Technology Acceptance and Performance in Online Learning Environments: Impact of Self-Regulation
|
Filiz Tabak
Professor
Department of Management
Towson University
Towson, MD 21252 USA
ftabak@towson.edu
Nhung T. Nguyen
Associate Professor
Department of Management
Towson University
Towson, MD 21252 USA
nnguyen@towson.edu
Abstract
This paper proposes a conceptual model that integrates the technology acceptance model with the self-regulation concept from social cognitive theory. The model explains the antecedents to perceived ease of use and perceived usefulness of online learning systems by learner self-regulatory processes and behaviors, personality differences, and extrinsic factors such as technical support, technology training, and equipment accessibility. Personality variables that are proposed to affect self-regulation and learners' perceived ease of use and perceived usefulness are conscientiousness, openness to experience, general self-efficacy, and risk propensity. Implications for future research and practice are discussed.
Keywords: technology acceptance, performance, self-regulation, personality, perceived ease of use, perceived usefulness |
Introduction
Despite the rapid increase in the number of college courses offered either fully online (e.g., Dillon, 2008; Golden, 2006) or in a blended (hybrid) format where at least 50% of the course is delivered online (e.g., Ross & Rosenbloom, 2011; Rossett, 2006), research on what determines student performance in such environments is still not fully understood. How are effective online learning environments designed? What type of student is more likely to succeed in online or hybrid courses? Are there particular student characteristics that would differentially impact the process of learning and course success for traditional, face-to-face courses versus online courses? These are a few of the questions that need to be addressed by future research. This paper proposes a model that integrates the technology acceptance model (TAM) originally proposed by Davis (1989) with the self-regulation concept from social cognitive theory (Bandura, 1986) to explicate the processes and factors that contribute to effective online learning and online student course performance. Further, it uses the five-factor model of personality (Barrick & Mount, 2005) to explain the intrinsic factors that contribute to self-regulation and technology acceptance.
TAM is a framework of acceptance of new technologies (Martins & Kellermanns, 2004) explaining user acceptance as a function of perceived ease of use and perceived usefulness of the technology. Perceived ease of use is defined as how easy the user believes the technology is to use (Park, 2009; Venkatesh & Davis, 2000a). Perceived usefulness is defined as the user's belief that the technology will improve his or her performance (Lee & Lee, 2008; Venkatesh & Davis, 2000b). The model proposes that perceived ease of use and usefulness of new technology affects attitudes toward the technology, which is an antecedent to behavioral intentions to use it. This paper applies the TAM to online learning and explains the antecedents to perceived ease of use and usefulness of new technologies by learners' self-regulatory processes and behaviors (Sitzmann & Ely, 2010) and personality differences (Barrick & Mount, 2005).
Self-regulation is a cyclical process involving three phases: (1) the forethought phase where planning and goal setting take place; (2) the performance phase where learners focus on self-instruction and monitoring; and (3) the self-reflection phase where learners evaluate their performance and make causal attributions (Williams & Hellman, 2004; Zimmerman, 2008). Skillful self-regulating learners set specific and proximal goals, keep a focus on learning rather than performing, and have high task-specific self-efficacy as well as a tendency to self-instruct (Zimmerman, 1998). These are critical qualities in an online learning environment as well.
Regarding the conceptualization of personality differences, the impact of the five-factor model is widely accepted (e.g., Barrick & Mount, 2005; Chamorro-Premuzic & Furnham, 2003; Judge & Ilies, 2002). This paper incorporates conscientiousness and openness to experience from the five-factor model as well as general self-efficacy and risk propensity as intrinsic factors that affect how learners perceive the level of easiness and usefulness of online learning, and consequently their performance in online courses. Even though there seems to exist plenty of research targeted at understanding which dispositional traits actually impact academic performance, more research is clearly needed to find out how these traits exert their influence. The "how" question can be addressed by investigating self-regulation as a mediating mechanism between personality traits and academic performance in online environments. Hence, the purpose of this paper is to develop a conceptual model that explains student attitudes toward online learning and performance in online learning environments, and to understand factors that lead to effective online course management systems.
Theoretical Background and Propositions
Self-Regulation
Self-regulation is a concept central to social cognitive theory. It specifically refers to the processes of setting and maintaining goals (Lord, Diefendorff, Schmidt, & Hall, 2010). Self-regulation involves individuals' efforts to compare their progress toward their set goals and to make changes to modify their behaviors if there is a discrepancy between the goals and the current state of progress (Karoly, 1993). Accordingly, Lord et al. specify a model of a negative feedback loop (as opposed to a positive feedback loop where individuals set goals higher than current performance) where individuals' perception of their current performance (behavior) serves as an input to compare to the standard (i.e., the goal), and the outcome of which is to either change the behavior or the cognition.
In general, individuals employ a three stage cognitive process of goal attainment, mainly the forethought phase (including task analysis and self-motivation beliefs), the performance phase (including self-control and self-observation), and the self-reflection phase (including self-judgment and self-reaction) (Zimmerman, 2008). The forethought phase focuses on goal setting, strategic planning, self-efficacy, outcome expectations, goal orientation, and task interest/value. The performance phase involves self-instruction, attention focusing, cognitive monitoring, and task strategies, while the self-reflection phase includes self-evaluation as well as self-satisfaction and affect. These three phases are assumed to be causally related in a circular fashion, in that the forethought phase predicts the performance phase, which predicts the self-reflection phase. Self-reflection outcomes are used to change the forethought phase processes like planning. Growing empirical support for this model seems to exist (Sitzmann & Ely, 2010; Zimmerman & Bandura, 1994; Zimmerman & Kitsantas, 1997). The model has been applied to online learning as well. For example, Williams and Hellman (2004) provided evidence that first-generation college students seem to have significantly lower levels of self-regulation for online learning than second-generation college students.
Self-regulated learning. Research on self-regulation of academic learning and academic performance in particular has focused on proactive, self-directed processes and beliefs that students employ to monitor their academic performance. Research on students' self-regulatory strategies has yielded evidence that such strategies are significantly associated with course performance (e.g., Pintrich, 2000; Pintrich, Smith, Garcia, & McKeachie, 1993; Tsai, Tung, & Laffey, 2008). Students who are good at self-regulation seem to also set specific proximal goals as opposed to general, distal ones, to be focused on learning rather than just performing in the course as well as on self-instruction, self-monitoring, and self-evaluation, according to Zimmerman (1998). Zimmerman further notes that learners with high self-regulatory skills are also high in self-efficacy, more adaptive to contextual cues, and are intrinsically motivated by and interested in the subject. Research has shown that self-regulated learners set, monitor, and evaluate progress toward their goals (Nenniger, 2005; Schunk, 1996). Academic self-regulation seems to affect academic success positively via increased focus and attention during instruction (Boekaerts & Corno, 2005; Boekaerts, Pintrich, & Zeidner, 2000; Pintrich & Schrauben, 1992; Williams & Hellman, 1998, 2004). Even though one can say that collectively this line of research points to a significant and positive relationship between academic self-regulation and academic performance, there is little research looking at the processes of how self-regulated learning leads to higher online learning and online course performance. Since online learning allows the learner flexibility and choice in how, when, and where to learn, individuals who are more skillful in self-regulation should perform higher than those who are not as skillful in online learning environments.
Self-regulation in learning happens in phases (Pintrich, 2000; Winne & Hadwin, 1998; Zimmerman, 2000). In the forethought phase, learners think about and develop strategies and goals most suitable for their learning, how interesting and valuable the task is to them, and their ability to complete the task successfully. This phase further involves the learner's expectations about the outcomes and the amount of time and effort needed to reach these outcomes. The performance phase follows the forethought phase where learners focus attention to the task and engage in self-instruction and self-observation of their progress. The third phase of the model is self-reflection where learners evaluate and make causal attributions about their performance and consequently develop goal-congruent or goal-incongruent affect and satisfaction with their performance. Recently self-regulated learning researchers have argued that internal (e.g., cognition, motivation, affect) and external (e.g., exams, writing, social interaction) factors work together to produce context-specific self-regulated learning (Cho & Jonassen, 2009) and that self-regulatory efficacy affects academic performance (Lee & Lee, 2008). In parallel, Zhang and Goel (2011) have shown that a positive external environment and a strong internal drive for e-learning is important for successful e-learning outcomes. The model presented in this paper focuses on intrinsic and extrinsic factors specific to learning in online environments. The proposed model is depicted in Figure 1.
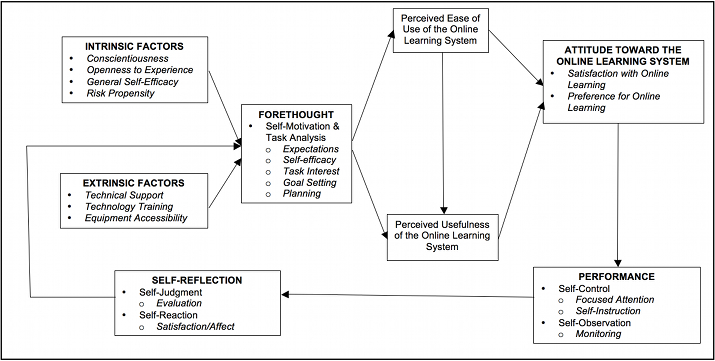
Figure 1. A conceptual model of self-regulation in online learning environments
Technology Acceptance Model
Building on Fishbein and Ajzen's (1975) theory of reasoned action, Davis (1989) developed the TAM to explain and predict adoption and use of technology. This well-accepted model has been used in management education research before (Arbaugh, 2000; Martins & Kellermanns, 2004) and provides a theoretically grounded conceptualization of user adoption and acceptance of new technology. Accordingly, perceived usefulness and perceived ease of use are the two main determinants of adoption of new technology. Perceived usefulness refers to the extent that a learner believes the technology will improve their performance and thus represents extrinsic motivation to use the technology (Arbaugh, 2000; Selim, 2003). In an online learning context, perceived usefulness involves the learner's belief that the online learning environment will enhance performance in the course (Lee, Cheung, & Chen, 2005). Perceived ease of use refers to the extent that a learner believes that the use of the particular technology will be relatively easy and thus represents intrinsic motivation to use the technology (Park, 2009). In an online learning context, it involves the learner's belief that the online learning system will be easy to use. Adoption or use of technology is interconnected to a positive attitude toward the system and the behavioral intention to use the system (Martins & Kellermanns, 2004; Park, 2009; Tsai, Tung, & Laffey, 2013). Research has shown that the greater the perceived usefulness of new technology and perceived ease of use, the more positive the attitude will be toward the new technology, leading to greater intention to use the system (e.g., Lee et al., 2005; Lin & Lu, 2000; Martins & Kellermanns, 2004; Park, 2009; Venkatesh & Davis, 2000b). Even though researchers have used varying definitions and measurements of attitude, the most commonly used one is satisfaction with the system (Compeau, Higgins, & Huff, 1999; Martins & Kellermanns, 2004). In an online learning context, Lee (2008) found that perceived usefulness and perceived ease of use are positively related to behavioral intention to use the new system but did not measure attitude toward online learning.
Further, TAM researchers have studied contextual factors that affect perceived ease of use and perceived usefulness of a particular new technology. For example, Martins and Kellermanns (2004) integrated the literature on change implementation to the TAM, and explicated motivators and enablers that impact perceived usefulness and ease of use as perceived incentive to use new technology, perceived encouragement, awareness of capabilities of new technology, access to the system, availability of technical support, prior experience, as well as self-efficacy. Lee (2008) delineated intra-organizational and extra-organizational factors such as internal and external computing support and training and equipment accessibility. Yet this line of research has not extended to individual characteristics or personality factors as antecedents of technology acceptance and adoption.
Intrinsic and Extrinsic Factors
Most self-regulated learning models incorporate intrinsic and extrinsic factors to explain the process of self-regulation in a specific context (e.g., Cho & Jonassen, 2009; Muis, 2007; Tsai et al., 2013). Research has identified intrinsic factors as affect/motivation and cognition, while extrinsic factors were described as typical course activities such as writing papers, exams, and social interaction (Cho & Jonassen, 2009; Muis, 2007; Winne & Hadwin, 1998; Zimmerman, 2000). In parallel, the TAM researchers have also identified various internal and external factors that influence perceived usefulness and perceived ease of use of new technology (e.g., Zhang, Zhao, & Tan, 2008). Yet, few, if any, studies have adopted the TAM to explain satisfaction with and use of online learning environments (Lee, 2008). And no studies, to the knowledge of the authors, have explored the impact of stable individual (intrinsic) characteristics such as personality on perceived usefulness or perceived ease of use of online courses and online learning. This model explicates intrinsic (conscientiousness, openness to experience, risk propensity, and self-regulation) and extrinsic (perceived computing support availability, technology training, and equipment accessibility) factors that are likely to affect how usefulness and ease of use is perceived in online learning environments.
Among the various personality factor models that exist in literature, the most notable framework is the five-factor model, also known as the Big Five model (Just, 2011). An impressive body of research attests to the influence of the five-factor model as a significant area of investigation (e.g., Barrick & Mount, 2005; Tabak, Nguyen, Basuray, & Darrow, 2009). Researchers have studied the relationships between factors of the Big Five model and a variety of outcome variables (e.g., Judge, Jackson, Shaw, Scott, & Rich, 2007). Among them, research support for agreeableness and its relationship to performance is mixed at best (Judge & Ilies, 2002; Kamdar & Van Dyne, 2007). Agreeableness may be an influential factor in managing personal relationships and in group contexts (Small & Diefendorff, 2006); yet there is no clear evidence that it may have an impact on adopting new technology, online learning, or self-regulation.
While extraversion was positively related to leadership emergence in past empirical research (Judge, Bono, Ilies, & Gerhardt, 2002), its association with task performance is positive but small (Judge & Erez, 2007), and again, there is no evidence that extraversion may be influential in online learning, academic performance, or new technology adoption. Emotional stability refers to being calm, secure, and relaxed. There seems to be no clear evidence, empirical or otherwise, that this personality factor should predict self-motivation and task analysis or impact perceived ease of use or perceived usefulness of new technology. Hence, the authors chose to focus on conscientiousness and openness to experience as the factors of the Big Five model that have the potential to influence perceptions of ease of use and usefulness of new technology and online learning, in particular. Below, the authors review research on these two Big Five model factors and their proposed impact on self-regulation and TAM.
Conscientiousness. Conscientiousness refers to how dependable and responsible an individual is in different situations (Digman, 1990). Researchers have investigated the personality dimensions of the five-factor model in training and learning contexts. Chamorro-Premuzic and Furnham (2003), for example, in a longitudinal study, found that conscientiousness improves academic achievement. Lee and Klein (2002) also showed that conscientiousness is related to academic performance, indicating that individuals who are responsible and persistent tend to also be more committed to learning goals and have higher levels of success in measures of learning outcomes. Klein and Lee (2006) found that learning goal orientation was also positively related to conscientiousness. Tabak et al. (2009) provide empirical support that conscientiousness is a significant predictor of course performance. Collectively, this line of research points to evidence of a positive relationship between conscientiousness and academic achievement.
Yet, what are the processes that link distal personality traits like conscientiousness to outcome variables such as performance? In online learning environments, it is particularly important to be able to plan and monitor progress independently. It is also important to be able to regulate effort to achieve a desired level of performance. To that end, self-regulation is a significant concept central to online learning. The first phase of self-regulation, called the forethought phase (Zimmerman & Campillo, 2003), involves task analysis, in particular goal setting and strategic planning, and the self-motivation beliefs of the learner, such as outcome expectations and interest in and perceived value of the task. Individuals who are high in conscientiousness are more likely to make long-term plans, set goals, and have positive outcome expectations since one of the main sets of traits that distinguishes them is their organization, responsibility, and work ethic.
Individuals high in conscientiousness are organized, and usually are planners with a clear goal-orientation. Goal oriented behavior leads to adoption of strategies and a can-do attitude, which predict self-motivation and higher levels of performance (Gerhardt, Rode, & Peterson, 2007). Planning, organizing, and goal setting are indicators of self-motivation that facilitate learners' perceptions of ease of use and usefulness of an online learning system. Individuals who engage in planning and self-motivation will tend to perceive the new technology, change, or challenge as more achievable, more manageable, and hence easier to use and more useful for their learning performance than those who are not. For this reason, while conscientiousness as a personality trait is a distal variable, it manifests itself in task analysis, goal setting, and planning behaviors. This shapes individuals' perceptions that technology is manageable and relatively easy to use, and is instrumental in reinforcing the learning outcomes (and is therefore useful).
Proposition 1. Conscientiousness will be positively related to perceived ease of use and usefulness of online learning environments, and these relationships will be mediated by the self-regulation phase of task analysis and self-motivation beliefs.
Openness to experience. Individuals who are open to intellectual challenges and gaining new experiences will tend to also be more receptive to learning new technology. Openness to experience entails intellectualism and imagination. Individuals who score high on openness to experience are curious, creative, behaviorally flexible, and broad minded (McCrae, 1996). Empirically, openness to experience is associated with academic performance (Blickle, 1996). Openness further relates to artistic creativity, divergent thinking, intelligence, and being open to learning new materials (Feist, 1998; Holland, Dollinger, Holland, & MacDonald, 1995; Klein & Lee, 2006; McCrae, 1987, 1993-1994). Hence, it is predicted here that openness to experience will be positively related to perceived ease of use of online learning environments because online learning systems indicate a change from the traditional ways of content delivery such as face-to-face instruction.
It is also predicted that this relationship will be mediated by task analysis processes such as goal setting, planning, and belief in oneself to achieve in a new technology environment, since individuals open to new experiences will be more likely to believe in their ability to perform in a new technology environment and hence prepare and plan for their expected performance. As a personality dimension encompassing a variety of traits such as intellectual curiosity and flexibility, openness to experience is likely to have a positive impact on learning through self-efficacy. Individuals who are open to new experiences will likely have built experiences in attempting tasks in new areas and therefore believe that they have the ability to go through uncharted waters. They will tend not to be intimidated by the novelty of ideas or concepts as prior experiences with uncertainty have given them the basis for believing in their capacity to handle the uncertainties inherent in learning something new. Individuals high in openness to experience will tend to view change as worthwhile (Klein & Lee, 2006), and so they are more likely to perceive online learning systems as useful than those who are low in this dimension.
Proposition 2. Openness to experience will be positively related to perceived ease of use and usefulness of online learning environments, and these relationships will be mediated by the self-regulation phase of task analysis and self-motivation beliefs.
General self-efficacy. This stable personality characteristic refers to one's belief in his or her ability to perform across a variety of different situations (Chen, Gully, & Eden, 2001; Judge, Erez, & Bono, 1998). It is different from task-specific self-efficacy, which indicates one's belief in his or her ability to perform a specific task (Wood & Bandura, 1989). Accordingly, general self-efficacy is a motivational trait and relates strongly to self-esteem, locus of control, and emotional stability (Judge, Bono, & Locke, 2000). There is also evidence linking general self-efficacy with learning goal orientation (Chen, Gully, Whiteman, & Kilcullen, 2000), and so it is plausible to expect that individuals high in general self-efficacy are more likely to plan and set goals, as well as have more positive outcome expectations and a goal orientation.
In a sample of 628 students, Park (2009) found that e-learning self-efficacy predicted both perceived usefulness and perceived ease of use of e-learning systems in a university. Individuals who are high in self-efficacy are also more likely to perceive new tasks as easier to accomplish than those who are not because this is a learned effect, in that general self-efficacy influences task-specific self-efficacy across tasks and situations (Eden, 2001). In parallel, Grandon, Alshare, and Kwun (2005) assert that e-learning self-efficacy affects behavioral intention through perceived ease of use. The authors of the present paper propose that self-efficacy will lead to perceptions of ease of use of specific online learning systems. As Chen et al. (2001) point out, the tendency to believe in one's general ability to achieve "spills over" to specific tasks and situations. As individuals become interested in specific tasks and are more self-motivated, their perceptions of usefulness of new technology will also tend to strengthen because usefulness indicates utility values of using new technology in enhancing performance. Substantial benefits exist for students using learning systems such as accessing and downloading lecture notes, presentation videos, interactive puzzles, study tools, as well as group decision making tools such as chat rooms and wikis. It is predicted that self-regulation processes of task analysis and self-motivation beliefs will mediate the relationship between general self-efficacy and perceived ease of use and usefulness of online learning environments.
Proposition 3. General self-efficacy will be positively related to perceived ease of use and usefulness of online learning environments, and these relationships will be mediated by the self-regulation phase of task analysis and self-motivation beliefs.
Risk propensity. Sitkin and Pablo (1992) define risk propensity as "the tendency of a decision maker either to take or to avoid risks" (p. 12). Risk propensity is a stable, generalized personality trait indicating one's willingness to take risk (Das & Teng, 2004). Risk propensity influences an individual's assessment of risk at any particular situation (Brockhaus, 1980). There is also empirical evidence that as risk propensity increases, perception of risk decreases (Sitkin & Weingart, 1995). Das and Teng assert that a linear relationship exists among risk propensity, risk perception, and risk taking, in that high risk propensity leads to low risk perception, which leads to high risk taking. As a result, when individuals are faced with new technology or experiences, those with high risk propensity, as opposed to ones with low risk propensity, can be expected to perceive less risk and have more positive outcome expectations. As part of the self-regulatory phase of forethought, it is more likely for those with high risk propensity to make strategic long term plans inherently involving more risk and to plan and set goals when faced with uncharted waters or new technology in online learning environments.
New technology implies change. TAM is a valuable framework that explains why a user accepts or rejects technology or change. Risk propensity as a stable personality characteristic implies a tendency to be open to change and not be intimidated with the uncertainty of new technology. As student risk propensity increases, students will tend to view online learning technology as more controllable and manageable, leading to outcome expectations and high utility associated with the system. The magnitude of risk is negatively related to perceived benefits (Horst, Kuttschreuter, & Gutteling, 2007). Horst et al., for example, report that risk perception significantly predicts perceived usefulness of electronic services. A greater perception of risk will reduce the perceived usefulness of new technology (Gefen, Warkentin, Pavlou, & Rose, 2002). Goal setting and planning is also more likely when students with higher risk propensity link new technology with lower levels of uncertainty. This will enhance perceived usefulness of new technology, since the higher the perceived manageability, the higher the perceived usefulness is for improved performance.
Proposition 4. Risk propensity will be positively related to perceived ease of use and usefulness of online learning environments, and these relationships will be mediated by the self-regulation phase of task analysis and self-motivation beliefs.
Technical support availability. Computing or technical support is considered to be an important predictor of technology use (e.g., Lee, 2008). Such support may be provided by either internal or external sources. Internal sources are those internal to the organization such as the information technology (IT) department of a college or company, and IT personnel or experts employed by the institution. External sources could be peers, friends, software or hardware vendors, or any institution outside of the organization in which the technology is being used. For online learning, computing support may be available through IT centers and specialists, through manuals and handbooks, or through instructors. Research has found that lack of technical support leads to failure of technology adoption (Guimaraes, Gupta, & Rainer, 1999). Availability of technical support promotes a learner's belief that new technology is more likely to enhance his/her performance as help is available when needed or when an obstacle is faced, and so in the learner's eyes, technical problems are more likely to find solutions fast, and rather than be a hurdle to learning, technology can in fact facilitate learning. Hence, technical support availability contributes to positive outcome expectations, and more specific goal setting and planning. The more available technical support and related resources are, the more likely it is for a learner to perceive the new online learning system as easy to use and believe that the technology is useful for their learning and online course performance.
Proposition 5. Technical support availability will be positively related to perceived ease of use and usefulness of online learning environments, and these relationships will be mediated by the self-regulation phase of task analysis and self-motivation beliefs.
Technology training. Technology training with regard to online learning refers to transfer of knowledge about the specific course management software or system that will be used as the platform for online learning. Training may be available through departments or resources internal to the institution, for example an IT department of a university or college. As well, training may exist outside the institution from software vendors or educational institutions outside the current organizational context. Training, whether internal or external, is positively related to technology acceptance (e.g., Agarwal & Prasad, 1998). The main reason for this finding is that training serves as a precursor to positive attitudes about the technology, and it eliminates negative affect such as apprehension about something new and unpredictable, arming the learner with more perceived control. Learner perceptions that training is available facilitates acceptance of the system and leads to more positive attitudes about the technology involved in learning. In parallel, several studies have found that perceived technology training is positively related to perceived ease of use and perceived usefulness of the technology (Amoroso & Cheney, 1991; Igbaria, Zinatelli, Cragg, & Cavaye, 1997; Lee, 2008). Technology training also helps the learners' planning and goal setting process in that it provides relatively more concrete information about what to expect, and thus aligns learned expectations with the actual work that will be demanded by the process of learning through the use of this system.
Proposition 6. Availability of training will be positively related to perceived ease of use and usefulness of online learning environments, and these relationships will be mediated by the self-regulation phase of task analysis and self-motivation beliefs.
Equipment accessibility. Often times, learners perceive a system or technology as challenging and difficult to use because the hardware and software equipment that is necessary to complete the tasks is not readily accessible. There is evidence that easy access to necessary technology and equipment is an antecedent of learner acceptance of course management systems (e.g., Dos Santos & Wright, 2001; Park, 2009). Even though Martins and Kellermanns (2004) report a non-significant relationship between system accessibility and perceived ease of system use, their measure involves an overall general access to the system rather than separating internal and external equipment access. Lee (2008) separates internal and external equipment accessibility, defining internal equipment as hardware and software provided by the organization and external equipment as system hardware and software outside an organization. Lee's findings have provided no support for a relationship between equipment accessibility and perceived usefulness, and no support for a relationship between perceived ease of use and internal equipment accessibility. However, results have indicated that access to an online learning system outside the university is positively associated with ease of use, meaning that learners have more chances to practice, which contributes to their finding the system easy to use (Lee, 2008).
Similar to Lee's (2008) findings, Park (2009) also reports a non-significant relationship between system accessibility and perceived usefulness. Hence, the authors chose not to propose a relationship for usefulness. As for perceived ease of use, external equipment accessibility, meaning access to the software and hardware outside the campus or classroom seems likely to facilitate learner goal setting and planning as well as enhance outcome expectations (Lee, 2008; Park, 2009) because of the effect of the sense of convenience that people have from being able to access the system without having to go to campus. Sense of convenience and easy access contribute to positive affect about the online learning system, and so users are more likely to accept it and learn it. Accessibility of equipment outside the campus is also likely to help support beliefs about one's ability to complete tasks offered online successfully. As a result, users with an increased sense of equipment access and convenience off campus will tend to more easily engage in task analysis, goal setting, planning, and other self-motivating mechanisms. These mechanisms will contribute to the perception of ease of use of the online system through strengthening a sense of control over the online learning system.
Proposition 7. External equipment accessibility will be positively related to perceived ease of use of online learning environments, and this relationship will be mediated by the self-regulation phase of task analysis and self-motivation beliefs.
Perceived Ease of Use and Perceived Usefulness
According to the TAM, perceived ease of use predicts perceived usefulness of an information system (Davis, 1989). Specifically, when learners perceive that their use of a system is relatively easy or rather free of extra effort, they are also more likely to believe that using the system will improve their performance. Lee (2008) found evidence in support of this prediction. With over 1,100 student participants in a research study, Lee showed that students' perceived ease of use of an online learning system is significantly and positively related to their perceptions of usefulness of the online learning system. Martins and Kellermanns (2004) also found that perceived ease of use of a web-based course management system is positively and significantly related to the perceived usefulness of the system. More recent research (Lee et al., 2005; Teo, 2009, 2010) further links perceived ease of use with perceived usefulness. For example, Teo (2009) found that teachers' self-reported perceived ease of use of computers explains their perceived usefulness of computers.
Proposition 8. Perceived ease of use of the online learning system will predict perceived usefulness of the online learning system.
Acceptance of Online Learning Systems
TAM has focused on user acceptance of a new information system as its main criterion variable (Arbaugh, 2000). Prior research on the model has defined user acceptance as an interrelated combination of user attitudes toward the new information system, behavioral intention to use the system, and the actual use of the system (Martins & Kellermanns, 2004; Teo, 2009). The most common operationalization of attitude toward the system is user satisfaction with the system (e.g., Compeau et al., 1999; Martins & Kellermanns, 2004). Martins and Kellermanns also included student preference for the system as an additional dimension to capture attitude toward course management systems and found support for a positive relationship between attitudes toward the course management system and the students' intentions to use the system. Preference for online learning is an important dimension of online learning as it indicates that the user actually prefers the new system over more traditional methods of course delivery like completely face-to-face or web-supported instruction.
This model takes these well-tested relationships one step further and looks at the actual performance implications of attitude toward a system. In online learning, basic technology skills as well as skills with learning through an online system and so having a self-regulated focus become key factors for performance. Satisfaction with the system in use for online course management may be a critical mediating variable to explain the processes through which users' perceptions of ease of use and usefulness of the system lead to performance. Since each online learning system has different characteristics, one can expect that learners' level of satisfaction and preference for using the system over more traditional methods will also vary accordingly. Organizational behavior literature provides meta-analytic support for the satisfaction-performance relationship (e.g., Whitman, Van Rooy, & Viswesvaran, 2010). In fact, "attitudes serve as a host of important psychological functions including assisting knowledge and schema formation, providing evaluative strategies for problem solving, helping to organize and order memory" (Whitman et al., 2010, p. 44). Thus, attitude toward an online learning system is likely to affect the level of performance reached by using the system.
Proposition 9. Perceived ease of use and perceived usefulness of online courses will be positively related to performance in online learning systems, and this relationship will be mediated by attitudes toward the online learning system.
Performance in Online Courses and Self-Regulation
Actual performance in a course as a whole or in any course component serves as feedback for individual learners to evaluate their own performance and make judgments and causal attributions. An example could be lower than expected performance. When the learner evaluates this lower than expected level of performance he or she can attribute it to different causes such as little time allocated to studying, lack of an alignment between the instructor's style and the student's learning style, lack of resource availability, unfair grading, or course difficulty. This is the stage of self-reflection in the self-regulation phases (Zimmerman, 2008). Performance information is also likely to lead to specific emotions depending on how the information is evaluated. For example, if the learner evaluates the grade or course outcome to be largely unfair, negative emotions are likely to follow. If the learner attributes the performance level to little time allocated to studying, he or she may feel disappointment at his or her own lack of good planning or time management. There is evidence that learner performance phase processes such as task instruction and task strategies are causally associated with self-reflection phase sub-processes such as attribution, self-satisfaction, and affect (Zimmerman & Kitsantas, 1997).
Proposition 10. Overall online course performance will predict self-reflection phase processes of causal attribution, self-evaluation, and feelings of satisfaction with performance in the course.
Self-Reflection and Forethought Phases
According to the self-regulation theory, as part of the iterative phase cycle, the subsequent phase after self-reflection is the forethought phase where individuals, now armed with new information that they evaluated and judged, are ready for incorporating this new information into their strategic plans and goal setting (Zimmerman, 2008). The new information, which primarily is their interpretation and evaluation of their performance data, also serves the function of prior experience and so has direct influence on task-specific self-efficacy, task interest and task value, as well as their outcome expectations. For example, prior experience with and past performance in online courses may shape the self-efficacy beliefs of students and also have an influence on how much interest they will have in learning the course material through this platform. Prior experience may also determine what students expect from online courses in the future. Zimmerman and Kitsantas (1999) provide evidence for this line of thought in that they have found that the self-reflection phase feeling of satisfaction with one’s own performance predicted students' motivation to improve performance, specifically self-efficacy and task interest.
Proposition 11. Processes of the self-reflection phase such as self-evaluation, causal attribution, and self-satisfaction with performance will predict the task analysis and self-motivation belief processes of the forethought phase.
Discussion
In this paper, it is suggested that the iterative process of self-regulated learning is crucial in calibrating students in an online learning environment. The framework that was developed represents an integration of three different theories (i.e., the five-factor model of personality, cyclical phases and sub-processes of self-regulation, and TAM). The paper contributes to previous research on online learning, self-regulation, and technology acceptance in three ways.
First, previous literature in self-regulated learning processes shows a positive relationship between self-regulated learning processes and calibration of knowledge although the causality in this relationship is still uncertain (Zimmerman, 2008). Recently, Sitzmann and Ely (2010) reported a causal relationship between self-regulatory processes and both learning and attrition in an online class. Furthermore, self-regulatory processes were found to mediate the relationship between prompting self-regulation and learning outcomes such as knowledge acquisition and attrition. However, few studies have integrated the role of personality in the self-regulatory process. To the knowledge of the authors, the few prior research studies (e.g., Caspi, Chajut, Saporta, & Beyth-Marom, 2006) only examined the relationship between personality traits of students and their participation in an online learning environment.
Second, previous research only focused on whether teachers could modify students' level of self-regulatory processes across three phases (i.e., forethought, performance, and self-reflection) using a variety of measures such as prompting: asking questions prior to and during the online training course (e.g., Sitzmann & Ely, 2010). In contrast, it is suggested here that students are active participants of the self-regulatory process by their own initiative of making changes. It is suggested that students evaluate the usefulness of technology as well as its ease of use prior to and during online courses. This, in turn, is proposed to have an impact on key performance indicators of online learning such as knowledge acquisition and attrition.
Third, previous research seems to be most concerned with the consequences of online learning and online communities such as knowledge acquisition and/or attrition or student engagement in online courses (Young & Bruce, 2011). In contrast, it is suggested here that antecedents of online learning are important to consider as well. In the proposed model, situational variables such as technical support availability, technology training, and external equipment accessibility are introduced.
It must be noted that an alternative conceptualization of the model exists. For example, even though personality has been found to have a direct impact on overall online course performance (e.g., Caspi et al., 2006), this direct linkage was not included in our model. It was suggested that a self-regulated learning process will fully mediate the relationship between personality and overall course performance. Given the moderate positive effect of conscientiousness on learning performance (r = .3 as reported in most meta-analyses) any leftover effect would be non-significant after taking into consideration self-regulated learning's calibration effect on performance. It was also noted that some scholars conceptualize the self-regulatory process as three distinct and cyclical phases consisting of three constructs (i.e., motivation representing forethought, meta-cognition, representing performance, and concentration, representing self-reflection) (e.g., Bandura, 1986, Vancouver & Day, 2005). In this model, self-regulation is viewed as one higher order construct consisting of forethought, performance, and self-reflection (Zimmerman, 2008), which facilitates the empirical testing of the model.
Limitations and Future Research
One of the limitations of this paper is that currently there is no data or empirical evidence to support the model as a whole. Even though there is empirical and conceptual support for the individual relationships discussed and the propositions developed, an empirical test of the whole model is certainly warranted so that the model can be appropriately validated.
Another limitation is the conceptualization of the proposed relationships from an individual learner's perspective only. Many online or blended courses involve mandatory group projects. Group dynamics bring several challenges to an online learning context. For example, how does personality factor into group dynamics and group learning outcomes? This is an area of future empirical research. At the same time, theoretically, incorporating a teamwork concept to this model will likely have changed the proposed personality variables. For example, the agreeableness dimension of the Big Five model becomes relevant when group interaction is taken into account.
This model has several implications for research on online learning. First, future research should examine how self-regulated learning can be best measured in an online class. Because the previous research relies predominantly on student self-report of self-regulatory process (e.g., Sitzmann & Ely, 2010), the accuracy of self-regulated learning measures is suspect. Zimmerman (2008) reviewed several online measures (e.g., trace logs, online diary) as comparable measures of self-regulated learning for online learning environments. However, more research is needed.
Second, future studies should investigate whether and how instructors can modify course design to increase self-regulated learning among students enrolled in online classes. This will provide the needed empirical evidence to justify the rush to online course offerings currently happening in U.S. colleges.
Third, future scholars should attempt to build upon the integrated model the authors have proposed in this paper. Currently, the model includes variables at the individual level. It is possible to integrate a multi-level or mixed-level perspective into this conceptualization. For example, it is possible to generalize self-regulated learning to the calibration of self-regulated learning for groups or populations of students.
Further, course design can be studied as a moderating variable of the self-regulatory process and overall performance in an online course. Whereas previous research examines instructors' interventions such as using prompts (e.g., Sitzmann & Ely, 2010), learner control, and feedback received during the course (e.g., Sitzmann, Kraiger, Stewart, & Wisher, 2006) as course design variables, there is a need to expand the list to include course content (e.g., leadership vs. statistics). There are also potential moderators of the attitude-performance relationship such as time spent on task, focus in studying, and an accommodating physical environment conducive to effective learning and course performance.
Future empirical studies should involve longitudinal designs and longitudinal data collection. There are many studies that rely on cross-sectional measurement methods. Such methods, while producing findings in a more efficient way, suffer from not being able to assert temporal causality. In addition, collecting data at one point in time through a single source, such as by use of self-report measures, makes findings suspect and subject to common methods bias. Collecting data through multiple sources at different points in time or running post-hoc analyses for common methods bias (Martins & Kellermanns, 2004) may alleviate this problem.
In practice, an emergent question is whether the instructors can (or whether they should) modify their courses so that improvements in self-regulated learning are encouraged and fostered. As the tendency to offer more online courses increases and as more learning opportunities become available to students, the need to understand and explain how learner traits and resources interact with the learning environment grows as well. A better understanding of such interactions will help instructors in effective course design and in channeling support where needed and appropriate, such as to lower level self-regulating learners.
References
Agarwal, R., & Prasad, J. (1998). A conceptual and operational definition of innovativeness in the domain of information technology. Information Systems Research, 9(2), 204-215. doi:10.1287/isre.9.2.204
Amoroso, D. L., & Cheney, P. H. (1991). Testing a causal model of end-user application effectiveness. Journal of Management Information Systems, 8(1), 63-90.
Arbaugh, J. B. (2000). How classroom environment and student engagement affect learning in Internet-based MBA courses. Business Communication Quarterly, 63(4), 9-26. doi:10.1177/108056990006300402
Bandura, A. (1986). Social foundations of thought and action: A social cognitive theory. Englewood Cliffs, NJ: Prentice-Hall.
Barrick, M. R., & Mount, M. K. (2005). Yes, personality matters: Moving on to more important matters. Human Performance, 18(4), 359-372. doi:10.1207/s15327043hup1804_3
Blickle, G. (1996). Personality traits, learning strategies, and performance. European Journal of Personality, 10(5), 337-352. doi:10.1002/(SICI)1099-0984(199612)10:5<337::AID-PER258>3.0.CO;2-7
Boekaerts, M., Pintrich, P. R., & Zeidner, M. (Eds.). (2000). Handbook of self-regulation. Orlando, FL: Academic Press.
Boekaerts, M., & Corno, L. (2005). Self-regulation in the classroom: A perspective on assessment and intervention. Applied Psychology, 54(2), 199-231. doi:10.1111/j.1464-0597.2005.00205.x
Brockhaus, R. H. (1980). Risk taking propensity of entrepreneurs. Academy of Management Journal, 23(3), 509-520. doi:10.2307/255515
Caspi, A., Chajut, E., Saporta, K., & Beyth-Marom, R. (2006). The influence of personality on social participation in learning environments. Learning and Individual Differences, 16(2), 129-144. doi:10.1016/j.lindif.2005.07.003
Chamorro-Premuzic, T., & Furnham, A. (2003). Personality predicts academic performance: Evidence from two longitudinal university samples. Journal of Research in Personality, 37(4), 319-338. doi:10.1016/S0092-6566(02)00578-0
Chen, G., Gully, S. M., & Eden, D. (2001). Validation of a new general self-efficacy scale. Organizational Research Methods, 4(1), 62-83. doi:10.1177/109442810141004
Chen, G., Gully, S. M., Whiteman, J.-A., & Kilcullen, B. N. (2000). Examination of relationships among trait-like individual differences, state-like individual differences, and learning performance. Journal of Applied Psychology, 85(6), 835-847. doi:10.1037/0021-9010.85.6.835
Cho, M.-H., & Jonassen, D. H. (2009). Development of the human interaction dimension of the Self-Regulated Learning Questionnaire in asynchronous online learning environments. Educational Psychology, 29(1), 117-138. doi:10.1080/01443410802516934
Compeau, D., Higgins, C. A., & Huff, S. (1999). Social cognitive theory and individual reactions to computing technology: A longitudinal study. MIS Quarterly, 23(2), 145-158. doi:10.2307/249749
Das, T. K., & Teng, B. (2004). The risk-based view of trust: A conceptual framework. Journal of Business and Psychology, 19(1), 85-116. doi:10.1023/B:JOBU.0000040274.23551.1b
Davis, F. D. (1989). Perceived usefulness, perceived ease of use, and user acceptance of information technology. MIS Quarterly, 13(3), 319-340. doi:10.2307/249008
Digman, J. M. (1990). Personality structure: Emergence of the five-factor model. Annual Review of Psychology, 41, 417-440. doi:10.1146/annurev.ps.41.020190.002221
Dillon, S. (2008, July 11). High cost of driving to college ignites online classes boom. The New York Times. Retrieved from http://www.nytimes.com/2008/07/11/education/11colleges.html?
pagewanted=all&_r=0
Dos Santos, B. L., & Wright, A. L. (2001). Internet-supported management education. Information Services & Use, 21(2), 53-64.
Eden, D. (2001). Means efficacy: External sources of general and specific subjective efficacy. In M. Erez, U. Kleinbeck, & H. Thierry (Eds.), Work motivation in the context of a globalizing economy (pp. 73-86). Hillsdale, NJ: Erlbaum.
Feist, G. J. (1998). A meta-analysis of personality in scientific and artistic creativity. Personality and Social Psychology Review, 2(4), 290-309. doi:10.1207/s15327957pspr0204_5
Fishbein, M., & Ajzen, I. (1975). Belief, attitude, intention, and behavior: An introduction to theory and research. Reading, MA: Addison-Wesley.
Gefen, D., Warkentin, M., Pavlou, P. A., & Rose, G. M. (2002). eGovernment adoption. In R. D. Banker, H. Chang, & Y.-C. Kao (Eds.), Proceedings of the Eighth Americas Conference on Information Systems (pp. 569-576). Atlanta, GA: Association for Information Systems. Available from AIS Electronic Library. (AMCIS 2002, Paper 83)
Gerhardt, M. W., Rode, J. C., & Peterson, S. J. (2007). Exploring mechanisms in the personality–performance relationship: Mediating roles of self-management and situational constraints. Personality and Individual Differences, 43(6), 1344-1355. doi:10.1016/j.paid.2007.04.001
Golden, D. (2006, May 9). Degrees@StateU.edu: Online university enrollment soars as quality improves; tuition funds other projects. The Wall Street Journal. Retrieved from http://online.wsj.com/article/SB114713782174047386.html
Grandon, E. E., Alshare, K., & Kwun, O. (2005). Factors influencing student intention to adopt online classes: A cross-cultural study. Journal of Computing Sciences in Colleges, 20(4), 46-56. Available from ACM Digital Library. (1047853)
Guimaraes, T., Gupta, Y. P., & Rainer, R. K., Jr. (1999). Empirically testing the relationship between end-user computing problems and information center success factors. Decision Sciences, 30(2), 393-413. doi:10.1111/j.1540-5915.1999.tb01615.x
Holland, D. C., Dollinger, S. J., Holland, C. J., & MacDonald, D. A. (1995). The relationship between psychometric intelligence and the five factor model of personality in a rehabilitation sample. Journal of Clinical Psychology, 51(1), 79-88. doi:10.1002/1097-4679(199501)51:1<79::AID-JCLP2270510113>3.0.CO;2-P
Horst, M., Kuttschreuter, M., & Gutteling, J. M. (2007). Perceived usefulness, personal experiences, risk perception and trust as determinants of adoption of e-government services in The Netherlands. Computers in Human Behavior, 23(4), 1838-1852. doi:10.1016/j.chb.2005.11.003
Igbaria, M., Zinatelli, N., Cragg, P., & Cavaye, A. L. M. (1997). Personal computing acceptance factors in small firms: A structural equation model. MIS Quarterly, 21(3), 279-305. doi:10.2307/249498
Judge, T. A., Bono, J. E., & Locke, E. A. (2000). Personality and job satisfaction: The mediating role of job characteristics. Journal of Applied Psychology, 85(2), 237-249. doi:10.1037/0021-9010.85.2.237
Judge, T. A., Bono, J. E., Ilies, R., & Gerhardt, M. W. (2002). Personality and leadership: A qualitative and quantitative review. Journal of Applied Psychology, 87(4), 765-780. doi:10.1037/0021-9010.87.4.765
Judge, T. A., & Erez, A. (2007). Interaction and intersection: The constellation of emotional stability and extraversion in predicting performance. Personnel Psychology, 60(3), 573-596. doi:10.1111/j.1744-6570.2007.00084.x
Judge, T. A., Erez, A., & Bono, J. E. (1998). The power of being positive: The relation between positive self-concept and job performance. Human Performance, 11(2-3), 167-187. doi:10.1080/08959285.1998.9668030
Judge, T. A., & Ilies, R. (2002). Relationship of personality to performance motivation: A meta-analytic review. Journal of Applied Psychology, 87(4), 797-807. doi:10.1037/0021-9010.87.4.797
Judge, T. A., Jackson, C. L., Shaw, J. C., Scott, B. A., & Rich, B. L. (2007). Self-efficacy and work-related performance: The integral role of individual differences. Journal of Applied Psychology, 92(1), 107-127. doi:10.1037/0021-9010.92.1.107
Just, C. (2011). A review of literature on the general factor of personality. Personality and Individual Differences, 50(6), 765-771. doi:10.1016/j.paid.2011.01.008
Kamdar, D., & Van Dyne, L. (2007). The joint effects of personality and workplace social exchange relationships in predicting task performance and citizenship performance. Journal of Applied Psychology, 92(5), 1286-1298. doi:10.1037/0021-9010.92.5.1286
Karoly, P. (1993). Mechanisms of self-regulation: A systems view. Annual Review of Psychology, 44, 23-52. doi:10.1146/annurev.ps.44.020193.000323
Klein, H. J., & Lee, S. (2006). The effects of personality on learning: The mediating role of goal setting. Human Performance, 19(1), 43-66. doi:10.1207/s15327043hup1901_3
Lee, Y.-C. (2008). The role of perceived resources in online learning adoption. Computers & Education, 50(4), 1423-1438. doi:10.1016/j.compedu.2007.01.001
Lee, M. K. O., Cheung, C. M. K., & Chen, Z. (2005). Acceptance of Internet-based learning medium: The role of extrinsic and intrinsic motivation. Information & Management, 42(8), 1095-1104. doi:10.1016/j.im.2003.10.007
Lee, S., & Klein, H. J. (2002). Relationships between conscientiousness, self-efficacy, self-deception, and learning over time. Journal of Applied Psychology, 87(6), 1175-1182. doi:10.1037/0021-9010.87.6.1175
Lee, J.-K., & Lee, W.-K. (2008). The relationship of e-learner's self-regulatory efficacy and perception of e-learning environmental quality. Computers in Human Behavior, 24(1), 32-47. doi:10.1016/j.chb.2006.12.001
Lin, J. C., & Lu, H. (2000). Towards an understanding of the behavioural intention to use a web site. International Journal of Information Management, 20(3), 197-208. doi:10.1016/S0268-4012(00)00005-0
Lord, R. G., Diefendorff, J. M., Schmidt, A. M., & Hall, R. J. (2010). Self-regulation at work. Annual Review of Psychology, 61, 543-568. doi:10.1146/annurev.psych.093008.100314
Martins, L. L., & Kellermanns, F. W. (2004). A model of business school students' acceptance of a web-based course management system. Academy of Management Learning and Education, 3(1), 7-26. doi:dx.doi.org/10.5465/AMLE.2004.12436815
McCrae, R. R. (1987). Creativity, divergent thinking, and openness to experience. Journal of Personality and Social Psychology, 52(6), 1258-1265. doi:10.1037/0022-3514.52.6.1258
McCrae, R. R. (1993-1994). Openness to experience as a basic dimension of personality. Imagination, Cognition and Personality, 13(1), 39-55. doi:10.2190/H8H6-QYKR-KEU8-GAQ0
McCrae, R. R. (1996). Social consequences of experiential openness. Psychological Bulletin, 120(3), 323-337. doi:10.1037/0033-2909.120.3.323
Muis, K. R. (2007). The role of epistemic beliefs in self-regulated learning. Educational Psychologist, 42(3), 173-190. doi:10.1080/00461520701416306
Nenniger, P. (2005). Commentary on self-regulation in the classroom: A perspective on assessment and intervention. Applied Psychology, 54(2), 239-244. doi:10.1111/j.1464-0597.2005.00207.x
Park, S. Y. (2009). An analysis of the technology acceptance model in understanding university students' behavioral intention to use e-learning. Educational Technology & Society, 12(3), 150-162. Retrieved from http://www.ifets.info/journals/12_3/14.pdf
Pintrich, P. R. (2000). The role of goal orientation in self-regulated learning. In M. Boekaerts, P. R. Pintrich, & M. Zeidner (Eds.), Handbook of self-regulation (pp. 451-502). San Diego, CA: Academic Press. doi:10.1016/B978-012109890-2/50043-3
Pintrich, P. R., & Schrauben, B. (1992). Students' motivational beliefs and their cognitive engagement in classroom academic tasks. In D. H. Schunk & J. L. Meece (Eds.), Student perceptions in the classroom (pp. 149-183). Hillsdale, NJ: Erlbaum.
Pintrich, P. R., Smith, D. A. F., Garcia, T., & McKeachie, W. J. (1993). Reliability and predictive validity of the Motivated Strategies for Learning Questionnaire (MSLQ). Educational and Psychological Measurement, 53, 801-813. doi:10.1177/0013164493053003024
Ross, D. N., & Rosenbloom, A. (2011). Reflections on building and teaching an undergraduate strategic management course in a blended format. Journal of Management Education, 35(3), 351-376. doi:10.1177/1052562911398979
Rossett, A. (2006, September). How blended learning changes what we do. Learning Circuits. Retrieved June 2, 2008 from http://www.learningcircuits.org/2006/September/rossett.htm (archived at http://web.archive.org/web/20080602115803/http://www.learningcircuits.org/2006/September/
rossett.htm)
Schunk, D. H. (1996). Learning theories: An educational perspective (2nd ed.). Englewood Cliffs, NJ: Prentice-Hall.
Selim, H. M. (2003). An empirical investigation of student acceptance of course web sites. Computers & Education, 40(4), 343-360. doi:10.1016/S0360-1315(02)00142-2
Sitkin, S. B., & Pablo, A. L. (1992). Reconceptualizing the determinants of risk behavior. Academy of Management Review, 17(1), 9-38. doi:10.5465/AMR.1992.4279564
Sitkin, S. B., & Weingart, L. R. (1995). Determinants of risky decision-making behavior: A test of the mediating role of risk perceptions and propensity. Academy of Management Journal, 38(6), 1573-1592. doi:10.2307/256844
Sitzmann, T. M., & Ely, K. (2010). Sometimes you need a reminder: The effects of prompting self-regulation on regulatory processes, learning, and attrition. Journal of Applied Psychology, 95(1), 132-144. doi:10.1037/a0018080
Sitzmann, T. M., Kraiger, K., Stewart, D., & Wisher, R. (2006). The comparative effectiveness of web-based and classroom instruction: A meta-analysis. Personnel Psychology, 59(3), 623-664. doi:10.1111/j.1744-6570.2006.00049.x
Small, E. E., & Diefendorff, J. M. (2006). The impact of contextual self-ratings and observer ratings of personality on the personality–performance relationship. Journal of Applied Social Psychology, 36(2), 297-320. doi:10.1111/j.0021-9029.2006.00009.x
Tabak, F., Nguyen, N. T., Basuray, T., & Darrow, W. (2009). Exploring the impact of personality on performance: How time-on-task moderates the mediation by self-efficacy. Personality and Individual Differences, 47(8), 823-828. doi:10.1016/j.paid.2009.06.027
Teo, T. (2009). The impact of subjective norm and facilitating conditions on pre-service teachers' attitude toward computer use: A structural equation modeling of an extended technology acceptance model. Journal of Educational Computing Research, 40(1), 89-109. doi:10.2190/EC.40.1.d
Teo, T. (2010). Explaining the intention to use technology among volitional users in education: An evaluation of the technology acceptance model (TAM) using structural equation modeling. International Journal of Instructional Media, 37(4), 379–389.
Tsai, I.-C., Tung, I.-P., & Laffey, J. (2008, March). Exploring how students' self-regulated learning influences the social nature of online learning. Paper presented at the 2008 Annual Meeting of the American Educational Research Association, New York, NY. Retrieved from http://gozips.uakron.edu/~tsai1/files/AERA08_submission_0731.pdf
Tsai, I.-C., Tung, I.-P., & Laffey, J. (2013). Exploring the impact of students' motivation and self-regulation on the social nature of online learning experiences. International Journal of Learning Technology, 8(1), 86-108. doi:10.1504/IJLT.2013.052833
Vancouver, J. B., & Day, D. V. (2005). Industrial and organisation research on self-regulation: From constructs to applications. Applied Psychology, 54(2), 155-185. doi:10.1111/j.1464-0597.2005.00202.x
Venkatesh, V., & Davis, F. D. (2000a). A model of the antecedents of perceived ease of use: Development and test. Decision Sciences, 27(3), 451-481. doi:10.1111/j.1540-5915.1996.tb00860.x
Venkatesh, V., & Davis, F. D. (2000b). A theoretical extension of the technology acceptance model: Four longitudinal field studies. Management Science, 46(2), 186-204. doi:10.1287/mnsc.46.2.186.11926
Whitman, D. S., Van Rooy, D. L., & Viswesvaran, C. (2010). Satisfaction, citizenship behaviors, and performance in work units: A meta-analysis of collective construct relations. Personnel Psychology, 63, 41-81. doi:10.1111/j.1744-6570.2009.01162.x
Williams, J. E., & Hellman, C. M. (1998). Investigating self-regulated learning among first-generation community college students. Journal of Applied Research in the Community College, 5(2), 83-87.
Williams, P. E., & Hellman, C. M. (2004). Differences in self-regulation for online learning between first- and second-generation college students. Research in Higher Education, 45(1), 71-82. doi:10.1023/B:RIHE.0000010047.46814.78
Winne, P. H., & Hadwin, A. F. (1998). Studying as self-regulated learning. In D. J. Hacker, J. Dunlosky, & A. C. Graesser (Eds.), Metacognition in educational theory and practice (pp. 277-304). Mahwah, NJ: Erlbaum.
Wood, R., & Bandura, A. (1989). Impact of conceptions of ability on self-regulatory mechanisms and complex decision making. Journal of Personality and Social Psychology, 56, 407-415. doi:10.1037/0022-3514.56.3.407
Young, S., & Bruce, M. A. (2011). Classroom community and student engagement in online courses. MERLOT Journal of Online Learning and Teaching, 7(2), 219-230. https://jolt.merlot.org/vol7no2/young_0611.htm
Zhang, P., & Goel, L. (2011). Is e-learning for everyone? An internal–external framework of e-learning initiatives. MERLOT Journal of Online Learning and Teaching, 7(2), 193-205. Retrieved from https://jolt.merlot.org/vol7no2/goel_0611.htm
Zhang, S., Zhao, J., & Tan, W. (2008). Extending TAM for online learning systems: An intrinsic motivation perspective. Tsinghua Science & Technology, 13(3), 312-317. doi:10.1016/S1007-0214(08)70050-6
Zimmerman, B. J. (1998). Developing self-fulfilling cycles of academic regulation: An analysis of exemplary instructional models. In D. H. Schunk & B. J. Zimmerman (Eds.), Self-regulated learning: From teaching to self-reflective practice (pp. 1-19). New York, NY: Guilford Press.
Zimmerman, B. J. (2000). Attaining self-regulation: A social cognitive perspective. In M. Boekaerts, P. R. Pintrich, & M. Zeidner (Eds.), Handbook of self-regulation: Research and applications (pp. 13-39). Orlando, FL: Academic Press. doi:10.1016/B978-012109890-2/50031-7
Zimmerman, B. J. (2008). Investigating self-regulation and motivation: Historical background, methodological developments, and future prospects. American Educational Research Journal, 45(1), 166-183. doi:10.3102/0002831207312909
Zimmerman, B. J., & Bandura, A. (1994). Impact of self-regulatory influences on writing course attainment. American Educational Research Journal, 31(4), 845-862. doi:10.3102/00028312031004845
Zimmerman, B. J., & Campillo, M. (2003). Motivating self-regulated problem solvers. In J. E. Davidson & R. J. Sternberg (Eds.), The psychology of problem solving (p. 233-262). Cambridge, UK: Cambridge University Press. doi:10.1017/CBO9780511615771.009
Zimmerman, B. J., & Kitsantas, A. (1997). Developmental phases in self-regulation: Shifting from process goals to outcome goals. Journal of Educational Psychology, 89(1), 29-36. doi:10.1037/0022-0663.89.1.29
Zimmerman, B. J., & Kitsantas, A. (1999). Acquiring writing revision skill: Shifting from process to outcome self-regulatory goals. Journal of Educational Psychology, 91(2), 241-250. doi:10.1037/0022-0663.91.2.241