, 2004; Mupinga, 2005), such that geographic location and temporal boundaries are not restrictive in obtaining education.
The second driver is the technological advancement of this age. Information technologies continue to increase in power and decrease in cost. The past five years have seen an exponential growth in the processing capacity of mobile devices such as laptops and handhelds. Correspondingly, information representation is changing from basic text to richer, more intuitive forms that involve sound, motion, and touch. Besides improvement to hardware and software, support for connectivity to the web has increased. There are increasing number of ‘hotspots’ and higher signal strengths available at public places. The Internet is even more pervasive than it was predicted to be, and this pervasiveness continues to grow.
The last driver is demographic forces that come from the nature of the students, and the workforce today. The age at which individuals are exposed to technologies such as the Internet and social networking keeps decreasing. Disney’s Toontown, Whyville, Barbie Girls and Club Penguin are only some examples of the many virtual worlds that are available for pre-teens. Whyville (www.whyville.net) is: “…an educational virtual world for children and teens, ages 10-16. …this world's motto is "learning by doing." Whyville actively engages its visitors and encourages them to participate in fun, educational events that give kids "hands-on" experience with science projects (in a virtual way, of course).”
There are specific virtual worlds geared for children of different ages. By the time they are in high school, most individuals in the US are very comfortable with navigating the web using rich interfaces to communicate and conduct business. As suggested by Dede (2004), technological complementarities that will shape how people learn include familiarity with the “world through the desktop” notion where individuals have access to people and information from around the world through the Internet; familiarity with multiuser virtual environments (MUVEs) due to their exposure to gaming and social interaction through these interfaces; and familiarity with ubiquitous computing such that individuals increasingly use mobile devices (such as PDAs and media players like iPod) that allow infusion of virtual resources in day-to-day real life.
As the generation familiar with the use of technologies to gain information gets assimilated into the future workforce, technologies for learning and communication will reflect their needs. In keeping with the demand generated from the forces above, many higher education institutions have adopted e-learning in some form as part of their curriculum offering. Courses branded as online, distance, hybrid, or virtual, have some component which leverages electronic platforms for education. While the move towards e-learning is seen as inexorable, it is important to look at possible factors that determine the success of e-learning initiatives at an individual level. In conducting a literature review, we find that the phenomenon of e-learning has received considerable attention. However, the few studies on predicting individuals' success in e-learning environments have achieved inconclusive results (for example Rodriguez, Ooms, & Montanez, 2008). In part, the inconclusiveness of the results can be attributed to the failure of these studies to consider a comprehensive list of factors that may come into play when determining e-learning success.
We rely on literature in strategy, specifically the strengths, weaknesses, opportunities, threats (SWOT) analysis, to guide the development of a framework that explains e-learning success. While the use of the SWOT framework in explaining e-learning is not new, prior research falls short in describing a model specific to the e-learning context, and providing empirical support for such a model. Our aim in this paper is to address these gaps in research. The following sections 1) describe the Internal-External Model of e-learning and formulate propositions relating to the factors identified in the literature review 2) present a proposed methodology of testing the propositions, and 3) discuss specific implications of the research. Results from this research program have the potential to guide the development and conduction of e-learning initiatives in order to maximize the chances of success at an individual level.
Literature Review
E-Learning Success
E-learning success can be defined through multiple perspectives. One way to define success is through outcome factors such as enhanced learning, time savings, and academic success (Davies & Graff, 2005; Govindasamy, 2002; Garrison & Anderson, 2003). Another perspective on e-learning success considers system delivery factors such as the degree of use of the e-learning system and its adoption (Holsapple & Lee-Post, 2006). User satisfaction has proved to be a reliable proxy for the success of an IT-based initiative (Bailey & Pearson, 1983). Since e-learning initiatives are strongly tied to IT, students’ satisfaction is an important outcome of e-learning (Goda, 2008; Siritongthawon & Krairit, 2004) . Satisfaction itself can be multi-faceted and include elements such as perceptions of student achievement, attitudes and retention (Bernard et al., 2004), online interactions, thinking skills, information-processing skills (Hew & Cheung, 2003), and perceived quality of online courses (Rodriguez et al., 2008). We continue this line of research, focusing on a multi-dimensional view of students’ satisfaction of e-learning, and try to understand potential factors that impact students’ satisfaction, future intentions, and perceptions of efficiency and effectiveness. Prior research that predicts e-learning success outcomes has yielded inconclusive results. For example, Rodriguez et al. (2008) failed to find a significant link between comfort with technology and number of online courses taken, while Gunawardena and Duphorne (2000) found that a learner well equipped with online skills is significantly associated with the satisfaction of online learning. Such inconclusive results present a gap in research which fully explains e-learning outcomes. In order to resolve these inconsistencies, we attempt to include multiple factors within a single framework to predict e-learning success. The SWOT framework is used as a template to derive an Internal-External model for e-learning success.
SWOT Framework
The SWOT framework is a strategic analysis tool used to identify and evaluate the strengths, weaknesses, opportunities and threats involved in a project or business venture. Since e-learning initiatives in universities can be considered projects undertaken by these institutions, the SWOT tool may well apply to this context. The technique is credited to Albert Humphrey in the 1960s, and has been successfully applied to various contexts since. A central idea in SWOT analysis is identifying a primary objective, or desired end state of the project. For most institutions that undertake e-learning initiatives, the desired outcome would be successful adoption of e-learning by students. We hence focus on e-learning success as our primary outcome. Strengths and weaknesses are identified for the persons or practices within the organization that help or impede the achievement of the objective. Opportunities and threats relate to external environmental conditions that help or impede the achievement of the objective. Hence, strengths and weaknesses are considered factors internal to students participating e-learning; while opportunities and threats are factors external to the students. This framework is used as a guide to identify internal and external factors of interest in our context, i.e. e-learning initiatives.
In earlier studies, SWOT analysis has been used to evaluate software tools for e-learning systems (Bilalis et al., 2002), distance learning opportunities (Tait & Mills, 1999), broad university strategies (Cardosa, Trigueriros & Narciso , 2005), student perceptions (Jackson & Helms, 2008), and digital library implementations (Wang, 2003). Our study differs from prior literature in our object of analysis. We focus on e-learning initiatives, with the goal of their success. To our knowledge, only one other study attempts to do the same. Engelbrecht (2003) uses the SWOT analysis to assess e-learning success outcomes. Our study also differs from prior literature in that we use the SWOT framework only as a guiding lens to arrive at a model, called the Internal-External Model, that is specific to the e-learning context, and can help predict success. Prior literature also falls short in providing empirical support for factors identified. The SWOT can be typically represented as a two-by-two matrix, such that combinations of strengths, weaknesses, opportunities and threats at different levels create different conditions that influence outcomes. We use this representation to frame the Internal-External Model. The Internal-External Model is tested empirically, and thus contributes to literature and practice in this stream of research.
Internal-External Model
We develop a general Internal-External Model to describe potential factors that impact outcomes of e-learning in higher education institutions. As discussed earlier, e-learning success can be defined through multiple perspectives, such as outcome factors and student satisfaction. We continue this line of research, focusing on a multi-dimensional view of students’ satisfaction of e-learning, and try to understand potential factors that impact students’ satisfaction with the content and the institution, future intentions of returning to an e-learning course, and perceptions of efficiency and effectiveness.
These factors are included in a broad framework, which we call the Internal-External Model. The label is not merely renaming an existing model. We specifically choose the title because we do not consider the SWOT tool as-is, but adapt it to evaluate factors that are individual-dependent (internal) are those that individual’s do not have control over (external). Hence while our model is derived from a SWOT perspective, we do not adhere to identifying strengths, weaknesses, opportunities, and threats. Rather, we adapt the tool to identify the combined influence of factors internal to, and external to, and individual as they relate to e-learning outcomes. This is shown in the figure below:
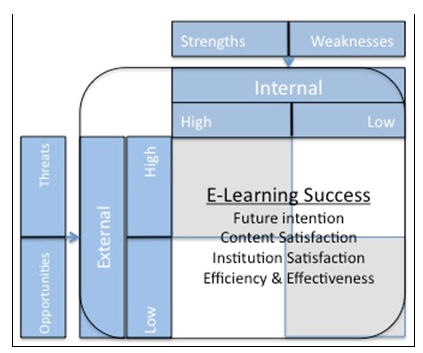
Figure 1 . Internal-External Model
The key of the Internal-External Model is that both internal and external aspects affect perceptions of e-learning, and hence e-learning outcomes, in the same way that internal and external forces influence organizational performance simultaneously. In the model, internal factors include an individual’s online skill, general attitude towards IT, personal innovativeness with information technology, and prior experience with online education. It is reasonable to assume that a positive link exists between internal factors and individuals’ satisfaction with e-learning. For instance, if poorly equipped with basic online skills, individuals would feel less comfortable with technology involved in e-learning, which could further hinder their learning quality, and as a result, decrease their inclination to take e-learning courses.
Prior research that has studied the relationship between some of the internal factors and e-learning satisfaction, however, has encountered empirical inconclusiveness. For example, Rodriguez et al. (2008) failed to find a significant link between comfort with technology and number of online courses taken, while Gunawardena and Duphorne (2000) found that a learner well equipped with online skills is significantly associated with the satisfaction of online learning. Such inconclusive results present a gap in research which fully explains e-learning outcomes. In this article, we aim to address this gap, by proposing that when both favorable external and internal factors present, could e-learning satisfaction be improved. The internal factors examined in this study are online skills, attitudes towards technology, personal innovativeness with technology, and online experience.
Online Skills
Individuals’ ability to use e-learning systems includes computer skills and comfort with the online mode of learning content delivery (Eastmond, 1994). This factor has received a strong empirical support in the context of the academic computer conference system (Harasim et al., 1995; Gunawardena & Duphorne, 2000), indicating the central role played by online skills and computer skills in the perceived satisfaction of e-learning. Other studies focused on the drivers of successful e-learning generated similar results. For example, one study found that students’ computer anxiety is one of the critical factors that reduced their satisfaction (Sun et al., 2008). Another study of e-learning set in Japan concluded that students’ online skill predicted perceived satisfaction with e-learning (Bray, Aoki, & Dlugosh, 2008).
Attitude Toward Technology
Capturing online skills is not only a static report of a learner’s current skill set, but also implies a continuously evolving process. This change perspective explains that students with poor online skills could learn to master them, and over time, could become satisfied with e-learning. The change corresponds with process thinking, where an evaluation that involves process thinking could be useful in understanding the success of e-learning (Gunawardena, Carabaial, & Lowe, 2001). We continue this line of thinking, and analyze factors beyond online skills. For example, factors such as a general positive attitude towards IT, that could motivate individuals to improve online skills, are thus merit further investigation. Attitudes shape our actions. Individuals with a general positive attitude towards technology are more likely motivated to learn skills that e-learning requires and that they do not have. A significant relationship has been found between attitudes to learn technology and comfort with technology, albeit with a small impact factor (Rodriguez et. al, 2008).
Personal Innovativeness in Information Technology
An openness to experience new technologies in general may also motivate students to develop online skills that they do not possess. Empirical studies concerning this link, however, are still limited. Personal innovativeness in information technology (PIIT), defined as “the willingness of an individual to try out any new information technology” (Agarwal & Prasad, 1998, p. 206), also has an explicit relationship with individual perceptions of new technology (Agarwal & Prasad, 1998), which could impact students’ online skills learning.
Online Experience
Prior experience with e-learning could also enhance the comfort with technology involved in e-learning, thus satisfaction of e-learning (Eastmond, 1994). For example, Gunawardena and Duphorne (2000) found that prior experience acquired from training improves e-learners’ readiness, which further enhances e-learners’ satisfaction. However, in another study, Rodriguez et al. (2008) failed to find the significant correlation between online experience and the level of comfort with technology.
Integrating Internal and External Forces
As noted earlier, the inconsistent results of e-learning satisfaction could be due to the lack of an integrated model, since factors seldom affect individual outcomes in an isolated manner. Using the Internal-External model, we propose that only when external and internal factors are considered simultaneously, could we meaningfully evaluate the results of e-learning satisfaction. Two external forces are analyzed: ease of use and institutional support. We posit that each external force coupled with each internal factor influences e-learning satisfaction, thus generating either hypotheses that are discussed below.
Ease of Use
A factor inherent to the technology for e-learning is the ease of use of technological medium (Davis, 1989). The technology acceptance model (TAM) posits that individuals are more likely to adopt a technology if it is easy to use, and useful (Davis, 1989). We expect that in the case of e-learning technology, the usefulness is not easily differentiable since students need to use an online platform in order to do e-learning. However, the ease of use of the system can indeed influence their perception of e-learning success, particularly when educators use different software for assisting learning, with different levels of ease of use. The easier the interface between the end user and the technology, the higher the likelihood that students will be motivated to participate in e-learning, and yield higher levels of satisfaction, intend to use the technology again, and perceive higher levels of efficiency and effectiveness. However, the relationships may not be direct. Technological features may facilitate, or hamper, successful use of a technology given internal drivers towards the technology. For example, ease of use coupled with existing online skills in technology use would result in the highest level of satisfaction, while low ease of use coupled with lack of skills would result in low levels of satisfaction. Hence it is hypothesized that:
Hypothesis 1: A high level of online skills with e-learning technology coupled with high ease of use of the technology will generate higher e-learning outcomes than a low level of online skills with technology coupled with low ease of use of the technology.
Similarly, the relationship between attitudes towards the technology and satisfaction of e-learning would become more prominent when technology of high ease of use coexists. Thus,
Hypothesis 2: Higher levels of attitude towards technology coupled with high ease of use of the technology will generate higher e-learning outcomes than lower levels of attitude towards technology coupled with low ease of use of the technology.
Students who show strong personal innovativeness in information technology (PIIT) is likely to enroll in e-learning classes. Nevertheless, the likelihood of learners returning for another e-learning class will be influenced by the experience with the learning environment. For example, when the perceived difficulty to maneuver the technology in e-learning is high, the willingness to try the technology again may be deterred by the unfavorable e-learning environment. Therefore,
Hypothesis 3: Strong personal innovativeness in IT coupled with high e-learning technology ease of use will generate higher e-learning outcomes than low personal innovativeness in IT coupled with low e-learning technology.
Prior online experience may help students prepare for e-learning (Gunawardena & Duphorne, 2000; Rodriguez et al., 2008); however, as noted earlier, there are different technology mediums to conduct e-learning with various levels of ease of use. Thus, the choice of technology would affect how students’ experiences in an e-learning environment. Hence,
Hypothesis 4: Prior online experience coupled with high e-learning technology ease of use will generate higher e-learning outcomes than the absence of online experience coupled with low e-learning technology ease of use.
Institutional Support
Besides technological issues, institutional issues constitute factors that are external to an individual. Institutional factors include the support students perceive from the institution offering the e-learning course (Govindasamy, 2002; Phipps & Merisotis, 2000). Support may come from the instructor’s ability and skill to teach the course, and the availability and skill of the technical staff. This external condition may facilitate, or hamper, e-learning satisfaction that is driven by internal factors. For example, those with high online skills may be satisfied with e-learning taken in an institution where the support is high, and vice versa. Hence,
Hypothesis 5: A high level of online skills coupled with a high level of institutional support will generate higher e-learning outcomes than a low level of online skills coupled with a low level of institutional support.
Similarly, positive attitudes towards technology would be magnified when there is strong institutional support for an e-learning technology, giving us,
Hypothesis 6: Strong attitudes towards technology coupled with a high level of institutional support will generate higher e-learning outcomes than weak attitudes towards technology coupled with a low level of institutional support.
The likelihood of e-learning satisfaction perceived by students that have high PIIT would be increased when the level of institutional support is high. On the other hand, students low on PIIT will have low levels of satisfaction especially when institutional support is low. Therefore,
Hypothesis 7: Strong personal innovativeness in IT coupled with a high level of institutional support will generate higher e-learning outcomes than weak personal innovativeness in IT coupled with a low level of institutional support.
Prior experience with online technology would yield positive satisfaction with e-learning when the level of institutional support is high, while lack of prior experience with low intuitional support would result in low levels of satisfaction. Thus,
Hypothesis 8: Previous online experience equipped with a high level of institutional support will generate higher e-learning outcomes than none-online experience equipped with a low level of institutional support.
Our research model with the variables of interest is presented in Figure 2.
Methodology
Survey data from a sample of 280 students in a large southern U.S. university was used for the analysis. The sample was selected from a pool of business students who had taken a course that employed e-learning technologies.
Validity and Reliability
Validity measures the extent to which the scale represents the construct accurately. Two steps are taken to ensure the validity of the constructs measurement in this study. First, an initial version of the survey was piloted with a total of 64 students enrolled in upper division management courses. The pilot study helped test for the psychometric validity of the scales, and to improve the questionnaire used for the survey. Second, when possible, items from validated pre-existing scales in literature were used. For example, personal innovativeness with IT was measured using the PIIT scale from Agarwal and Prasad (1998).
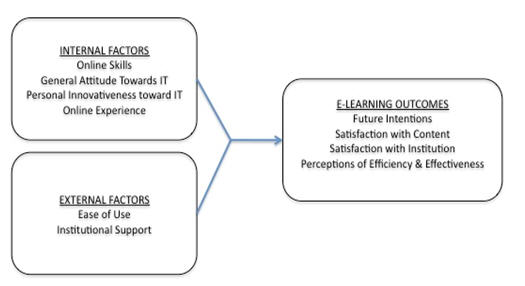
Figure 2 . Research Model
The reliability of constructs is checked by using Cronbach’s alpha. A value that is around 0.6 is considered acceptable, and the higher the value, the better the reliability (Bollen, 1989). The alpha value of all constructs are above 0.7 (see Table 1), indicating reliabilities of constructs acceptable for further analysis. A description of constructs is also provided in the same table. The correlation matrix is provided in Table 2. Online experience is a dichotomous measurement, where we ask students whether they have experience with on-line communication before, and therefore it is not included in the table.
Table 1 : Construct Reliabilities
|
Constructs |
Cronbach’s alpha |
Median |
External Factors |
Ease of use |
0.79 |
.055 |
Institutional support |
0.77 |
.205 |
Internal Factors |
Personal innovativeness towards IT |
0.84 |
.059 |
Online skill |
0.86 |
.194 |
General attitude towards IT |
0.78 |
.116 |
E-Learning Outcomes |
Intention to take more e-learning courses |
0.81 |
.096 |
Satisfaction with e-learning content |
0.92 |
.156 |
Satisfaction with the institution |
0.85 |
-.018 |
Efficiency and effectiveness |
0.89 |
.107 |
|
Table 2 . The correlation matrix provides the correlations between the constructs of interest in the study, with significant relationships indicated. The interesting correlation values are discussed in the section of analysis and results.
|
EOU |
Support |
PIIT |
Skill |
Online Experience |
Attitude |
Intention |
Content Satisfaction |
Institution Satisfaction |
Efficiency Effectiveness |
EOU |
1 |
|
|
|
|
|
|
|
|
|
Support |
.010 |
1 |
|
|
|
|
|
|
|
|
PIIT |
.014 |
.008 |
1 |
|
|
|
|
|
|
|
Skill |
.025 |
.001 |
.026 |
1 |
|
|
|
|
|
|
Online experience |
.077 |
-.009 |
-.021 |
-.028 |
1 |
|
|
|
|
|
Attitude |
.027 |
-.016 |
.017 |
.058 |
-.062 |
1 |
|
|
|
|
Intention |
.038 |
.105 |
.101 |
.232** |
-.256** |
.270** |
1 |
|
|
|
Content Satisfaction |
.376** |
-.003 |
.101 |
.113 |
.028* |
.180** |
.014 |
1 |
|
|
Institution Satisfaction |
.113 |
.237** |
.138* |
.163* |
-.116 |
.-166* |
.016 |
.072 |
1 |
|
Efficiency Effectiveness |
.155* |
-.008 |
-.002 |
.214** |
.050 |
.241** |
.068 |
.042 |
.024 |
1 |
Note: ** correlation is significant at the 0.01 level ,
* correlation is significant at the 0.05 level
|
Analysis and Results
It is interesting to notice that the correlation between general attitude towards IT and satisfaction with the institution is negative and significant (-.116*). The negative correlation suggests that increasing general attitudes towards IT will decrease the satisfaction of students towards the institution that provides e-learning. This seemingly contradictory effect of attitudes indicates a necessity to conduct a more detailed analysis using stratified datasets. Specifically, we use the median of internal and external construct scores to divide the sample, such that we could compare, for example, the sample where students who scored high on both ease of use and online skill, with the sample where students scored low on the both constructs. Non parametric analysis is then applied to compare the difference of medians of dependent constructs (Intention to take more e-learning courses, satisfaction with content, satisfaction with institution, and efficiency and effectiveness). The result of nonparametric analysis is present in Table 3.
Hypotheses one to four stress the effect of external construct—ease of use of technology—and four internal constructs—online skill, general attitudes towards IT, personal innovativeness in IT and online experience.
Hypothesis 1 states that a high level of online skills with technology of high ease of use will generate better e-learning satisfaction than a low level of online skills with technology of low ease of use. In Table 3, significant differences between medians of the stratified datasets are identified for three of four outcomes—Intention to take more e-learning courses, institutional satisfaction and efficiency and effectiveness. The median of content satisfaction is higher for the dataset of high level of online skills and high ease of use than that of low level of online skills and low ease of use, although the difference is not significant. In general, this hypothesis is supported.
Similarly, Hypothesis 2 describes that a strong attitude towards technology and high ease of use will generate better e-learning satisfaction than weak attitude towards technology and low ease of use. We find significant differences for two outcomes, intention to take more e-learning courses and efficiency & effectiveness. Institutional satisfaction is lower for dataset of strong attitude and high ease of use than that of weak attitude and low ease of use, nevertheless, this difference is not significant. Hypothesis 2 could be considered as supported.
Table 3 : Non-parametric Analysis
|
Intention |
Content satisfaction |
Institutional satisfaction |
Efficiency & effectiveness |
High EOU & High Skill vs.
Low EOU & Low Skill |
.249/.070† |
.153/.023 |
.298/.052† |
.098/-.067** |
High EOU & High Attitude vs.
Low EOU & Low Attitude |
.242/-.281*** |
.303/.003 |
-.163/.054 |
.232/-.237*** |
High EOU & High PIIT vs.
Low EOU & Low PIIT |
.015 / -.105 |
.298/-.215 *** |
.189 / -.070 |
.158 / -.081** |
High EOU & High Experience vs.
Low EOU & Low Experience |
.242/-.271** |
.293/.309 |
.006/-.266† |
.131/.123 |
High Support & High Skill vs.
Low Support & Low Skill |
.247/-.154* |
.240/.171 |
.061/-.164 |
.063/.209 |
High Support & High Attitude vs.
Low Support & Low Attitude |
.365/-.271*** |
.112/.040 |
.120/.103 |
.052/-.016 |
High Support & High PIIT vs.
Low Support & Low PIIT |
.128/-.165† |
.223/-.084* |
.312/-.040† |
.053/.073 |
High Support & High Experience vs.
Low Support & Low Experience |
.100/.-271 † |
.085/.289 |
-.047/.080 |
.073/.125 |
|
Note:
*** difference is significant at the 0.001 level
** difference is significant at the 0.01 level
* difference is significant at the 0.05 level
† difference is significant at the 0.10 level
|
In testing Hypothesis 3, we found significant differences for two outcomes, content satisfaction and efficiency & effectiveness, indicating strong personal innovativeness in IT and high ease of use will generate better e-learning satisfaction than low personal innovativeness towards IT and low ease of use. Hypothesis 3 is supported.
Hypothesis 4 points out that previous online experience and high ease of use will generate better e-learning satisfaction than none-online experience and low ease of use. The difference is significant for two outcomes, intention to take more e-learning courses and institutional satisfaction. Hypothesis 4 is supported.
Hypotheses five to eight focus on datasets of external factor—institutional support—and four internal constructs. Hypothesis 5 states that a high level of online skills and a high level of institutional support will generate better e-learning satisfaction than a low level of online skills and low level of institutional support. We only find significant difference for one outcome, intention to take more e-learning courses. Hypothesis 5 is partially supported. Hypothesis 6 is also partially supported as we only found significant difference for one outcome, intention to take more e-learning courses. In testing hypothesis 7, a high level of institutional support and high personal innovativeness in IT leads to significantly higher medians for three of four outcome measures than a level low institutional support and low personal innovativeness in IT, indicating Hypothesis 7 is supported. Hypothesis 8 tests whether previous online experience and a high level of institutional support will generate better e-learning satisfaction than none-online experience equipped and a low level of institutional support. Significant difference is found for one measurement, intention to take more e-learning courses. The hypothesis 8 is partially supported.
Discussion and Conclusion
While e-learning is becoming increasingly important to higher educational institutions, literature on the success of e-learning at an individual level remains fragmented and inconclusive. This paper makes two contributions to research. First, prior research on individual e-learning success is synthesized by identifying relevant empirical literature. In doing so, inconsistencies in empirical findings, which have resulted in inconclusiveness of factors that influence individual e-learning success, are highlighted.
Table 4 : Results for Hypotheses
Hypothesis |
Result |
Support Received |
H1 |
Significant for Intention, Institutional Satisfaction and Efficiency & Effectiveness |
Strongly Supported |
H2 |
Significant for Intention and Efficiency & Effectiveness |
Partially Supported |
H3 |
Significant for Content Satisfaction and Efficiency & Effectiveness |
Partially Supported |
H4 |
Significant for Intention and Institutional Satisfaction |
Partially Supported |
H5 |
Significant for Intention only |
Partially Supported |
H6 |
Significant for Intention only |
Partially Supported |
H7 |
Significant for Intention, Institutional Satisfaction and Content Satisfaction |
Strongly Supported |
H8 |
Significant for Intention only |
Partially Supported |
|
Second, a theoretical framework is offered, which may account for these inconsistencies by specifying the simultaneous role played by both internal and external factors. We term this framework the Internal-External Model. In the analysis, most hypotheses are supported. A favorable external environment for e-learning together with a strong internal drives to take e-learning would in general lead higher e-learning outcomes. The results explain inconclusiveness of prior empirical research that considered only a single, or a few factors, in isolation. We find that it is important to study factors in relation to one another in a unified framework, in order to understand their influence on e-learning outcomes.
It is also interesting to notice different combinations of internal and external factors differently impact the four outcomes. Intention to take more e-learning courses, and efficiency and effectiveness, received more significant support than the satisfaction-related outcomes. This result implies that the choice of measurement of e-learning success matters for empirical analysis, particularly when educators have different focuses for different e-learning designs. This result also brings into question the use of satisfaction as a proxy for IT related outcomes. We find that levels of satisfaction may not necessarily vary with variance in combinations of internal and external factors, but other outcomes such as intention to take other e-learning courses are indeed influenced. Comparing results of different e-learning courses may also involve additional analysis of the comparability of the course in concern, which provides one area of the current analysis for future studies to improve.
As with most research, our study is limited in generalizability due to the sampling constraints of gathering data at one university. Future research may benefit from gathering data from multiple universities. One particular avenue for research might be comparing e-learning outcomes between universities that are at different levels of maturity in their e-learning offerings. Generalizability may also be strengthened by studying universities in different countries or regions to look at the effects of culture on e-learning outcomes.
References
Agarwal, R. & Prasad, J. (1998). A conceptual and operational definition of personal innovativeness in the domain of information technology. Information Systems Research, 9, 204-215.
Bailey, J.E. & Pearson, S.W. (1983). Development of a tool for measuring and analyzing computer user satisfaction. Management science, 29, 530-545.
Bernard, R. M. Rubalcava, B. R., & St-Pierre, D. (2000). Collaborative online distance learning: Issues for future practices and research. Distance Education, 21, 260-277.
Bilalis, N., Dalivigas, A., Antoniadis, A., Athanasaki-Michailidou, C. (2002). A methodology for evaluating software tools for the development of e-learning systems. Session paper at the International Conference on Engineering Education, August 18-21, Manchester, UK.
Bleicher, J. 1982. Hermeneutic Imagination: Outline ofa Positive Critique of Scientism and Sociology. London: Routledge.
Bray, E., Aoki, K. & Dlugosh, L. (2008). Predictors of learning satisfaction in Japanese online distance learners. International Review of Research in Open and Distance Learning,9, 1-24.
Cardosa, E., Trigueiros, M.J. & Narciso, P. (2005). Balanced Scorecard Approach for Strategy- and Quality-driven Universities . Presented at the 11th International Conference of European University Information Systems (EUNIS 2005) – Leadership and Strategy in a Cyber-Infrastructure World, Manchester, June 2005.
Cohen, J. (1992). A Power Primer. Psychology Bulletin, 112, 155-159.
Davies, J., & Graff. M. (2005). Performance in e-learning: Online participation and student grades. British Journal of Educational Technology, 36, 657-663.
Davis, F. D. (1989). Perceived usefulness, perceived ease of use, and user acceptance of information technology. MIS Quarterly, 13, 319-340.
Dede, C. (2004). Planning for "Neomilenial" Learning styles: Implications for Investments in Technology and Faculty. In Educating the Net Generation. Oblinger, D. & Oblinger, J. (Editors). Available online at educause.edu/ir/library/pdf/pub7101o.pdf
Eastmond, D. V.(1994). Adult distance study through computer conferencing. Distance Education, 15,128-152.
Engelbrecht, E. (2003). A look at e-learning models: investigating their value for developing an e-learning strategy. Progressio, 25, 38-47.
Eom, S., Wen, J., & Ashill, N. (2006). The Determinants of Students’ Perceived Learning Outcomes and Satisfaction in University Online Education: An Empirical Investigation. Decision Sciences Journal of Innovative Education, 4, 215-235.
Garrison, D. R. & Anderson, T. (2003) E-Learning in the 21 st Century: A Framework for Research and Practice, Taylor & Francis, New York.
Gernard, R., Abrami, P. C., Lou, Y., Borokhovski, E., Wade, A., Wozney, L., Wallet, P. A., Fiset, M. & Huang, B. (2004). How does distance education compare with classroom instruction? A meta-analysis of the empirical literature. Review of Educational Research, 74, 379-439.
Goda, Y. (2008). Factors Affecting Learners’ Overall Satisfaction in e-Learning as a Wholistic Evaluation in Japan. In K. McFerrin et al. (Eds.), Proceedings of Society for Information Technology & Teacher Education International Conference 2008 (pp. 389-394). Chesapeake, VA: AACE.
Govindasamy, T. (2002) Successful implementation of e-Learning: Pedagogical considerations, The Internet and Higher Education, 4, 287-299.
Gunawardena, C., Carabajal, K, & Lowe, C. A. (2001). Critical analysis of models and methods used to evaluate online learning networks. Paper presentation at American Educational Research Association Annual Meeting, April 11, Seattle, WA.
Gunawardena C. N. & Duphorne, P. L.(2000). Predictors of learner satisfaction in an academic computer conference. Distance Education, 21, 101-117.
Gunawardena, C. N. & Zittle, F. (1997). Social presence as a predictor of satisfaction within a computer mediated conferencing environment. American Journal of Distance Education, 11, 8-25.
Harasim, L., Hiltz, S. R., Teles, L. & Turoff, M. (1995). Learning Networks. Cambridge, MA: MIT Press.
Harper, K., Chen, K., & Yen, D. (2004). Distance Learning, Virtual Classrooms, and Teaching Pedagogy in the Internet Environment. Technology in Society, 26, 585-598.
Hew, Khe F. & Cheung, Wing S. (2003). Models to evaluate online learning communities of asynchronous discussion forums. Australian Journal of Educational Technology, 19, 241-259.
Holsapple, C., & Lee-Post, A. (2006). Defining, Assessing, and Promoting E-Learning Success: An Information Systems Perspective. Decision Sciences Journal of Innovative Education, 4, 67-85.
Jackson, M.J. & Helms, M. (2008). Student Perceptions in Hybrid Courses: Measuring and Interpreting Quality. Journal of Education for Business, 7-12.
Keller, C., & Cernerud, L. (2002). Students’ Perceptions of E-learning in University Education. Journal of Educational Media, 27, 55-67.
Lombard, M. & Ditton, T. (1997). At the heart of it all: The concept of presence. Journal of Computer-Mediated Communication. 13. Available online at http://jcmc.indiana.edu/vol3/issue2/lombard.html
Moore, M. G. (1989). Editorial: Three types of interaction. American Journal of Distance Education, 3, 1-7.
Mupinga, D. (2005). Distance Education in High Schools. The Clearing House , 78, 105-109.
Phipps, R., & Merisotis, J. (2000). Quality on the Line: Benchmarks for Success in Internet-Based Distance Education. Washington, DC: Institute for Higher Education Policy.
Ray, C., Sormunen, C., & Harris, T. (1999). Men’s and Women’s Attitudes Toward Computer Technology: A Comparison. Office Systems Research Journal, 17, 1-8.
Rodriguez, Michael C., Ooms, A. & Montanez, M. (2008). Students’ perceptions of online-learning quality given comfort, motivation, satisfaction, and experience. Journal of Interactive Online Learning, 7, 105-125.
Sanders, P. 1982. Phenomenology: A New Way of Viewing Organizational Research. Academy of Management Review, 7, 353-360.
Short, J., Williams, E. & Christie, B. (1976). The social psychology of telecommunications. London: John Wiley & Sons.
Siritongthawon, S. & Krairit, D. (2004) Use of Interactions in E-learning: A Study of Undergraduate Courses in Thailand. International Journal of The Computer, the Internet and Management, 12, 162-170.
So, Hyo-Jeong & Brush, T. A. (2008). Student perceptions of collaborative learning, social presence and satisfaction in a blended learning environment: Relationships and critical factors. Computers & Education, 51, 318-336.
Sun, P., Tsai, R. J., Finger, G., Chen, Y. & Yeh, D.(2008). What drives a successful e-learning? An empirical investigation of the critical factors influencing learner satisfaction. Computers & Education, 50, 1183-1202.
Tait, A. & Mills, R. 1990. The convergence of distance and conventional education patterns of flexibility for the individual learner. Monografi Publisher: London New York Routeledge.
Taylor, C. (1971). Interpretation and the sciences of man. Review of Metaphysics, 25, 3-51.
Wang, M-Y. (2003). The strategic role of digital libraries: issues in e-learning environments. Library Review, 52, 111-116.
Appendix: Survey Instrument Sample Questions
Please indicate to what extent you agree with the following statement, using a five-point Likert scale.
Online Skills (from Rodriguez, Ooms, & Montanez, 2008)
Sample questions: Assuming that the technology you are using is functioning well, how comfortable are you with the following tasks (from very uncomfortable to very comfortable)?
a. Accessing the web
b. Sending/ Receiving documents electronically
General Attitude Towards IT (from Ray, Sormunen, & Harris, 1999)
Sample questions: Computers and technology have made students more productive
(from strongly disagree to strongly agree)
a. I prefer getting information from a printed page instead of computer screen. (Reverse coded)
Personal Innovativeness in Information Technology (from Agarwal and Prasad, 1998)
Sample questions (from strongly disagree to strongly agree):
a. If I heard about a new information technology, I would look for ways to experiment with it.
b. Among my peers, I am usually the first to try our new information technologies.
Online Experience
Sample questions (from strongly disagree to strongly agree):
a. How many online courses have you taken (1-4, more than 4)
Ease of Use (from Davis, 1989)
Sample questions (from strongly disagree to strongly agree):
a. I found the e-learning software program is easy to use even without special training.
Institutional Support
Sample questions (from strongly disagree to strongly agree):
a. I found [the university’s] technical support is helpful in solving e-learning technological problems.
b. I found the technical support from [the university] is helpful in solving e-learning technical problems.
Future Intentions
Sample questions (from strongly disagree to strongly agree):
a. I would consider registering for another online course.
b. I would advise a fellow student to take an online course
Satisfaction with Content (from Goda, 2008)
Sample questions (from strongly disagree to strongly agree):
a. I feel that the content provided in the e-learning course was sufficient.
b. I was satisfied with the content from additional resources and links (to other web sites)
Satisfaction with Institution
Sample questions (from strongly disagree to strongly agree):
a. My perception of [the university’s] focus on student satisfaction changed because of the e-learning course
Perceptions of Efficiency and Effectiveness
Sample questions (from strongly disagree to strongly agree):
a. I felt that e-learning will/have greatly save(d) my time, it is an efficient way to learn.