The Use of E-Learning Tools for Improving Hispanic Students' Academic Performance
|
Jennie Johnson
Associate Professor
Department of Management and Marketing
University of Texas at Brownsville
Brownsville, TX 78520 USA
jennie.johnson@utb.edu
Edith Galy
Associate Professor
Department of Accounting and Management Information Systems
University of Texas at Brownsville
Brownsville, TX 78520 USA
edith.galy@utb.edu
Abstract
Most research in higher education related to Hispanic students has focused on institutional-level strategies to determine ways of improving graduation and retention rates. Reports published by the U.S. Census Bureau, Department of Education, and Department of Labor have identified the growth of the Hispanic population in the United States as a challenge for both higher education and the future of the U.S. workforce as university retention, graduation rates, and grade point averages remain low for Hispanics, trailing whites and all other minority groups. At the same time, technology has proliferated in classrooms, institutions have proffered both online courses and complete degree programs, and instructors have increased the use of e-learning tools in both online and campus-based classes. To build upon a limited research base focused on Hispanic student success, the study reported in this paper was conducted at the classroom level in a Hispanic-serving higher education institution. It involved students in a Bachelor of Business Administration program undertaking both online and campus-based courses that made use of various e-learning tools, and examined the impact that technology acceptance constructs had on students' academic performance. Results indicated that three constructs – computer self-efficacy, ability to work independently, and time management – were statistically significant predictors of course performance for Hispanic students. Perceived value and age were used as control variables.
Keywords: Hispanic students, higher education, e-learning tools, computer self-efficacy, working independently, time management, academic performance |
Introduction
"The Hispanic population in the U.S. is continuing to increase, with Hispanic youth currently being the fastest growing segment in the U.S. population" (Maceli & Box, 2010, p. 119). It has been estimated that by 2050, U.S. Hispanics will represent one third of the U.S. population ("Telemundo Launches," 2009). In addition, the U.S. Census Bureau (2012) reports that more than half of the growth of the 27.3 million increase in the total U.S. population between 2000 and 2010 was attributable to the increase in the Hispanic population. This population grew 43%, rising from 35.3 million in 2000 to 50.5 million in 2010. Hispanics comprised 16% of the total U.S. population of 308.7 million (Humes, Jones, & Ramirez, 2011). Based upon projections for the period 2005 to 2050, Hispanics will represent 60% of the U.S. population growth (U.S. Department of Education & White House Initiative on Educational Excellence for Hispanics, 2011). Based upon projections about the growth of the Hispanic population, the U.S. Department of Labor (2012) predicts that Hispanics will drive the U.S. labor market. However, at the same time, the U.S. Department of Education (2011) reports that only 13% of Hispanics hold a bachelor's degree and 4% a graduate or professional degree. Similarly, according to the U.S. Department of Labor, only 16.9% of Hispanics over 25 years old were college graduates, compared to 36.1% of Whites, and 26% of African Americans. As well as having a compounding effect on the labor force, the Hispanic population expansion challenges education at all levels from early learning programs to university studies. Hispanic higher education enrollment is expected to increase faster than other groups and is anticipated to increase 39% by 2017, compared to 5% increase in enrollment for Whites and a 26% increase for African Americans. It is estimated that there are 334 Hispanic-serving institutions (HSIs) currently in the U.S. In 2011, the Department of Education defined an HSI as a public or private college or university that had a student population that is at least 25% Hispanic and served a high portion of low to middle income students.
Although there is an expected rise in Hispanic educational enrollment, research has shown that the Hispanic youth has fallen behind in educational attainment compared to other cultural/ethnic groups (Alon, Domina, & Tienda, 2010). Several studies have suggested that minorities, specifically Hispanics, have fallen prey to the digital divide (Attewell, 2001; Slate, Manuel, & Brinson, 2001; Stanley, 2003). The digital divide has been defined as the "inequities in access to the Internet, extent of use, knowledge of search strategies, quality of technical connections and social support, ability to evaluate the quality of information, and diversity of uses" (DiMaggio, Hargittai, Neuman, & Robinson, 2001, p. 310). Originally, the concept of the digital divide was concerned with Internet availability and barriers to technology use experienced by individuals and groups (Katz, Rice, & Aspden, 2001). As the availability of technology has proliferated, the reference to the digital divide has evolved to evaluating individual and group technology use or non-use (Enoch & Soker, 2006). Minority students seeking higher education may lack the essential technological capabilities and may also be hindered by anxiety regarding the use of technology.
The purpose of this study was to explore Hispanic student attitudes toward e-learning tools, and to measure the explanatory power of these attitudes in predicting their course grade. Furthermore, this study contributes to the existing literature specifically on Hispanic students' academic performance related to e-learning tools. The literature survey and proposed hypotheses presented in the following sections suggest that Hispanic students' academic performance is linked to their computer self-efficacy, their ability to work independently, and their ability to manage time effectively.
Background Literature
Given the trends in the growth of the Hispanic population and corresponding impact on the U.S. labor market, research that attempts to explain and support Hispanic student success in higher education is imperative. Literature in higher education for Hispanics and other minorities has primarily measured academic performance by completion or graduation rate statistics. Most studies have focused on institutional practices and policy choices and their effect on access, and subsequent graduation rates (Lynch & Engle, 2010). Other studies have suggested improving teaching practices used with Hispanic students (Padrón, Waxman, & Rivera, 2002), while others have emphasized the sociocultural perspective of teaching and learning (Tharp, Estrada, Dalton, & Yamauchi, 2000). Fewer empirical studies in HSIs have been conducted that focus specifically on classroom outcomes and variables that predict these outcomes and relate those to student success.
Instructional practices related to Hispanic students have been researched to some degree. According to Padrón et al. (2002), direct instruction was the most common approach found in teaching Hispanic students. This approach emphasizes lecture (Stephen, Varble, & Taitt, 1993), instructor control of the discussion, and decision-making in the classroom (Haberman, 1991). Tharp et al. (2000) suggest that instruction of Hispanics who come from different cultures should be more meaningful and responsive to their particular needs, and according to Vasquez (1990), one way for instruction to be more meaningful is to teach to the distinctive traits of these students. Padrón et al. stress that educators need to know which instructional classroom practices, based upon research, have been found to be most effective with Hispanic students.
Hispanics are grossly underrepresented in selective universities because they are more likely to attain a bachelor's degree where the Hispanic student population represents the majority of the student population in the institution (Alon et al., 2010; Gates, 2010). Gates suggests that Hispanics make a conscious choice to attend HSIs and to teach at HSIs even when they have been accepted into more selective institutions. HSIs therefore provide an appropriate venue for undertaking research related to improving Hispanic student graduation rates. This study was carried out in an HSI where 95% of the students were Hispanic. This institution provided an opportunity to explore Hispanic student success and the factors that contribute to it.
The advent of e-learning, defined as computer networked technology to provide course material and content (Welsh, Wanberg, Brown, & Simmering, 2003) as part of the collaborative learning paradigm includes Web 2.0 technologies that are now making their way into the classroom. Web 2.0 technologies include wikis, blogs, podcasts, social networking sites such as Facebook, video-sharing sites such as YouTube, and virtual worlds such as Second Life. Educators and researchers can anticipate that new technologies will continue to be introduced, which will require adaptation by students, instructors, and researchers exploring their effectiveness. It is important to look for "clues as to how e-learning technologies can become powerful catalysts for change as well as tools for redesigning our learning and instructional systems" (Shroff & Vogel, 2009, p. 60) and what implications those have for Hispanic learners.
The maturing of instructional platforms, also referred to as learning management systems (LMSs), has been another development in recent distance education (DE) history. Saadé and Kira (2009) describe an LMS as a framework that includes instructor tools, learning process tools, and a repository of data. The students used in this study have been exposed to the Blackboard LMS through which students participated in and made use of discussion forums, e-journals, wikis, video-sharing sites, online testing, practice quizzes, and other online tools.
Many formal education institutions have integrated DE into their curriculum format. While campus-based courses continue to be offered, students are increasingly given the option to pursue degrees entirely offered online or to supplement the traditional format with online classes (Allen & Seaman, 2010). Considering the proliferation of students seeking courses online, it is essential to investigate student success in online and campus-based platforms. In their comprehensive review, Bekele and Menchaca (2008) suggest that relevant research should utilize a strong theoretical framework, employ compatible implementation and assessment, and have a clear methodological structure. Although their respective studies were constrained to only online students, Kilic-Cakmak, Karatas, and Ocak (2009) and Seok, DaCosta, Kinsell, and Tung (2010) posit that student preferences regarding instructional design features, communication with the instructor, and assessments used to evaluate their performance require further investigation.
In Fall 2009, the number of students taking at least one online course rose to 5.6 million, a 21% increase from the previous year (Allen & Seaman, 2010). However, DE has evolved as students choose to add online courses onto their existing, campus-based curriculum. In other words, students are seeking to complement, not substitute, campus-based education with online courses. Adding to the validity of this phenomenon is the fact that students take online courses in institutions close to their homes with three fourths of online students living less than 40 miles from their school. Over the last decade, many instructors have moved away from authoritarian and non-interactive instructional methods, and students have gained control in their courses with the aid of two-way communication as well as group-oriented activities. E-learning tools facilitate flexible asynchronous learning because students participate in discussions and group activities online at their own pace and at a time convenient for them. This provides students with a sense of autonomy and control. During the past decade e-learning tools have become prevalent not just in DE courses, but also in campus-based courses (Lou, Bernard, & Abrami, 2006).
Somenarain, Akkaraju, and Gharbaran (2010) found that 93% of first-time users reacted positively to their first experience in either asynchronous or synchronous courses. The high approval rating of first-time online users was attributable to the clear course expectations given to all students in a required 1-hour orientation provided at the beginning of the semester.
Formulation of Research Hypotheses
Research in academic performance has employed a student's grade as an indicator of course performance. Findings from a meta-analysis of research concerning online education of business students indicated that early studies were not able to substantiate differences in test performance. Subsequent studies revealed differences in attitudes about a medium affected student performance as the semester progressed (Arbaugh et al., 2009).
The technology acceptance model (TAM) (Davis, 1989) has been widely used to measure the use of various information technologies and has been accepted as a framework to measure the effectiveness of DE and e-learning tools (Arbaugh, 2005; Manochehri & Sharif, 2010; Martins & Kellermanns, 2004; Unal & Unal, 2011). The model provides insights into student perceptions of various e-learning tools. These perceptions and attitudes play a predictive role in course grades. Although many variables have been studied in relationship to academic performance, few have looked specifically at Hispanic student performance in relation to technology acceptance.
Compeau and Higgins' (1995) review of several studies concerning the measurement of self-efficacy within the computing context resulted in defining computer self-efficacy (CSE) as "a judgment of one's capability to use a computer" (p. 192). CSE is a dynamic construct and reflects more than ability assessment; it forms a critical influence on future intentions. For example, students with greater feelings of preparedness reported a strong relationship with CSE (Mykytyn, Pearson, Paul, & Mykytyn, 2008). Saadé and Kira (2009) found evidence to support the important mediating role of CSE in student usage of LMS such as Blackboard. Ease of use increased LMS usage and created a positive learning experience. Puzziferro (2008) and Galy, Downey, and Johnson (2011) found a correlation between online self-efficacy and student performance, which encourages further exploration into the relationship. Martins and Kellermanns (2004) assessed business students' sense of self-efficacy using the Web and its relationship to perceived ease of use, an element of CSE. Simmering, Posey, and Piccoli (2009) found a strong relationship between CSE and student achievement. This upholds the contention that individuals' confidence in their personal abilities contributes to their knowledge acquisition. Additionally, few studies have specifically examined the role of CSE within the Hispanic student population. Thus, the following hypothesis was formulated for this study:
H1: Self-efficacy, as it relates to e-learning tools, has a positive and significant impact on the course grade.
Online courses and e-learning tools create learning situations where students are more responsible for their own learning. Thus, online courses and the use of e-learning tools engage students in the learning process that Knowles (1975) describes as participating in self-directed inquiry, and Candy (1991) characterizes as learning with predominant learner control. Generally recognized for revisiting the term "andragogy," Knowles (1980) was one of the first theorists to use the terms self-directed learning and self-directed inquiry in his writings related to adult learning. A student's ability to work independently is embodied in this idea of self-directed or self-regulated learning (Manochehri & Sharif, 2010; Shroff & Vogel, 2009; Smart & Cappel, 2006). Considered a pre-entry requirement for enrolling in an online course, a student characteristic associated with online success, which is sometimes referred to as a student success factor or a learning style preference, is the ability to work independently. It has been studied in relationship to student online learning adaptation and success (Artino, 2009; Hung, Chou, Chen, & Own, 2010; Shinkareva & Benson, 2007; Smith, Murphy, & Mahoney, 2003). Independence has been defined as "self-management of studies by working on one's own initiative where a student takes more responsibility for his or her own learning" (Manochehri & Sharif, 2010, p. 36). The authors elaborated that technology has the opportunity to enhance independence with its speed and ubiquitous accessibility, allowing for individualized instruction.
Many university DE departments provide students with the opportunity to self-assess their readiness for online learning via survey instruments. Student ability to work independently and other factors such as academic and life skills, and course expectations are incorporated into such assessments. Haigh (2007) reviewed several universities' DE student self-assessments to create questions to capture the notion of student self-sufficiency or ability to work independently and measured this in a study profiling online and face-to-face information science students. The second hypothesis was articulated as follows:
H2: The ability to work independently has a positive and significant effect on the course grade.
Time-management skills have been researched in relationship to course performance and studies determined that these skills are indeed predictors of course grades (Bliss & Sandiford, 2010; Britton & Tesser, 1991; Loomis, 2000; Macan, Shahani, Dipboye, & Phillips, 1990). Bliss and Sandiford's study was specifically related to Hispanic students in a community college. These studies concluded that time management skills explain a statistically significant amount of the variance in students' course performance.
In a study about the effects of time management practices on college grades, researchers found that time management was a statistically significant predictor of grade point average (GPA) and accounted for more variance than did SAT scores (Britton & Tesser, 1991). Time management skills correlated with both student academic performance and student stress levels in Macan et al.'s (1990) study. Several researchers have investigated the ability for a student to coordinate their study time (Loomis, 2000; Rabe-Hemp, Woollen, & Humiston, 2009; Syler, Cegielski, Oswald, & Rainer, 2006). All of these studies report that a fundamental component of student success is course preparation and study. A study conducted by Rabe-Hemp et al. found that online students reported more time allocated to class preparation activities than campus-based students. Hurtado, Carter, and Spuler's (1996) survey of first-year Latino college students revealed that time management was one of the top three concerns and among the most difficult aspects regarding adjusting to the first year of college. In a study of Hispanic students, linking study behaviors and student culture in an HSI, Bliss and Sandiford (2010) found that time management was one of the three variables that explained student study behaviors. Management of time for routine and recurring tasks, and management of time for longer-term, specific, non-recurring tasks were specifically related to time management for Hispanic students. For two separate samples of Mexican students in the study, the researchers found that self-efficacy accounted for the largest proportion of variance in study behaviors with managing time related to both long-term and recurring tasks explaining the second largest proportion of variance. They concluded that Hispanic students who demonstrate appropriate long-term and short-term time management skills will likely perform better than those that do not. Given that time management skills seem to be relevant to Hispanic student success, the third hypothesis was phrased:
H3: Student Time Management Skill has a positive and significant effect on the course grade.
Assessing a student's perception of the utility of online learning is related to the concept of perceived usefulness presented in TAM (Davis, 1989) and is an important determinant of computer usage (Saadé & Kira, 2009). This model has been used extensively in the literature to explain information systems use as a result of a set of beliefs about technology (Compeau, Higgins, & Huff, 1999). Dobbs conducted a meta-analysis that concluded that student perceptions significantly explain variances in online courses course grades. Because the literature indicates that the difference in students' attitudes toward online learning was statistically significant between campus-based and online students (Arbaugh et al., 2009; Fortune, Shifflett, & Sibley, 2006; Haigh, 2007), it was included as a control variable in this study.
Stewart, Bachman, and Johnson (2010) considered age, among other student characteristics, in their study of online versus campus-based degree programs and found that students under the age of 30 were more likely to take campus-based courses. Others have found that age was a statistically significant factor in predicting the performance of online students (Allen & Seaman, 2010; Doyle, 2009; Geddes, 2009). The question remained, however, if age played a significant role in the use of e-learning tools. Therefore, age was included as a control variable in this study.
Methods
The population in this study was upper-level students in the Bachelor of Business Administration (BBA) Program in an HSI in South Texas. The students were admitted to the upper division upon completion of 60 hours of required courses with a GPA of 2.5 or higher. The students were sent an invitation to participate in the following courses: Human Resource Management, International Management, and Information Systems in Organizations. Extra points were offered as an incentive to increase their participation in both campus-based and online courses. A questionnaire was delivered in the same platform (Blackboard), and students completed it on their own time. The number of points did not affect the final grade because they were a minimal percentage of the total points tabulated in the courses. A total of 268 students in different course sections in the BBA program agreed to participate in the survey. These participants were used as a matter of convenience and they were not randomly selected. The response rate of 65% was high, but it was not the entire population because students were asked to participate in the survey during their free time. The sample size was large enough to meet the requirements for using structural equation modeling (SEM).
SEM was chosen for this study because it is a graphical and very powerful multivariate analysis technique that uses a path analysis to explain the causal relationship between the variables used in the model. A structural equation model implies a structure of the covariance matrix of the measures. Once the model's parameters have been estimated, the resulting model-implied covariance matrix can then be compared to an empirical or data-based covariance matrix. If the two matrices are consistent with one another, then the structural equation model can be considered a plausible explanation for relations between the measures (Bollen, 1989).
Demographics of the respondents that are of interest are shown in Table 1. There were slightly more female students than male students, 138 to 130. The age of the students divided into typical students under the age of 24 (47%), students between the ages of 24 and 30 (33%), and older students (20%). Two thirds of the students resided within the limits of the city in which the University is based, and the rest were largely from within a 60-mile radius.
Table 1. Respondent demographics
Category |
Frequency |
Percent |
Gender |
|
|
Male |
130 |
48.5 |
Female |
138 |
51.5 |
Age |
|
|
Typical (< 24 years) |
126 |
47.0 |
24-30 years |
88 |
33.0 |
> 30 years |
54 |
20.0 |
Proximity to campus |
|
|
In city |
179 |
66.8 |
Near (within 60 miles) |
82 |
30.6 |
Far (> 60 miles) |
7 |
2.6 |
With regard to the dependent variable, course grade, the distribution shown in Table 2 indicates that 31% of the students earned an A, 41% of them received a B, 21% a C, and 7% of them failed the course. The distribution curve did lean towards the left and indicated the majority of the students earned either an A or a B. This is due to the fact that students that do not have a 2.5 GPA are not admitted to upper division. There is enough variation, however, for an in-depth analysis of the factors that predict course grade.
Table 2. Dependent variable characteristics
Course Grade |
Frequency |
Percent |
A |
83 |
31.0 |
B |
110 |
41.0 |
C |
57 |
21.0 |
D |
11 |
4.0 |
F |
7 |
3.0 |
The questions used in this research were gathered from the literature and adapted for the study. The e-learning tools self-efficacy construct (Saadé & Kira, 2009) was a three-item scale; the ability to work independently construct used a five-item scale, and the time management skills construct used a two-item scale which was adapted from Haigh (2007).
SEM was used to test the relationships in the hypotheses. SEM provides a confirmatory factor analysis, a confirmation test of the scales already provided in the literature, and a measure of internal consistency reliability of each construct assessed. Table 3 lists the scales used for the SEM. The table displays the standardized Cronbach's α values. The α attained for e-learning tools self-efficacy is .80; for ability to work independently, the score is .77, and for time management skills, a score of .70 was achieved.
Table 3. Scales
Construct |
Items |
α |
E-Learning Toolsa Self-Efficacy |
- Before the course started, I thought I could complete the required tasks using e-learning tools even if there was no one around to tell me what to do.
- Before the course started, I thought I could complete the required tasks using e-learning tools even if I had never used a learning tool like it before.
- Before the course started, I thought I could complete the required tasks using e-learning tools.
|
.80 |
Ability to Work Independently |
- I am able to easily access the Internet as needed for my studies.
- I am able to work independently.
- I am self-motivated and self-disciplined.
- I can get motivated if I push myself.
- I can usually figure out what to do in an assignment by reading and following the instructions.
|
.77 |
Time Management Skills
|
- I do not need reminding to get things done on time.
- I am able to get things done on time.
|
.70 |
a"E-learning tools" refers to Blackboard tools such as discussion boards, videos, quizzes, exams, and other online references or activities.
The goodness-of-fit measures for the measurement model shown in Table 4 indicate a good model fit. The practical significance of the model is that it provides empirical support for the constructs of e-learning tools self-efficacy, ability to work independently, and time management skills. The goodness-of-fit indices indicate that the absolute and incremental fit measures for the constructs are considered very good for all three constructs; they are all above the .90 recommended for a very good fit. A score of 1 for the indices denotes a perfect fit. The parsimonious fit measures are lower, however, but fall within the "fair" range. The root mean square error of approximation (RMSEA) for the constructs was well within the recommended level. According to Jöreskog (1990), a normed χ2 ratio of 5 is acceptable, especially when taking the high values of the other indices into consideration. With the normed χ2 scores of 3.230, the model is considered good. A standardized summary of the average covariance residuals (RMSEA) is .091 below the .10 range deemed acceptable (Hair, Anderson, Tatham, & Black, 1998).
Table 4. Goodness-of-fit measures
Category |
Value |
Absolute fit measures |
|
Likelihood ratio χ2 |
203.519 |
Degrees of freedom |
63 |
p |
.000 |
Normed fit index (NFI) |
.982 |
Relative fit index (RFI) |
.974 |
Root mean square error of approximation (RMSEA) |
.091 |
Incremental fit measures |
|
Incremental fit index (IFI) |
.988 |
Tucker–Lewis index (TLI) / non-normed fit index (NNFI) |
.982 |
Comparative fit index (CFI) |
.988 |
Parsimonious fit measures |
|
Parsimonious fit index (PNFI) |
.680 |
Parsimony adjusted CFI (PCFI) |
.684 |
Normed χ2 |
3.230 |
Results
This study yielded empirical evidence to support psychological factors to predict course grades for Hispanic students. Standardized path coefficients with values less than .10 showed a small effect; values in the .30 range indicate a medium effect, while values larger than .50 suggest a large effect (Kline, 1998). As depicted in Figure 1, effect size of e-learning tools self-efficacy – course grade path is small (.13); however it was positive and statistically significant to the highest level with a p value less than .01, thereby, supporting Hypothesis 1. The ability to work independently – course grade path was of the same effect size as the previous path (.13), also positive and statistically significant, thereby providing support for Hypothesis 2. The direct effect of time management skills was significant (.50), positive, and of a large effect size supporting Hypotheses 3. The relationship between course grade and perceived value of the e-learning tool was statistically significant to the .10 level and inverse. This suggests that when students perceive that the online learning environment is inferior to a campus-based environment, their grade suffers. The second control variable is age. The results signal that age and course grade have a negative relationship, that is, the older the student, the higher the likelihood that the course grade will be lower. The relationship was found to be highly statistically significant. The R2 for the model was .32, meaning that the factors in the model can predict 32% of the variance for course grade.
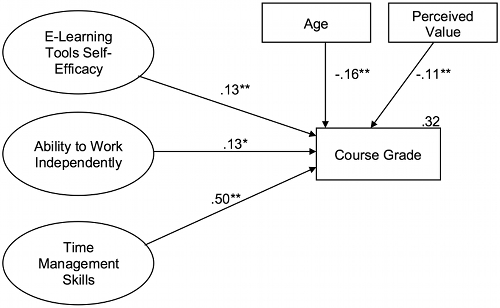
*path significant at p < .05. ** path significant at p < .01.
Figure 1. Estimated model for Course Grade
Discussion
Contributing to the literature on self-efficacy, this study's findings advance the idea that students' level of self-efficacy in the use of computers is related to their ability to achieve a high grade in the course using e-learning tools. E-learning tools self-efficacy, derived from computer self-efficacy, affect the course grade in a manner that is statistically significant and positive. Beyond the fact that students have access to computers and know how to use them for various functions such as e-mail and Facebook is the idea that they can transfer their previous computer experience to the use of the e-learning tools in their classes. This confidence motivates them to use the e-learning tools and benefit from the use of these technologies. "Research suggests that beyond the costs associated with access and a lack of proximity to computers, several social and psychological obstacles interfere with individual motivation to engage with and thus potentially benefit from this new technology" (Stanley, 2003, p. 407). Stanley refers to a person's self-concept as a psychosocial barrier towards increased computer literacy in underserved communities, suggesting that cultural factors are also at play. People's fear and anxiety or lack of self-confidence "prevented them from asking for help and having computer-literate partners only made them more self-conscious about their perceived inabilities" (p. 410).
The second hypothesis in this study was also empirically confirmed, suggesting that an individual's tendency toward working independently is an important predictor of course grade. Padrón et al. (2002) suggest that there is a "pedagogy of poverty" in which a student is not encouraged to think independently but rather is made to passively absorb lessons. "This teacher-directed instructional model emphasizes lecture, drill and practice, remediation, and student seatwork" (p. 9). Instead, Hispanic students should be encouraged to develop higher learning skills and to help themselves learn by questioning, cueing or prompting them to respond. The literature also recommends that more research is needed to determine which instructional methods are effective with Hispanic students. Our study suggests that the use of technology enriched e-learning tools is, in fact, an effective instructional tool for Hispanic students that can be used in both online and campus-based courses, offering similar value. These tools are linguistically and culturally appropriate as the literature indicates (Tharp et al., 2000). E-learning tools provide a medium for students with limited English proficiency to enhance their process of English language assimilation by having asynchronous participation because videos and visual presentations with sound and animation facilitate auditory skills, while digitized books present linking possibilities tied to definitions and pronunciation help. All of these student-centered activities represent a model of instruction that has not been very common for Hispanic students (Padrón et al., 2002).
The third construct supported by the data analysis in this study is developing time management skills of Hispanic students. According to the SEM model, this is the strongest indicator of course grade. A characteristic typical of Hispanic students is that they are likely to be commuter students; they tend to be older, and to have full-time jobs. This places greater time pressure on the students than typically demanded of younger full-time students because of the need to juggle school with family and work responsibilities. Stewart et al.'s (2010) investigation revealed that non-traditional students and traditional students in online and campus-based classes had similar motivation to complete their degrees with one exception. Non-traditional students placed greater emphasis on course schedules, time constraints, and scheduling concerns due to work and home responsibilities.
Conclusion
Lower enrollment and completion rates in 2-year and 4-year colleges by Hispanics represent the fastest growing minority group in the U.S., and makes researching the topic of Hispanic student success in higher education critical. In the last few years, a large percentage of the labor market in the U.S. will be Hispanic, and Hispanics will drive the growth of businesses and the economy by greater proportions, producing what could be called a Hispanic educational crisis. Now, more than ever, is the time to start to seriously increase the number of Hispanics completing university degrees and learning more about what can be done in the classroom to promote Hispanic student academic performance. This need will only increase as the population increases and should create a sense of urgency among researchers to learn more about the factors that contribute to Hispanic student academic performance. Understanding characteristics, such as e-learning tools self-efficacy, student ability to work independently, and time management skills that underlie Hispanic student academic performance can help HSIs as well as individual instructors positively affect course grades and, ultimately, degree completion. Because these three constructs are related to motivation and persistence, additional insights related to Hispanic student academic performance may be gleaned from studying motivation and persistence, as well. Additionally, how the constructs in this study relate to course design or how the design may influence the time management skills of Hispanic students may have implications for future studies. Continued research is encouraged with this population, not just at the university level, but also at the classroom level to address an issue that has far-reaching consequences.
Whether the classroom is physical (campus-based) or virtual (online) in nature, this study has shown that attention to three key elements can improve course grades for Hispanic students. Professors in any classroom setting, but particularly in one with Hispanic students, will be able to improve course grades by incorporating e-learning tools into the course design. Hispanic students, because of language and cultural barriers, will greatly benefit from course design enriched with e-learning tools, because these tools will result in improved student self-efficacy. In addition, a course design with opportunities for students to operate independently within a set timeframe will develop both a student's ability to work independently and the student's time management skills. Incorporating activities and elements that reinforce any or all of these three key areas will improve student performance. Improved performance in the classroom will lead to better retention rates for Hispanic students.
References
Allen, I. E., & Seaman, J. (2010). Learning on demand: Online education in the United States, 2009. Babson Park, MA: Babson Survey Research Group. Retrieved from http://www.sloanconsortium.org/publications/survey/pdf/learningondemand.pdf
Alon, S., Domina, T., & Tienda, M. (2010). Stymied mobility or temporary lull? The puzzle of lagging Hispanic college degree attainment. Social Forces, 88(4), 1807-1832. doi:10.1353/sof.2010.0017
Arbaugh, J. B. (2005). Is there an optimal design for on-line MBA courses? Academy of Management Learning & Education, 4(2), 135-149. doi:10.5465/AMLE.2005.17268561
Arbaugh, J. B., Godfrey, M. R., Johnson, M., Pollack, B. L., Niendorf, B., & Wresch, W. (2009). Research in online and blended learning in the business disciplines: Key findings and possible future directions. The Internet and Higher Education, 12(2), 71-87. doi:10.1016/j.iheduc.2009.06.006
Artino, A. R., Jr. (2009). Online learning: Are subjective perceptions of instructional context related to academic success? The Internet and Higher Education, 12(3-4), 117-125. doi:10.1016/j.iheduc.2009.07.003
Attewell. P. (2001). The first and second digital divides. Sociology of Education, 74(3), 252-259. doi:10.2307/2673277
Bekele, T. A., & Menchaca, M. P. (2008). Research on Internet-supported learning: A review. Quarterly Review of Distance Education, 9(4), 373-405.
Bliss, L. B., & Sandiford, J. R. (2004). Linking study behaviors and student culture to academic success among Hispanic students. Community College Journal of Research and Practice, 28(3), 281-295. doi:10.1080/10668920490256462
Bollen, K. A. (1989). Structural equations with latent variables. New York, NY: Wiley.
Britton, B. K., & Tesser, A. (1991). Effects of time-management practices on college grades. Journal of Educational Psychology, 83(3), 405-410. doi:10.1037/0022-0663.83.3.405
Candy, P. C. (1991). Self-direction for lifelong learning: A comprehensive guide to theory and practice. San Francisco, CA: Jossey-Bass.
Compeau, D. R., & Higgins, C. A. (1995). Computer self-efficacy: Development of a measure and initial test. MIS Quarterly, 19(2), 189-211. doi:10.2307/249688
Compeau, D. R., Higgins, C. A., & Huff, S. (1999). Social cognitive theory and individual reactions to computing technology: A longitudinal study. MIS Quarterly, 23(2), 145-158. doi:10.2307/249749
Davis, F. D. (1989). Perceived usefulness, perceived ease of use, and user acceptance of information technology. MIS Quarterly, 13(3), 319-340. doi:10.2307/249008
DiMaggio, P., Hargittai, E., Neuman, W. R., & Robinson, J. P. (2001). Social implications of the Internet. Annual Review of Sociology, 27, 307-336. doi:10.1146/annurev.soc.27.1.307
Doyle, W. R. (2009). Online education: The revolution that wasn't. Change: The Magazine of Higher Learning, 41(3), 56-58. doi:10.3200/CHNG.41.3.56-58
Enoch, Y., & Soker, Z. (2006). Age, gender, ethnicity and the digital divide: University students' use of web-based instruction. Open Learning, 21(2), 99-110. doi:10.1080/02680510600713045
Fortune, M. F., Shifflett, B., & Sibley, R. E. (2006). A comparison of online (high tech) and traditional (high touch) learning in business communication courses in Silicon Valley. Journal of Education for Business, 81(4), 210-214. doi:10.3200/JOEB.81.4.210-214
Galy, E., Downey, C. & Johnson, J. (2011). The effect of using e-learning tools in online and campus-based classrooms on student performance. Journal of Information Technology Education, 10, 209-230. Retrieved from http://www.jite.org/documents/Vol10/JITEv10p209-230Galy975.pdf
Gates, A. Q. (2010). The role of Hispanic-serving institutions in contributing to an educated work force. Communications of the ACM, 53(12), 31-33. doi:10.1145/1859204.1859217
Geddes, D. (2009). How am I doing? Exploring on-line gradebook monitoring as a self-regulated learning practice that impacts academic achievement. Academy of Management Learning & Education, 8(4), 494-510. doi:10.5465/AMLE.2009.47785469
Haberman, M. (1991). The pedagogy of poverty versus good teaching. Phi Delta Kappan, 73(4), 290-294.
Haigh, M. (2007). Divided by a common degree program? Profiling online and face-to-face information science students. Education for Information, 25(2), 93-110.
Hair, J. F., Jr., Anderson, R. E., Tatham, R. L., & Black, W. C. (1998). Multivariate data analysis (5th ed.). Upper Saddle River, NJ: Prentice Hall.
Humes, K. R., Jones, N. A., & Ramirez, R. R. (2011). Overview of race and Hispanic origin: 2010. (2010 Census Briefs, Report No.C2010BR-02). Washington, DC: U.S. Census Bureau. Retrieved from http://www.census.gov/prod/cen2010/briefs/c2010br-02.pdf
Hung, M.-L., Chou, C., Chen, C.-H., & Own, Z.-Y. (2010). Learner readiness for online learning: Scale development and student perceptions. Computers & Education, 55(3), 1080-1090. doi:10.1016/j.compedu.2010.05.004
Hurtado, S., Carter, D. F., & Spuler, A. (1996). Latino student transition to college: Assessing difficulties and factors in successful college adjustment. Research in Higher Education, 37(2), 135-157. doi:10.1007/BF01730113
Jöreskog, K. G., (1990). New developments in LISREL: Analysis of ordinal variables using polychoric correlations and weighted least squares. Quality and Quantity, 24(4), 387-404. doi:10.1007/BF00152012
Katz, J. E., Rice, R. E., & Aspden, P. (2001). The Internet, 1995-2000: Access, civic involvement, and social interaction. American Behavioral Scientist, 45(3), 405-419. doi:10.1177/0002764201045003004
Kilic-Cakmak, E., Karatas, S., & Ocak, M. A. (2009). An analysis of factors affecting community college students' expectations on e-learning. Quarterly Review of Distance Education, 10(4), 351-361.
Kline, R. B. (1998). Principles and practice of structural equation modeling. New York, NY: Guilford Press.
Knowles, M. S. (1975). Self-directed learning: A guide for learners and teachers. New York, NY: Association Press.
Knowles, M. S. (1980). The modern practice of adult education: From pedagogy to andragogy (Rev. & expanded ed.). New York, NY: Cambridge.
Loomis, K. D. (2000). Learning styles and asynchronous learning: Comparing the LASSI model to class performance. Journal of Asynchronous Learning Networks, 4(1), 23-32. Retrieved from http://www.sloanconsortium.org/sites/default/files/v4n1_loomis_1.pdf
Lou, Y., Bernard, R. M., & Abrami, P. C. (2006). Media and pedagogy in undergraduate distance education: A theory-based meta-analysis of empirical literature. Educational Technology Research & Development, 54(2), 141-176. doi:10.1007/s11423-006-8252-x
Lynch, M., & Engle, J. (2010). Big gaps, small gaps: Some colleges and universities do better than others in graduating Hispanic students. Washington, DC: The Education Trust. Retrieved from http://www.edtrust.org/sites/edtrust.org/files/publications/files/CRO%20Brief-Hispanic.pdf
Macan, T. H., Shahani, C., Dipboye, R. L., & Phillips, A. P. (1990). College students' time management: Correlations with academic performance and stress. Journal of Educational Psychology, 82(4), 760-768. doi:10.1037/0022-0663.82.4.760
Maceli, K. M., & Box, T. M. (2010). Hispanic students' success in business education. Academy of Educational Leadership Journal, 14(2), 119-134.
Manochehri, N.-N., & Sharif, K. (2010). A model-based investigation of learner attitude towards recently introduced classroom technology. Journal of Information Technology Education, 9, 31-52. Retrieved from http://www.jite.org/documents/Vol9/JITEv9p031-052Manochehri744.pdf
Martins, L. L., & Kellermanns, F. W. (2004). A model of business school students' acceptance of a web-based course management system. Academy of Management Learning & Education, 3(1), 7-26. doi:10.5465/AMLE.2004.12436815
Mykytyn, K., Pearson, A., Paul, S., & Mykytyn, P. P., Jr. (2008). The use of problem-based learning to enhance MIS education. Decision Sciences Journal of Innovative Education, 6(1), 89-113. doi:10.1111/j.1540-4609.2007.00160.x
Padrón, Y. N., Waxman, H. C., & Rivera, H. H. (2002). Educating Hispanic students: Obstacles and avenues to improved academic achievement. Santa Cruz, CA: Center for Research on Education, Diversity & Excellence. Retrieved from http://www.cal.org/crede/pdfs/epr8.pdf
Puzziferro, M. (2008). Online technologies self-efficacy and self-regulated learning as predictors of final grade and satisfaction in college-level online courses. American Journal of Distance Education, 22(2), 72-89. doi:10.1080/08923640802039024
Rabe-Hemp, C., Woollen, S., & Humiston, G. S. (2009). A comparative analysis of student engagement, learning, and satisfaction in lecture hall and online learning settings. Quarterly Review of Distance Education, 10(2), 207-218.
Saadé, R. G., & Kira, D. (2009). Computer anxiety in e-learning: The effect of computer self-efficacy. Journal of Information Technology Education, 8, 177-191. Retrieved from http://www.jite.org/documents/Vol8/JITEv8p177-191Saade724.pdf
Seok, S., DaCosta, B., Kinsell, C., & Tung, C. K. (2010). Comparison of instructors' and students' perceptions of the effectiveness of online courses. Quarterly Review of Distance Education, 11(1), 25-36.
Shinkareva, O. N., & Benson, A. D. (2007). The relationship between adult students' instructional technology competency and self-directed learning ability in an online course. Human Resource Development International, 10(4), 417-435. doi:10.1080/13678860701723737
Shroff, R. H., & Vogel, D. R. (2009). Assessing the factors deemed to support individual student intrinsic motivation in technology supported online and face-to-face discussions. Journal of Information Technology Education, 8, 59-85. Retrieved from http://www.jite.org/documents/Vol8/JITEv8p059-085Shroff416.pdf
Simmering, M. J., Posey, C., & Piccoli, G. (2009). Computer self-efficacy and motivation to learn in a self-directed online course. Decision Sciences Journal of Innovative Education, 7(1), 99-121. doi:10.1111/j.1540-4609.2008.00207.x
Slate, J. R., Manuel, M., & Brinson, K. H., Jr. (2002). The "digital divide": Hispanic college students' views of educational uses of the Internet. Assessment & Evaluation in Higher Education, 27(1), 75-93. doi:10.1080/02602930120105081
Smart, K. L., & Cappel, J. J. (2006). Students' perceptions of online learning: A comparative study. Journal of Information Technology Education, 5, 201-219. Retrieved from http://www.jite.org/documents/Vol5/v5p201-219Smart54.pdf
Smith, P. J., Murphy, K. L., & Mahoney, S. E. (2003). Towards identifying factors underlying readiness for online learning: An exploratory study. Distance Education, 24(1), 57-67. doi:10.1080/01587910303043
Somenarain, L., Akkaraju, S., & Gharbaran, R. (2010). Student perceptions and learning outcomes in asynchronous and synchronous online learning environments in a biology course. MERLOT Journal of Online Learning and Teaching, 6(2), 353-356. Retrieved from https://jolt.merlot.org/vol6no2/somenarain_0610.htm
Stanley, L. D. (2003). Beyond access: Psychosocial barriers to computer literacy. The Information Society, 19(5), 407-416. doi:10.1080/715720560
Stephen, V. P., Varble, M. E., & Taitt, H. (1993). Instructional strategies for minority youth. The Clearing House, 67(2), 116-120. doi:10.1080/00098655.1993.9956037
Stewart, C., Bachman, C., & Johnson, R. (2010). Students' characteristics and motivation orientations for online and traditional degree programs. MERLOT Journal of Online Learning and Teaching, 6(2), 367-379. Retrieved from https://jolt.merlot.org/vol6no2/stewart_0610.htm
Syler, R. A., Cegielski, C. G., Oswald, S. L., & Rainer, R. K., Jr. (2006). Examining drivers of course performance: An exploratory examination of an introductory CIS applications course. Decision Sciences Journal of Innovative Education, 4(1), 51-65. doi:10.1111/j.1540-4609.2006.00101.x
Telemundo launches 2010 census campaign, "hazte contar!". (2009). Retrieved from http://www.businesswire.com/news/home/20090203006064/en/Telemundo-Launches-2010-Census-Campaign-%E2%80%9CHazte-Contar%21%E2%80%9D
Tharp, R. G., Estrada, P., Dalton, S. S., & Yamauchi, L. A. (2000). Teaching transformed: Achieving excellence, fairness, inclusion, and harmony. Boulder, CO: Westview Press.
Unal, Z., & Unal, A. (2011). Evaluating and comparing the usability of web-based course management systems. Journal of Information Technology Education, 10, 19-38. Retrieved from http://www.jite.org/documents/Vol10/JITEv10p019-036Unal904.pdf
U.S. Census Bureau. (2012). Educational attainment in the United States: 2010 – detailed tables. Retrieved from http://www.census.gov/hhes/socdemo/education/data/cps/2010/tables.html
U.S. Department of Education. (2011). Moving America forward: President Obama's agenda for the Latino community. Washington, DC: Author. Retrieved from http://www2.ed.gov/about/inits/list/hispanic-initiative/winning-the-future.pdf
U.S. Department of Education & White House Initiative on Educational Excellence for Hispanics. (2011). Winning the future: Improving education for the Latino community. Washington, DC: Authors. Retrieved from http://www.whitehouse.gov/sites/default/files/rss_viewer/WinningTheFutureImprovingLatinoEducation.pdf
U.S. Department of Labor. (2012). The Latino labor force at a glance. Washington, DC: Author. Retrieved from http://www.dol.gov/_sec/media/reports/HispanicLaborForce/HispanicLaborForce.pdf
Vasquez, J. A. (1990). Teaching to the distinctive traits of minority students. The Clearing House, 63(7), 299-304. doi:10.1080/00098655.1990.10114113
Welsh, E. T, Wanberg, C. R., Brown, K. G., & Simmering, M. J. (2003). E-learning: Emerging uses, empirical results and future directions. International Journal of Training and Development, 7(4), 245-258. doi:10.1046/j.1360-3736.2003.00184.x