Crowdsourcing Higher
Education: A Design Proposal for Distributed Learning
|
Michael Anderson
Director
of Online Learning
University of Texas at San Antonio
San Antonio, TX USA
Michael.Anderson1@utsa.edu
Abstract
Higher
education faces dual challenges to reduce expenditures and
improve learning outcomes through faster graduation rates. These
challenges can be met through a personalized learning system (PLS) that
employs techniques from previous successful instructional designs.
Based on social learning theory, the PLS combines a dynamic menu that
tracks individual topic mastery with a social communication interface
that connects knowledge-seekers with knowledge-providers. The
crowdsourced generation of content from these connections is stored in
a library for availability when live mentors are unavailable. The
quality of the generated stored content is vetted against subsequent
performance by knowledge-seekers whose performance creates recognition
for knowledge-providers in a game-like public rating scheme.
Keywords:
crowdsourcing, social, personalized, intrinsic motivation
|
The
Problem of Efficiency and Effectiveness
Higher education faces a
dilemma: institutions are being expected to
simultaneously reduce expenditures and improve learning outcomes
through faster graduation rates. In some states such as Texas, legal
requirements now stipulate demonstrable improvement in student
graduation rates (Texas Higher Education Coordinating Board, September,
2010). The quandary is not unique to Texas, although the problem is
more severe in Texas than in most states: Texas ranks 35th in the
nation for graduation within six years (Austin American-Statesman,
2010, July 13). Nationally, rates for graduation within six years have
improved only marginally from 52% to 55% over the last decade (NCHEMS,
2011), while rates for graduation within four years from public
universities has declined to 29% in 2008, the latest year for which
data is available (Horn, 2010). Retention (or continuation) between
first- and second-year new students offers another measure of
effectiveness. Between 2004 and 2010, the third and fourth national
surveys, ACT and the National Center for Higher Education Management
Systems (Habley & McClanahan, 2004; Habley, et.al, 2010)
found that the overall retention rate was unchanged over six years at
approximately 67%. With longer graduation times and stagnant retention
rates, little wonder that the American public views higher education
progress as minimal.
At the same time, the cost of
public higher education has risen even
faster than the cost of healthcare (Langfitt, 1990). Increased costs
have primarily impacted the pocketbooks of students as public
appropriations for universities over the last decade have declined an
average of 5.6% annually adjusted for inflation (Southern Regional
Education Board, 2010). Private universities are not significantly
better off. Although the average tuition and fee increase at private
institutions over the last decade was only 3.0% per year compared with
5.6% per year at public four-year schools (Baum & Ma, 2010), the
number of students with loans at private institutions rose 50% from
1993 to 2008 (Baum & Ma, 2010). Average student loan debt increased
24% from 2004 to 2008 according to The Project on Student Debt (2010),
and the average debt for graduates of private universities is now more
than $27,000 according to Lataif (2011) who calls this trend
"unsustainable." In 2010, student loan debt exceeded credit card debt
for the first time to become the largest financial obligation for
Americans (Lewin, 2011). Little wonder that the American public views
higher education as increasingly unaffordable. The combination of
rising costs and perceived low performance is reflected in the public's
lack of confidence in higher education to deliver a worthwhile service
(Texas Public Policy Foundation, December 6, 2010).
To meet the twin demands of
effectiveness and efficiency, the academy
must adopt a revolutionary approach to instruction which integrates
contemporary learning theories with recent research from information
science. While the path of instructional technology is littered with
the unfulfilled promises of all-encompassing answers, a possible
solution is emerging. The growing availability of low-cost computer
networks, capable of linking novices and experts in social and
contextual environments, reduces the inherent friction of production
and elaboration in higher education. Learners no longer need travel to
Cambridge to take a media literacy course from Henry Jenkins; they can
watch his lecture on an iPhone™ or friend him on Facebook™ and chat
about the utility of social networks. Using networks for distributed
learning can solve the efficiency challenge if the academy can embed
that learning in effective instruction.
A Proposed Solution
If networked communities can
increase efficiency, learning networks
that provide effective outcomes offer a solution to the dilemma faced
by higher education. Retention research suggests a potential
appropriate community: a Lumina study (Lesik, 2004) found that
successful developmental students were four times more likely to
graduate, a significant factor also identified by Fike (2004). Harvey
& Drew (2006) and Crossling & Heagney (2008) identified
student-centered experiences and formative assessments as two key
predictors of graduation as well as continuation rates. A personalized
mastery-based social learning environment may allow students to
increase course completion rates.
Effective instruction requires
constant adjustment to the learner,
reinforcing mastered concepts and holding out new concepts that are
barely able to be mastered by the learner at that point in his or her
concept knowledge trajectory. Computers can constantly evaluate and
adjust to inputs in an efficient manner, providing personalized
instruction. Computers can also track performance at a granular level
and match learners with experts on the basis of fine-grained
competencies for the purpose of targeted mentoring. Embedding these
metrics within a networked environment facilitates the computer's
information management capabilities across multiple characteristics of
learning interactions and within a computer-mediated socialized
network.
However,
humans can better interpret a lack of understanding of the intermediate
steps in a problem-solving process, and humans can offer complementary
explanations easily adjusted based on feedback. Digital capture of
these explanations can be archived for use by other students, and the
quality of those digital artifacts can be verified by the performance
of the student consumers, resulting in a collection of diverse and
proven solutions.
Proposal :
Integrating computerized management for individualized tracking with
system-allocated human guidance for problem interpretation via online
transactions among socialized learners can provide an effective and
efficient instructional environment.
Crowdsourcing Instruction
Crowdsourcing views humans as
processing units which can be integrated
with computer processors to draw on the unique strengths of each
(Alonso, 2011). Crowdsourcing is not a group of people performing a
task typically performed by an individual, but rather an approach that
leverages the individual strengths of human and machine processing. For
example, image recognition is a human strength. Humans can recognize
the now familiar reCaptcha web security words shown in Figure 1 faster
and more accurately than the computer that displays the text image.
However, that same computer can sort the reCaptcha image-word pairs
faster and more accurately than the human coders. The allocation of
human guidance in micro-work systems such as Amazon Turk relies on
crowdsourcing.
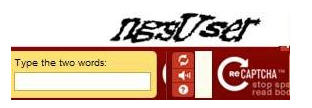
Figure 1. reCAPTCHA ™. Automated system to combine human and
machine strengths in image-to-text processing.
Computers excel at manipulating large data sets, while humans
excel at interpreting data.
Brabham (2008) argues that crowdsourcing offers a solution to complex
problems that require both types of computing: human and machine,
interpreting and manipulating. Because of the concealed nature of
individual cognition, the construction of effective educational
experiences requires the intervention of humans; however, efficiency
may also be realized if appropriate machine computing provides support
for data management and resource allocation. Surowiecki (2004)
maintains that crowdsourcing "wisdom" requires independent,
decentralized answers with cognitive variety, properties that are
characteristic of a collection of solutions created and rated by
individuals. As a result, this design proposal employs crowdsourcing as
a mechanism to match knowledge-providers with knowledge-seekers within
a defined group and to also provide seekers with a diverse solution set
through the digital capture of provider-seeker interactions.
A Design Scenario
Combining a crowdsourced
knowledge- mapping system with solution
capture builds a library of solutions for future use. Instruction at
the individual level that identifies topical areas requiring assistance
can offer both previously captured solutions and synchronous human
explication. Consider the following scenario:
It's 10 pm, and Will is
working on his assigned Chemistry 101 homework.
He logs into his personal learning system (PLS), and the Chemistry
course menu shows he left off his last session at "Balancing Equations"
so he decides to tackle that topic this evening. The PLS assigns random
problems from the "balancing" topic, and Will works a couple of
problems correctly, then misses a couple. After working on the problem
set and failing to correctly answer four consecutive questions, the PLS
soon offers him the choice of watching a video or talking with another
student. Will watches the video, but when he tries the problem set
again, he is still unable to correctly answer four problems in a row
and decides he needs to talk with someone. The system matches him
randomly with Miguel, another Chemistry 101 student who is online and
who has already mastered the topic. The system launches a semi-private
(first names only) voice-enabled whiteboard with the last problem Will
missed on the screen. Miguel talks Will through the problem and offers
hints on how he (Miguel) approaches the "balancing" topic, in this
case, by starting with the atom with the largest coefficient. The
whiteboard session is limited to five minutes, so neither person
dawdles; at the same time, because Will can complete a brief survey at
the end of the session about Miguel's helpfulness, Miguel tries to be
friendly and informal. When the whiteboard session is over, Will
returns to the "Balancing Equations" topic. If he can now correctly
answer four problems in a row, Will’s introductory level for that topic
is marked complete, and the PLS introduces a more difficult set of
"balancing" problems selected by his team of instructors who designed
the assessments. If Miguel's solution engenders Will’s success, Miguel
is credited with a point on the Chemistry leader board. If Miguel
accumulates enough points, he may be offered a teaching assistant job
next year. Miguel's and Will's video session was recorded and added to
the library of videos for the "balancing" topic and awarded a point if
it was successful. Over time, if other students are similarly
successful with the "balancing" topic after watching Miguel's and
Will's video, the session will be publicly recognized as an effective
instructional segment for the topic and will rise to the top of
recommended content objects for that topic.
The creation of effective
instructional media is expensive.
Crowdsourcing the learner-mentor interaction provides a necessary human
interpretation and at the same time creates instructional segments
which are vetted for quality by measuring segment success with both the
immediate learner and future learners.
Theoretical Underpinnings
These interactions, as well as
the personalized learning portal
envisioned, draw on communication and learning theories that have
common roots in social cognition research. Early studies in
computer-mediated communication (CMC) by Sproull & Kiesler (1986)
led to concerns over deindividuation, exacerbated by the innate
anonymity of mechanical intermediaries (telephone, computer) in
interpersonal communication. However, as the increasing ubiquity of
powerful devices with broadband access enabled mediated socialization,
these concerns have been replaced with theories for identifying
intrinsically motivating activities and for understanding learning
transactions.
Computer-mediated
Communication: Group Identification Overcomes Lack of Cues
Using computers as a message
intermediary draws on extensive research
in computer-mediated communication (CMC). The two prevalent theories
view communicative transactions through the lens of either Social
Information Processing (SIP) or the Social Identity model of
Deindividuation Effects (SIDE). Walther (1996) proposed that CMC
filters out cues and temporally retards communication; however, the
expectation of future interaction is processed as socializing
information that ultimately enables deeper interpersonal development.
On the other hand, SIDE proposed that CMC within groups can actually
enhance shared social identity: the process of depersonalization is
accentuated, and cognitive efforts to perceive groups as entities are
amplified (Postmes, et.al., 1998). While the two models
differ in how the effect of CMC anonymity on behavior is explained, the
theories converge on the important role of social groups on identity
formation and communicative behavior in CMC. The crowdsourced PLS works
within the environment of a social group—the class—to reduce
deindividuation effects.
Social Constructivism:
Learning is Social (Networking)
Vygotsky determined that
learning occurs primarily through social
mechanisms. Wertsch & Sohmer (1995) trace two additional themes
from Vygotsky's work: the requirement of a coach (or "more
knowledgeable other") and the identification that learning occurs in
the "zone of proximal development," the region between the learner's
ability to perform a task under the guidance of a coach and the
learner's ability to solve the problem independently. Bandura (1977)
introduced the importance of incentive and identified motivation as one
of four factors (along with attention, retention, and reproduction) for
knowledge modeling in social learning. Lave & Wenger (1990) added
the role of environment by showing that learning occurs in contextual
situations, evolving from "legitimate peripheral participation" to full
participation in an authentic community of practice. John Seely Brown
(1989) extended situated learning to emphasize the role of cognitive
apprenticeship. The crowdsourced PLS is based on social learning
experiences embedded in an authentic "just in time" community of
learning: the online mentor provides apprenticeship, and the dynamic
menu continues to increase the depth of the topic to the level needed
by each student individually (for example, an Engineering major needs
more depth in Calculus than a Journalism major).
Flow: Intrinsic Motivation
Compels Time on Task
Csíkszentmihályi (1990)
developed the concept of an immersive state of
consciousness he described as, “flow.” Csíkszentmihályi identified the
characteristics and conditions which produce a flow state in multiple
activities:
- Both expectations and rules
are discernible and provide direction and structure.
- Direct and immediate
feedback (both success and failure) is apparent so
that individuals may negotiate changing demands and adjust performance
to maintain the flow state.
- Goals are attainable and
aligned with personal abilities at the
specific moment; the challenge level of the task and the individual’s
skill are balanced. The activity delivers a sense of personal control
and provides intrinsic reward.
Flow typically has been
associated with leisure activities because of
their intrinsic reward structure, fun. In particular, game designers
such as Salen & Zimmerman (2003) build flow conditions to create
absorbing interactive experiences. The problem assignment mechanism in
the crowdsourced PLS relies on the same clear rules and continually
adjusts the difficulty level to match the learner's current
understanding, offering both stored and live instruction as requested.
These game-like features provide learner motivation which increases
time on task, a key function of improved learning (Chickering &
Gamson, 1987). Success provides continuing motivation via the
self-efficacy of individual achievement.
Social capital in academic
groups is based on session contribution to
subsequent (mentored) students’ successes. The contributions are
recognized via leader board badges and are based on intrinsic
motivation factors identified by Pink (2009). In addition, the
community welfare appeal offers altruistic reasons for mentor
participation, a factor that Rogstadiusa (2011) found increased quality
in crowdsourced mechanisms.
Transactional Distance:
Networked Learning Demands Student-To-Student Interaction
Moore’s (1997) transactional
distance model views non-proximate
learning in CMC as a series of interactions between a learner and three
entities: instructors, content, and other students. This perspective
enables the design of instruction to focus on the triplet of
learner-to-instructor, learner-to-content, and
learner-to-other-students. The crowdsourced PLS offers stored content
sessions and student-to-student live mentoring under the design of the
faculty member who created the assessment scheme.
Theoretical Fusion
These four theoretical
approaches find a common home in a distributed
learning network. An online system that combines the motivation of
personal goal achievement with the socialization aspects of peer
mentoring offers an effective solution. The environmental focus on a
defined group of individuals, students enrolled in a course whose
members anticipate future interaction in the classroom, reduces
anonymity in online mentoring. The integration of game mechanics
increases the use of the system through intrinsic motivators. The
management of personalized learning and the real-world application of
social learning transactions provide efficiency.
Proven Forerunners:
Successful Design Implementations
Instructional design proceeds
from theory, and the growth of design
techniques that rely on learning networks has accelerated in recent
years with the growth of broadband Internet access. Distributed work
teams and community evaluation and guidance have emerged as accepted
methods for solving problems over geographical distances (Resta &
Laferrière, 2007). Public productions offered as open content build
online reputations and become invaluable knowledge stores. The situated
challenges of online multiplayer games have spawned a multi-billion
dollar industry. The common thread in these applications is their
social nature, and the implementation of social learning techniques has
spawned several successful instructional designs.
Collaborative Projects
The application of CMC research
on the importance of group identity has
found expression in computer-supported collaborative learning (CSCL).
Early work in computer-assisted instruction (CAI) demonstrated the
efficacy of self-paced and personalized approaches, but the addition of
human communication can expand discipline-specific application beyond
skill development to analytical levels. Building on Internet-based
communication tools, collaborative projects such as wikis typically
involve student teams working together on projects. These
knowledge-building communities expose students to diverse opinions
where meaning is constructed through negotiation. The non-computer
implementation of this approach is the classroom-based study group. The
addition of network mediation enables spatial independence for students
who may collaborate synchronously from dispersed locations and temporal
independence for students who may cooperate asynchronously. Thus, CSCL
increases the possibility of communicative activities and participation
through ubiquitous access. The crowdsourced mentoring is a
collaborative activity in which the mentor earns recognition and the
learner succeeds. This team approach reduces faculty member workload by
focusing faculty-to-student interactions on assessment.
Supplemental Instruction
(SI)
Treisman (1983) investigated
techniques for improving the performance
of black students in Calculus at the University of California at
Berkeley; his findings on the effectiveness of peer study groups
presaged the development of supplemental instruction (SI) which was
codified by Deanna Martin (Burmeister, 1996) at the University of
Missouri at Kansas City (UMKC). Arendale (1994) identified key
characteristics of the SI model, one of which was tying the design to a
specific course as an archetypal rather than remedial practice; mentors
participate in the class, and a high degree of student-to-student
interaction occurs in SI groups.
Arendale (1996) analyzed data
from the UMKC campus for 16 years:
controlling for motivation, race, previous academic achievement, and
academic discipline, he found that:
- Grades among SI students on
a four-point scale averaged 2.682 compared with 2.292 for non-SI
students in the same courses.
- Attrition (percentage of W,
D and F grades) among SI students averaged
18.213% compared with 32.169% among non-SI students in the same
courses.
This longitudinal study also
notes that the University of Texas
compared SI with non-SI discussion sessions to control for time on task
and found that SI student grade performance and retention was still
significantly higher than non-SI student grade performance and
retention. The crowdsourced PLS is an online implementation of
supplemental instruction, although the PLS mentors are paid in social
recognition instead of cash. SI requires the process to be viewed as
additive, not remedial, which is accomplished in the crowdsourced
mentoring through its optional but omnipresent offering.
Open Educational Resources
(OER)
Exemplified by MIT's Open
Courseware Project, open educational
resources provide course materials for direct access and reuse.
Learners are encouraged to utilize and in some cases, modify and share
improvements in a content collaboration similar to Wikipedia. Websites
such as Sophia.org, SalKhan.org, and Merlot.org offer non- or
inter-institutional collections of learning objects tagged with expert
and student evaluations. Instructional variety serves diverse learning
styles because not every student can learn the same concept through the
same homogeneous content. Extending the open content model, the
University of Manitoba offered an open online course in 2008 which
enrolled more than 2,300 students (Fini, 2009). The PLS solutions
library functions as an open resource, at least for that class at a
specific institution, with diverse content which can be directed to
students on the basis of demographic data, learning patterns, and other
performance metrics captured and indexed by the crowdsourced PLS.
Public Content
Jenkins (2006) argues that
students are digital residents who live in a
participatory age. Participation in the content construction aspect of
learning environments is often characterized by the use of blogs or
discussion boards that ask student to analyze and summarize core
readings in a discipline and encourage (or require) peer responses to
those posts. The pedagogical affordance of analysis by each student
reduces faculty member workload by shifting the responsibility for
knowledge acquisition to each individual learner. The formalization of
blogs and other student-created content is realized in the advent of
student portfolios. Course assignments in a public venue are available
to an articulated audience in a durable format; access to this venue
can enable collaboration and peer assessment. The stored and published
output of crowdsourced mentoring sessions produces public content. The
value of that content is determined and publically recognized with a
game-like leader board. Points are awarded for evidence-based results
(when users of the content solve problems) as well as for subjective
user-based surveys.
Peer Assessment
Peer assessment in and of
itself reduces faculty member workload and
increases student analytical prowess. Implementations of peer
assessment system such as OASYS (Ward, 2004) and WebPA (Loddington, et.al.,
2009) use statistical techniques to adjust weights based on individual
reliability ratios. Quality control mechanisms in the form of peer
rating analysis that contribute to mentor grades also reduce gaming.
Davidson (2011) showed that crowd-sourced grading can provide an
effective assessment of student outcomes. Rubrics tied to goals produce
more precise assessment evaluations by both peers and experts. The
feedback mechanism to recognize successful solution interactions from
mentoring provides implicit peer assessment. Viewing the crowdsourced
PLS as a social group transforms this implicit peer assessment into a
community-based evaluation.
Problem-Based Learning
(PBL)
While acknowledging the
necessity of learning basic facts, social
constructivism approaches learning from the perspective of how those
facts will be utilized as building blocks within the individual
learner's cognitive domain. This approach is exemplified in PBL:
students are situated in a real-world environment which provides
intrinsic motivation through both the authentic context and the
socialization component. PBL relies on dyadic /group communication to
activate prior knowledge which is then elaborated and restructured. The
crowdsourced PLS situates students directly in the learning environment
at the moment of need and relies on human communication to interpret
complex problems. Faculty workload is reduced through the engagement of
external mentors (in real-time or as a content resource from the stored
sessions) who fill the role traditionally assigned to discussion
leaders. Situating these communications in virtual environments,
whether fictional (Liu, 2006) or real (Doering, Scharber, Miller, &
Veletsianos, 2009), provides intrinsic motivation for learners to
remain personally engaged.
Serious Games
Shaffer (2006) delineated a
class of serious games for learning
purposes which he termed “epistemic games,” characterized by asking
students to:
- Develop schematic knowledge
by combining facts (declarative knowledge)
and problem-solving strategies (procedural knowledge) to solve problems
- Develop symbolic knowledge
in solving one problem which can be used to solve other analogous
problems
- Develop social knowledge by
solving problems, discussing the solution
with the community, and repeating this iterative cycle until the
process is internalized
Epistemic games combine PBL
authenticity with the intrinsic motivation
of fun. Scaffolded instruction and the associated concept of fading
(withdrawing support as skill increases) are exemplified by levels of
achievement within the game. The crowdsourced PLS incorporates game
mechanics in the use of topic levels, public recognition of
accomplishment, and a social community that implicitly votes on
acceptance through successful use of the created artifacts. User
control is afforded by the learner's ability to select stored or live
mentoring content.
Design Integration
However, none of these
successful implementations fully integrate the
multiple applicable theoretical views, and thus each implementation
only partially realizes both effective outcomes and cost-efficiency.
Table 1 summarizes the learner advantages and the system disadvantages
of each approach. At the same time, these design implementations of the
four theoretical bases (computer-mediated communication, social
cognitivism, flow, and transactional distance) each contribute a
significant design element:
- Collaborative projects–
active participation in mentoring sessions
- Supplemental Instruction
(SI) – mentoring sessions which are integrated in the instruction
- Open Educational Resources
(OER) – content stored under the Creative
Commons BY-SA license extends the mentoring audience to external
populations defined by the institution
- Public content – stored
videos offered through personalized menus
- Peer assessment –
effectiveness quality ratings derived from subsequent
mastery; social quality determined from a brief post-session survey
- Problem-Based Learning
(PBL) – situated directly in course as the assignment and assessment
engine
- Serious games – level design
in problem menu with immediate feedback;
intrinsic motivation with public leader board; extrinsic motivation
with grades and potential future employment for successful mentors (in
teaching assista nt positions)
Table 1. Instructional Design Approaches
Approach
|
Advantages
for learners
|
System
disadvantages
|
Collaborative
projects
|
Socialization within
group
|
Potential for no
individual accountability
|
Supplemental
Instruction (SI)
|
Socialization within
group
Content placed in
immediate problem context
|
User-initiated (not
embedded)
|
Open Educational
Resources (OER)
|
Socialization within
group
User control
|
Lack of personal
direction
|
Public content
|
Opportunity for
reflection
Motivation from
participation
|
Absence of assessment
and feedback
|
Peer assessment
|
Motivation from
participation
|
Possible system
manipulation
|
Problem-Based
Learning (PBL)
|
Motivation from
participation
Socialization within
group
Content placed in
immediate problem context
|
Requires external
experts
|
Serious games
|
Motivation from
participation
Socialization within
group
Content placed in
immediate problem context
Intrinsic motivation
for learners and mentors
|
Expensive to develop
|
Design Functions
Gamification
The online mentoring sessions
are founded on supplemental instruction
techniques. However, game mechanics contribute significantly to the
dynamic menu design and the concept of public recognition for mentors.
Each student builds a personalized task menu of needed knowledge by
working through faculty-generated course objectives and assessments.
The menu is constantly updated as knowledge is acquired and provides a
self-actualizing progress report as shown in Figure 2. Discrete topics
identified as "in progress" are taught via live or stored mentoring
sessions.
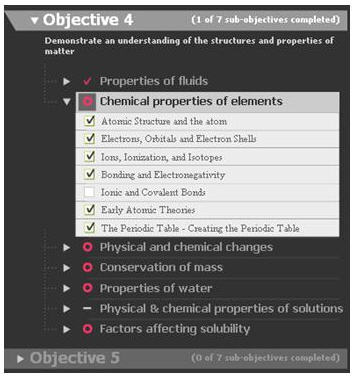
Figure 2.Task menu The dynamic and personalized menu shows mastered and
un-mastered topics
for each student: some are self-selected for study within a learning
objective, while others may be locked
until acquisition of prior requisite knowledge has been demonstrated.
Social interaction
Human interactions in the form
of stored mentoring sessions provide an
anthology of worked examples and address concerns regarding the high
cognitive load inherent in PBL (Kirschner et.al. 2006).
Stored sessions carry success ratios based on subsequent performance by
students using those sessions, and they form a pattern library with
increased payload value based on iterative and covert evaluation. The
stored sessions as shown in Figure 3 offer the increased knowledge
density of spatial memory over declarative and procedural memory
(Gagné, et.al., 1993). In order to accommodate students with
visual impairments, the voice chat audio stream from mentoring sessions
will be automatically captioned to provide a text alternative.
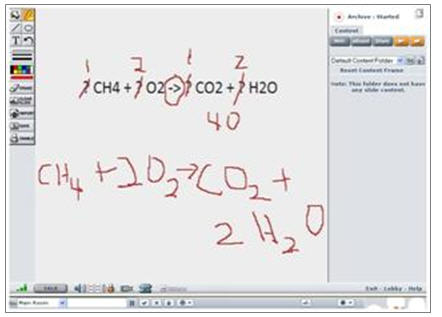
Figure
3. Mentoring whiteboard The whiteboard shows a mentor’s written
explanation drawn
on a chemical equation provided by the PLS as an image. The mentoring
session is accompanied
by voice chat capabilities for both participants. Note (upper right)
that the session is archived as an
MP4 video for incorporation into the stored library if subsequent
student use demonstrates the
video’s effectiveness.
Typical
instructional sequence
Table 2 traces a linear
sequence of events in the crowdsourced PLS, although many interactions
will be iterative.
Table 2. Human/Computer
interaction flow
Step
|
Student
|
Computer
|
Mentor
|
1) Problem generation
|
Logs into system and selects topic
|
Generates algorithmic problem based
on either previous stopping point or target skill level for topic
|
|
2a) Answer evaluation
|
Demonstrates topic mastery
|
Judges answer: if all are correct
after 4 consecutive solutions, return
to Step 1) and assign more difficult problem in same topic
|
|
2b) Answer evaluation
|
Is unable to demonstrate topic
mastery
(primed for assistance)
|
Judges answer: if incorrect, return to
Step 1) and assign parallel
problem in same topic; if 4 successive cycles have occurred (or >6
cycles total), locate stored pattern solution in library or match with
a mentor based on mentor’s demonstrated mastery
|
|
3a) Knowledge retrieval
|
Selects solution from previous stored
session (different student-mentor)
|
Recommends alternative explanations
from library of prior proven solutions
|
|
3b) Mentoring session
|
Interacts with live mentor
|
|
Provides one-on-one mentoring in a
problem-solution format
|
3b’) Knowledge capture
|
|
Captures, tags (with performance
metrics and survey results), and stores mentoring session in pattern
solution library
|
|
4) Knowledge evaluation
|
|
System provides covert feedback on
stored session effectiveness based
on student results (and later, subsequent student results); generates
reputation points for mentor
|
|
Considerations
Noise
Noise is minimized through the
reliance on post-session student
performance. While this reliance provides external validity to the PLS,
the design incorporates additional elements to prevent exploitation and
reduce potentially unpleasant interactions.
Exploitation
Student and mentor identities
are masked (only first names are used)
during the sessions. While physical identities may be recognizable from
classroom interactions, and while mentor recognition is public on the
leader board, student identities are always private in the PLS.
Student-mentor sessions are limited to five minutes, and no images may
be introduced other than the problem assigned by the PLS; this design
implementation limits off-topic interaction in the live mentoring
sessions.
Student-mentor assignments are
made through a topic mastery algorithm.
To the largest extent possible, these assignments are randomized to
prevent collusion and artificial inflation of mentor ratings and stored
session performance metrics. The inclusion of a brief exit survey
regarding student satisfaction at the end of each session produces data
used by the mentor-matching algorithm to avoid matches that create
interpersonal conflict.
Mentor Availability
In order to offer user control,
students choose between live and stored
mentoring sessions. However, this choice presupposes the availability
of willing mentors at the moment the student requests assistance, a
tenuous assumption that is even more suspect at start-up. Further,
until the pattern library is sufficiently robust, even the availability
of stored sessions is questionable. As a result, several initial
priming implementations may be required:
- Load existing third party
content from sources such as MERLOT, the
National Repository of Online Courses, and the Sal Khan Academy until a
sufficient number of local mentor sessions have been captured.
- Staff the mentor pool with
selected T.A.’s to provide coverage until the pool is self-sustaining
from the student community.
- Offer asynchronous support
using a wiki interface for question
collection and knowledge consolidation as an alternative to the
immediacy of either live or stored mentor sessions.
Future Considerations
Written Products
While the writing component of
many courses may be able to benefit from
a common assignment model (such as, “Construct a five-paragraph
argumentative essay on the topic of x, taking the position of
y” with the variables x and y
pulled from a database), the judging of answers to those problems
requires human evaluation despite advances in computerized discourse
assessment. In a writing implementation, crowdsourcing can provide that
assessment. Braddock, et.al. (1963) found that rubric-based
assessment of writing assignments yielded inter-rater reliabilities
ranging from .87 to .96. This high level of reliability suggests that
peer evaluations of written products can be accurate if accompanied by
clear assessment direction.
Non-algorithmic Learning
Not all courses lend themselves
to automated problem generation,
especially courses beyond the developmental and introductory levels.
Non-algorithmic problem sets can substitute crowdsourced assessment in
the answer judging step and follow the remainder of the interaction
flow diagram (see Appendix I) by offering both live and stored
mentoring sessions to implement refinements recommended by the
crowdsourced assessment. Cases, adventures, collaborative projects, and
other team-based designs are encompassed in the interaction flow
diagram by viewing groups of students as a proxy for a single user.
The Generalized Solution
Space
The dual challenges of
efficiency and effectiveness demand a radical
restructuring of the traditional roles in higher education. Faculty
members must cede sole responsibility for direct instruction to
knowledgeable students in exchange for deeper interaction with
individual students. At the same time, faculty members must retain
scope and sequence and assessment control. Students must take ownership
of their own learning in exchange for multiple modes of engagement in
familiar online social venues. At the same time, students must accept
communal responsibility and provide mentoring in a quid pro quo
environment where payment is non-material. Empowered by proven
techniques in social learning design and crowdsourcing, these new
responsibilities promise more effective and efficient learning
outcomes. Unlike previous models that require constant external and
expert maintenance, the PLS is an organic system that grows in value
with use. We must seize connected wisdom today.
Acknowledgement
This paper would not have been
possible without the guidance of Matthew
Lease in the School of Information’s Department of Computer Science at
the University of Texas at Austin. When I was lost among the
algorithms, Matthew helped me to see both the social and mathematical
implications of crowdsourcing. Any failings in this paper are
completely my own, but any contribution this paper makes to the
efficiency and effectiveness debate in higher education are due to
Matthew’s inspired instruction.
References
Alonso, O. (February 9, 2011).
Perspectives on Infrastructure for
Crowdsourcing. WSDM 2011 Workshop on Crowdsourcing for Search and Data
Mining. Hong Kong, China. Retrieved from the Internet October 30, 2011:
http://ir.ischool.utexas.edu/csdm2011/proceedings/csdm2011_alonso.pdf.
Arendale, D. (1994).
Understanding the supplemental instruction model. In D. C. Martin &
D. Arendale, Eds. Supplemental Instruction: Increasing Achievement
and Retention, New Directions for Teaching and Learning, 60. San
Francisco, CA: Jossey-Bass.
Arendale, D. (1996).
Supplemental instruction (SI): review of research
concerning the effectiveness of SI from the University of
Missouri-Kansas City and other institutions from across the United
States. In S. Mioduski & Gwyn Enright, Eds., Proceedings of
the 17th and 18th Annual Institutes for Learning Assistance
Professionals: 1996 and 1997. Tucson, AZ: University Learning
Center, University of Arizona, 1-25.
Austin American-Statesman
. (2010, July 13). Hochberg says only one third of Texas college
students graduate. Retrieved from the Internet on March 22 2011:
http :// politifact . com / texas / statements /2010/ jul /13/ scott -
hochberg / hochberg - says - only - one - third - texas - college -
student /.
Bandura, A. (1977). Social
learning theory. New York, NY: General Learning Press.
Baum, S. & Ma, J. (2010).
Trends in College Pricing 2010. College Board. Retrieved from the
Internet April 30, 2011:
http :// trends . collegeboard . org / downloads / College _ Pricing
_2010. pdf.
Brabham, D. (2008).
Crowdsourcing as a model for problem solving.
Convergence: The International Journal of Research into New Media
Technologies, 14(1), 75-90.
Braddock, R., Lloyd-Jones, R.,
& Schoer, L. (1963). Research in Written Composition.
Champaign, IL: National Council of Teachers of English.
Brown, J. S., Collins, A.
& Duguid, S. (1989). Situated cognition and the culture of
learning. Educational Researcher, 18(1), 32-42.
Burmeister, S. L. (1996).
Supplemental Instruction: An interview with Deanna Martin. Journal
of Developmental Education, 20(1), 22-24, 26.
Chickering, A. W. & Gamson,
Z. F. (1987). Seven principles for good
practice in undergraduate education. American Association for Higher
Education Bulletin. Retrieved from the Internet on March 23, 2011:
http :// honolulu . hawaii . edu / intranet / committees / FacDevCom /
guidebk / teachtip /7 princip . htm.
Crossling, G. & Heagney,
M. (2008). Improving student retention in higher education. Australian
Universities’ Review, 51(2). 9-18.
Csíkszentmihályi, M. (1990). Flow:
the psychology of optimal experience. New York, NY: Harper and
Row.
C. Davidson. (2009, July 26).
How To Crowdsource Grading [Web log]. Retrieved from the Internet
October 31, 2011: http://hastac.org/blogs/cathy-davidson/how-crowdsource-grading.
Doering, A., Scharber, C.,
Miller, C., & Veletsianos, G. (2009).
GeoThentic: Designing and assessing with technology, pedagogy, and
content knowledge. Contemporary Issues in Technology and Teacher
Education, 9(3). Retrieved from the Internet October 31, 2011: http://www.citejournal.org/vol9/iss3/socialstudies/article1.cfm.
Fike, D. S. & Fike, R.
(2008). Predictors of First-Year Student Retention in the Community
College. Community College Review, 36, 68-88.
Fini, A. (2009). The
Technological Dimension of a Massive Open Online Course: The Case of
the CCK08 Course Tools. The International Review of Research in
Open and Distance Learning, 10(5).
Gagné, E. D., Yekovich, C. W.
& Yekovich, F. R. (1993). The cognitive psychology of school
learning, 2nd edition. New York, NY: Harper Collins College
Publishers.
Habley, W.R. & McClanahan,
R. (2004). What Works in Student
Retention? ACT/NCHEMS. Retrieved from the Internet October 30, 2011: http://www.act.org/research/policymakers/pdf/droptables/AllInstitutions.pdf.
Habley, W.R., Valiga, M.,
McClanahan, R., & Burkum, K. (2010). What
Works in Student Retention? ACT/NCHEMS. Retrieved from the Internet
October 30, 2011: http://www.act.org/research/policymakers/pdf/droptables/AllInstitutions.pdf.
Harvey, L. & Drew, S.,
2006: The first-year experience: briefing
paper on integration. Retrieved from the Internet October 31, 2011: http://www.heacademy.ac.uk/assets/documents/research/literature_reviews/first_year_experience_briefing_on_integration.pdf.
Horn, L. U.S. Department of
Education, National Center for Education
Statistics. (2010, December). Tracking students to 200 percent of
normal time: effect on institutional graduation rates (NCES 2011-221).
Retrieved from the Internet on April 27, 2011: http :// nces . ed .
gov / pubs 2011/2011221. pdf.
Jenkins, H. (2006). Confronting
the challenges of participatory
culture: media education for the 21st century. Retrieved from the
Internet on April 2, 2011:
http :// digitallearning . macfound . org / atf / cf /%7 B 7 E 45 C 7 E
0- A 3 E 0-4 B 89- AC 9 C - E 807 E 1 B 0 AE 4 E %7 D / JENKINS _ WHITE
_ PAPER . PDF.
Kirschner, P. A., Sweller, J.,
& Clark, R. E. (2006). Why minimal
guidance during instruction does not work: an analysis of the failure
of constructivist, discovery, problem-based, experiential, and
inquiry-based teaching. Educational Psychologist 41(2).
75-86.
Langfitt, T. W. (1990). The
cost of higher education: lessons to learn from the health care
industry. Change, 22 (6). 10.
Lataif, L. L. (2011).
Universities on the brink. Commentary on Forbes.com. Retrieved from the
Internet April 29, 2011:
http :// www . forbes . com /2011/02/01/ college - education - bubble -
opinions - contributors - louis - lataif . html.
Lave, J. & Wenger, E.
(1990). Situated learning: legitimate peripheral participation.
Cambridge, UK: Cambridge University Press.
Lesik, S. (April, 2004).
Evaluating developmental education programs in higher education. ASHE/Lumina
Policy Brief, 4.
Lewin, T. (2011, April 11).
Burden of college loans on graduates grows. New York Times.
Retrieved from the Internet on April 29, 2011:
http :// www . nytimes . com /2011/04/12/ education /12 college . html
?_ r =2& hp.
Liu, M. (2006). The Effect of a
Hypermedia Learning Environment on
Middle School Students’ Motivation, Attitude, and Science Knowledge. Computers
in The Schools, 22(8). 159-171.
Loddington, S. Pond, K.,
Wilkinson, N. & Willmot, P. (2009). A case
study of the development of WebPA: an online peer-moderated marking
tool. British Journal of Educational Technology, 40(2).
329-341.
Moore, M. (1997). Theory of
transactional distance. In D. Keegan, Ed. Theoretical Principles
of Distance Education. New York, NY: Routledge. 22-38.
NCHEMS Information Center.
(2011). Graduation Rates. Retrieved from the Internet on April 28, 2011:
http :// www . higheredinfo . org / dbrowser /? year =2008& level =
nation & mode = graph & state =0& submeasure =27.
Pink, D. (2009). Drive: The
Surprising Truth About What Motivates Us. Riverhead Books: New York,
NY.
Postmes, T., Spears, R., &
Lea, M. (1998). Breaching or building
social boundaries? SIDE-effects of computer-mediated communication. Communication
Research, 25, 689-715.
Resta, P. & Laferrière, T.
(2007). Technology in Support of
Collaborative Learning. Educational Psychology Review, 19, 65–83. DOI
10.1007/s10648-007-9042-7.
Rogstadiusa, J., Kostakosa, V.,
Kitturb, A., Smusa, B., Laredoc, J.,
& Vukovicc, M. (2011). An Assessment of Intrinsic and Extrinsic
Motivation on Task Performance in Crowdsourcing Markets. Proceedings of
the Fifth International AAAI Conference on Weblogs and Social Media:
Barcelona, Spain.
Salen, K., & Zimmerman, E.
(2003). Rules of play: Game design fundamentals. Cambridge, Mass: MIT
Press.
Shaffer, D. (2006). How
computer games help children learn. New York, NY: Palgrave
Macmillan.
Southern Regional Education
Board. (2010, May). Higher Education
Finance and Budgets. Retrieved from the Internet on April 2 2011: http ://
info . sreb . org / DataLibrary / tables / FB 81_82. xls.
Sproull, L. & Kiesler, S.
(1986). Reducing social context cues:
Electronic mail in organizational communication. Management Science,
32, 1492-1512.
Surowiecki, J. (2004). The
wisdom of crowds: why the many are smarter
than the few and how collective wisdom shapes business, economies,
societies and nations. Boston, MA: Little, Brown.
Texas Higher Education
Coordinating Board. (2010, September).
Recommendations to the 82nd Texas Legislature. Retrieved from the
Internet on March 22 2011:
http :// www . tache . org / LinkClick . aspx ? fileticket = XA - knxZ
7 w 98%3 D & tabid =40& mid =400.
Texas Public Policy Foundation.
(2010, December 6). Texas voters want
more bang for the buck in higher education. Retrieved from the Internet
on March 22 2011:
http :// www . texaspolicy . com / pdf /2010-12- HigherEducationSurvey
- PressRelease . pdf. The Project on Student Debt. (2010). Student
debt and the class of 2009. Retrieved from the Internet on April 27,
2011:
http :// projectonstudentdebt . org / files / pub / classof 2009. pdf.
Treisman, P.U. (1983).
Improving the performance of minority students in college-level
mathematics. Innovation Abstracts 5(15).
Walther, J. B. (1996).
Computer-mediated communication: impersonal,
interpersonal, and hyperpersonal interaction. Communication Research,
23, 3-43.
Ward, A.,
Sitthiworachart, J. & Joy, M. (2004, February 16-18). Aspects of
web-based peer assessment systems for teaching and learning computer
programming. Proceedings of the IASTED International Conference on
Web-Based Education. Innsbruck, Austria.
Wertsch, J. V. & Sohmer,
R. (1995). Vygotsky on learning and development. Human
Development, 38. 332-37.
Appendix I: Interaction
flow diagram
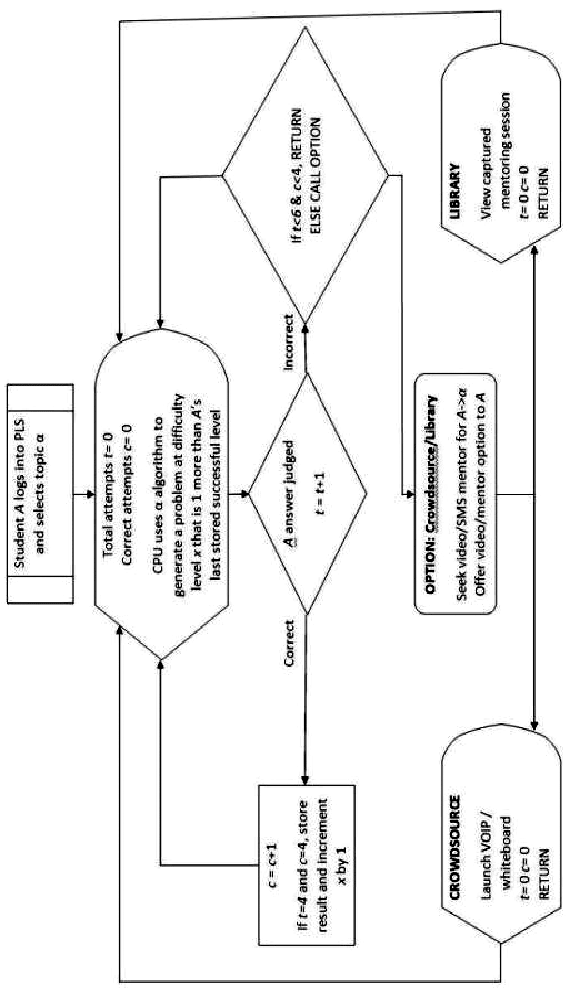